Satellite-based aerosol optical depth estimates over the continental U.S. during the 2020 wildfire season: Roles of smoke and land cover.
The Science of the total environment(2024)
摘要
Wildfires produce smoke that can affect an area >1000 times the burn extent, with far-reaching human health, ecologic, and economic impacts. Accurately estimating aerosol load within smoke plumes is therefore crucial for understanding and mitigating these impacts. We evaluated the effectiveness of the latest Collection 6.1 MODIS Multi-Angle Implementation of Atmospheric Correction (MAIAC) algorithm in estimating aerosol optical depth (AOD) across the U.S. during the historic 2020 wildfire season. We compared satellite-based MAIAC AOD to ground-based AERONET AOD measurements during no-, light-, medium-, and heavy-smoke conditions identified using the Hazard Mapping System Fire and Smoke Product. This smoke product consists of maximum extent smoke polygons digitized by analysts using visible band imagery and classified according to smoke density. We also examined the strength of the correlations between satellite- and ground-based AOD for major land cover types under various smoke density levels. MAIAC performed well in estimating AOD during smoke-affected conditions. Correlations between MAIAC and AERONET AOD were strong for medium- (r = 0.91) and heavy-smoke (r = 0.90) density, and MAIAC estimates of AOD showed little bias relative to ground-based AERONET measurements (normalized mean bias = 3 % for medium, 5 % for heavy smoke). During two high AOD, heavy smoke episodes, MAIAC underestimated ground-based AERONET AOD under mixed aerosol (i.e., smoke and dust; median bias = -0.08) and overestimated AOD under smoke-dominated (median bias = 0.02) aerosol. MAIAC most overestimated ground-based AERONET AOD over barren land (mean NMB = 48 %). Our findings indicate that MODIS MAIAC can provide robust estimates of AOD as smoke density increases in coming years. Increased frequency of mixed aerosol and expansion of developed land could affect the performance of the MAIAC algorithm in the future, however, with implications for evaluating wildfire-associated health and welfare effects and air quality standards.
更多查看译文
AI 理解论文
溯源树
样例
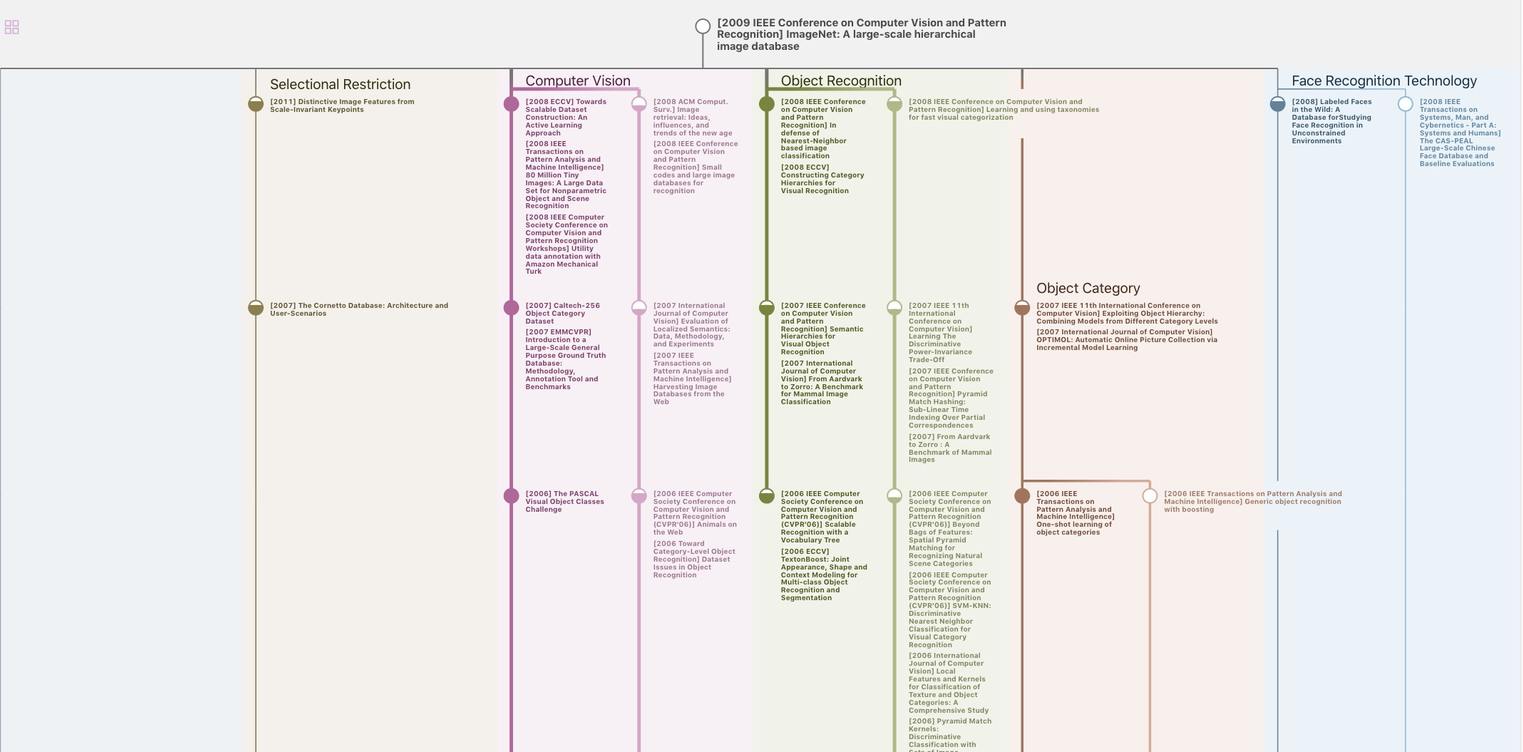
生成溯源树,研究论文发展脉络
Chat Paper
正在生成论文摘要