Unveiling camouflaged and partially occluded colorectal polyps: Introducing CPSNet for accurate colon polyp segmentation
COMPUTERS IN BIOLOGY AND MEDICINE(2024)
摘要
Background: Segmenting colorectal polyps presents a significant challenge due to the diverse variations in their size, shape, texture, and intricate backgrounds. Particularly demanding are the so-called "camouflaged"polyps, which are partially concealed by surrounding tissues or fluids, adding complexity to their detection. Methods: We present CPSNet, an innovative model designed for camouflaged polyp segmentation. CPSNet incorporates three key modules: the Deep Multi -Scale -Feature Fusion Module, the Camouflaged Object Detection Module, and the Multi -Scale Feature Enhancement Module. These modules work collaboratively to improve the segmentation process, enhancing both robustness and accuracy. Results: Our experiments confirm the effectiveness of CPSNet. When compared to state-of-the-art methods in colon polyp segmentation, CPSNet consistently outperforms the competition. Particularly noteworthy is its performance on the ETIS-LaribPolypDB dataset, where CPSNet achieved a remarkable 2.3% increase in the Dice coefficient compared to the Polyp -PVT model. Conclusion: In summary, CPSNet marks a significant advancement in the field of colorectal polyp segmentation. Its innovative approach, encompassing multi -scale feature fusion, camouflaged object detection, and feature enhancement, holds considerable promise for clinical applications.
更多查看译文
关键词
Camouflaged polyps,Segmentation,Feature fusion,Feature enhancement,Deep learning
AI 理解论文
溯源树
样例
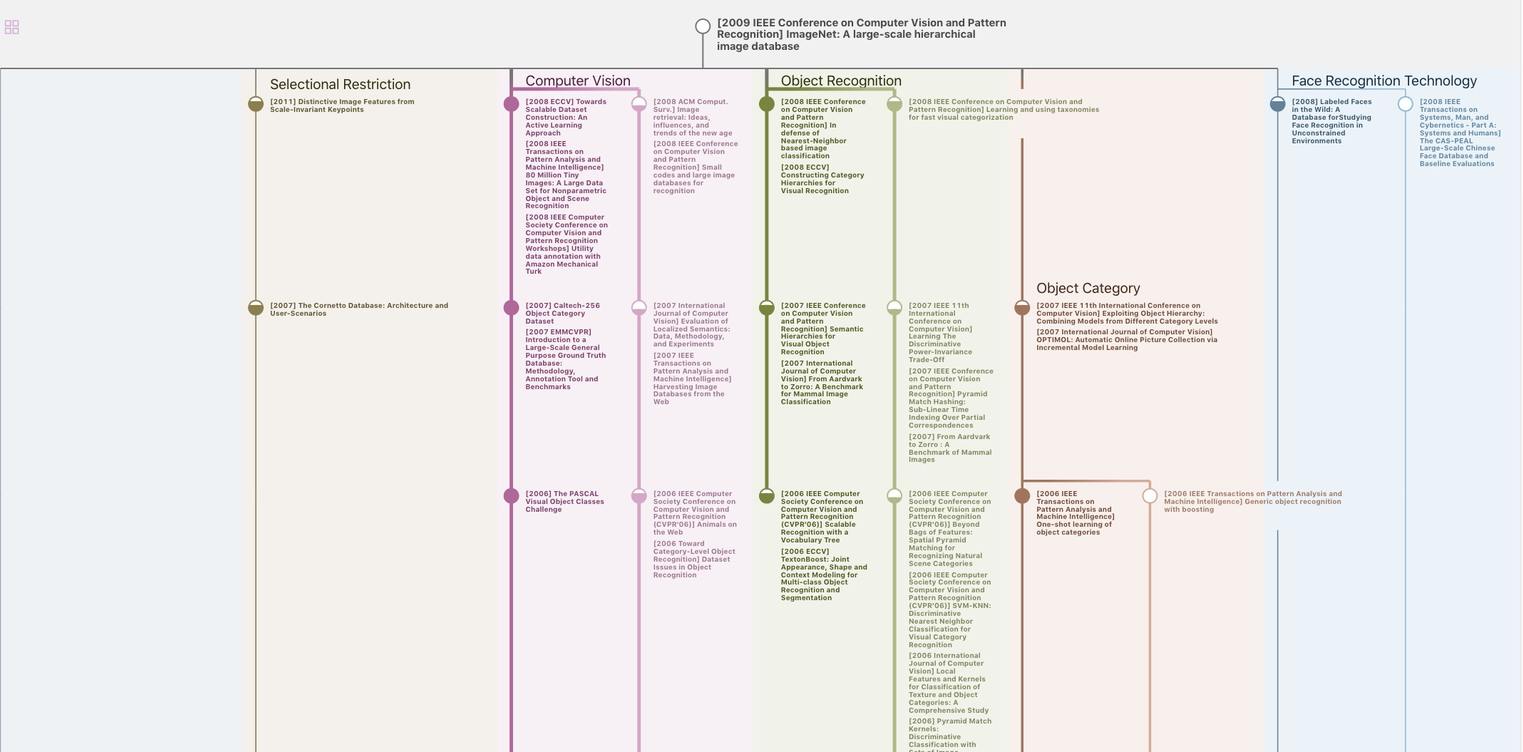
生成溯源树,研究论文发展脉络
Chat Paper
正在生成论文摘要