A Machine Learning Framework to Identify the Correlates of Disease Severity in Acute Arbovirus Infection
biorxiv(2024)
摘要
Most viral diseases display a variable clinical outcome due to differences in virus strain virulence and/or individual host susceptibility to infection. Understanding the biological mechanisms differentiating a viral infection displaying severe clinical manifestations from its milder forms can provide the intellectual framework toward therapies and early prognostic markers. This is especially true in arbovirus infections, where most clinical cases are present as mild febrile illness. Here, we used a naturally occurring vector-borne viral disease of ruminants, bluetongue, as an experimental system to uncover the fundamental mechanisms of virus-host interactions resulting in distinct clinical outcomes. As with most viral diseases, clinical symptoms in bluetongue can vary dramatically. We reproduced experimentally distinct clinical forms of bluetongue infection in sheep using three bluetongue virus (BTV) strains (BTV-1IT2006, BTV-1IT2013 and BTV-8FRA2017). Infected animals displayed clinical signs varying from clinically unapparent, to mild and severe disease. We collected and integrated clinical, haematological, virological, and histopathological data resulting in the analyses of 332 individual parameters from each infected and uninfected control animal. We subsequently used machine learning to identify the key viral and host processes associated with disease pathogenesis. We identified five different fundamental processes affecting the severity of bluetongue: (i) virus load and replication in target organs, (ii) modulation of the host type-I IFN response, (iii) pro-inflammatory responses, (iv) vascular damage, and (v) immunosuppression. Overall, our study using an agnostic machine learning approach, can be used to prioritise the different pathogenetic mechanisms affecting the disease outcome of an arbovirus infection.
### Competing Interest Statement
The authors have declared no competing interest.
更多查看译文
AI 理解论文
溯源树
样例
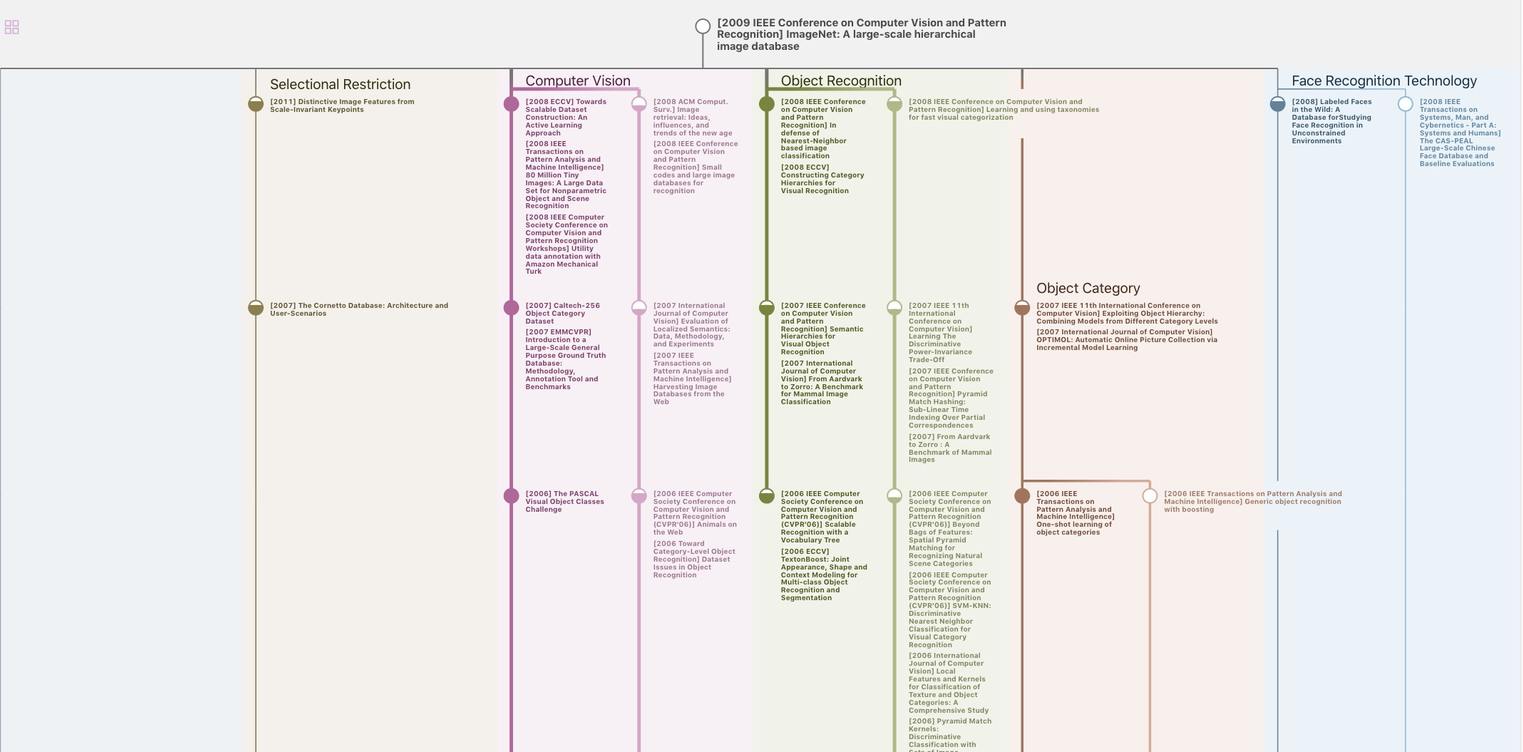
生成溯源树,研究论文发展脉络
Chat Paper
正在生成论文摘要