Advancing Drug-Target Interactions Prediction: Leveraging a Large-Scale Dataset with a Rapid and Robust Chemogenomic Algorithm.
biorxiv(2024)
摘要
Predicting drug-target interactions (DTIs) is crucial for drug discovery, and heavily relies on supervised learning techniques. In the context of DTI prediction, supervised learning algorithms use known DTIs to learn associations between molecule and protein features, allowing for the prediction of new interactions based on learned patterns. In this paper, we present a novel approach addressing two key challenges in DTI prediction: the availability of large, high-quality training datasets and the scalability of prediction methods. First, we introduce LCIdb, a curated, large-sized dataset of DTIs, offering extensive coverage of both the molecule and druggable protein spaces. Notably, LCIdb contains a much higher number of molecules, expanding coverage of the molecule space compared to traditional benchmarks. Second, we propose Komet (Kronecker Optimized METhod), a DTI prediction pipeline designed for scalability without compromising performance. Komet leverages a three-step framework, incorporating efficient computation choices tailored for large datasets and involving the Nystrom approximation. Specifically, Komet employs a Kronecker interaction module for (molecule, protein) pairs, which is sufficiently expressive and whose structure allows for reduced computational complexity. Our method is implemented in open-source software, leveraging GPU parallel computation for efficiency. We demonstrate the efficiency of our approach on various datasets, showing that Komet displays superior scalability and prediction performance compared to state-of-the-art deep-learning approaches. Additionally, we illustrate the generalization properties of Komet by showing its ability to solve challenging scaffold-hopping problems gathered in the publicly available LH benchmark. Komet is available open source at https://komet.readthedocs.io and all datasets, including LCIdb, can be found at https://zenodo.org/records/10731713.
### Competing Interest Statement
The authors have declared no competing interest.
更多查看译文
AI 理解论文
溯源树
样例
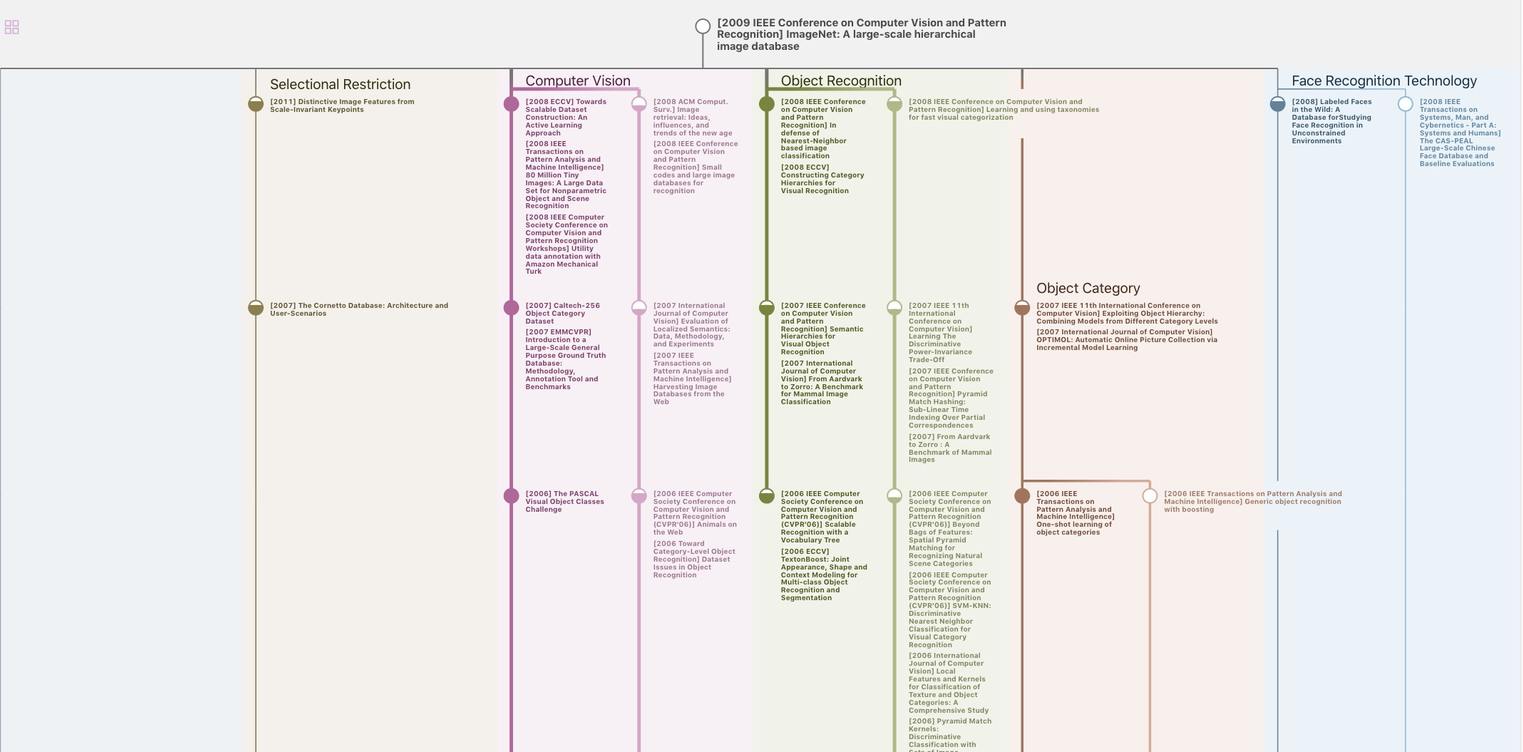
生成溯源树,研究论文发展脉络
Chat Paper
正在生成论文摘要