Contrastive Disentanglement for Quantitative Ultrasound Muscle Atrophy Evaluation.
IEEE transactions on bio-medical engineering(2024)
摘要
OBJECTIVE:Muscle atrophy reduces the quality of life and increases morbidity and mortality from other diseases. The development of non-invasive muscle atrophy evaluation method is of great practical value. The lack of gold standard for pathological grading usually allows only the duration of weightlessness as a criterion for the degree of atrophy. However, the adaptive reductive remodeling of muscle physiology and structure shows a trend of nonlinear changes in time. Consequently, using weightlessness time as a benchmark for the degree of atrophy is inaccurate.
METHODS:This paper proposes a new ultrasound imaging-based method for quantifying muscle atrophy that utilizes weakly supervised information between multiple data partitions with controlled variance components, overcoming the limitations of using the weightlessness time as a criterion. We introduce a group-supervised contrastive disentanglement network (GCDNet) to disentangle the individual variances, muscle growth and atrophy components of ultrasound images, and quantify the degree of atrophy using the disentangled atrophy component.
RESULTS:The feasibility of GCDNet is validated by the separability, independence, and representativeness of the disentangled components. To simplify the application of GCDNet, a muscle atrophy scoring network requiring no reference images is developed by distilling the GCDNet's knowledge of muscle atrophy quantization. The strength of the proposed methodology allows us, for the first time to our knowledge, to study the muscle growth attribute during hind-limb unloading and the spatial distribution of muscle atrophy.
更多查看译文
关键词
Muscle atrophy quantization,contrastive learning,disentangled representation learning,ultrasound
AI 理解论文
溯源树
样例
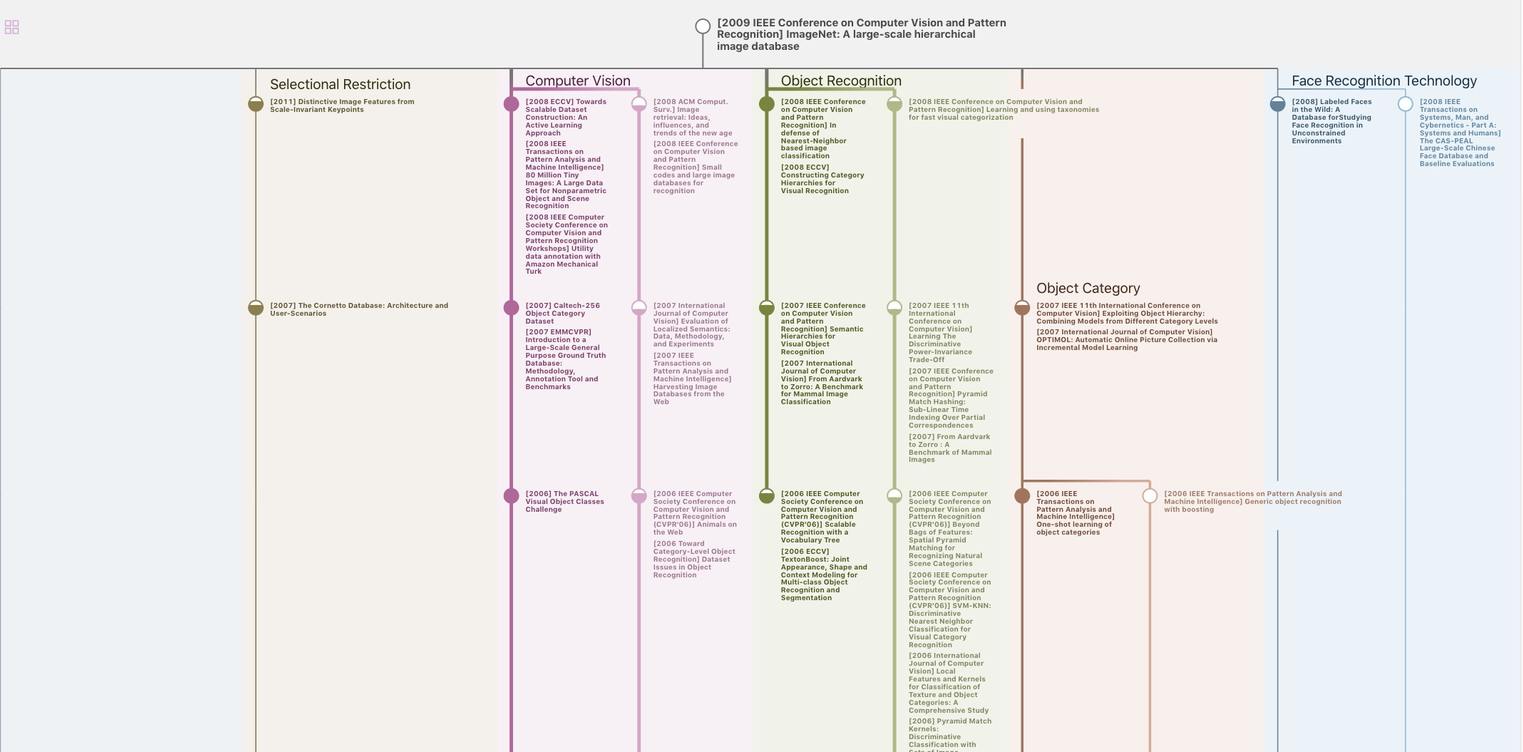
生成溯源树,研究论文发展脉络
Chat Paper
正在生成论文摘要