Automatic treatment planning for radiotherapy: a cross-modality and protocol study
arxiv(2024)
摘要
This study investigates the applicability of 3D dose predictions from a model
trained on one modality to a cross-modality automated planning workflow.
Additionally, we explore the impact of integrating a multi-criteria optimizer
on adapting predictions to different clinical preferences. Using a previously
created three-stage UNet in-house model trained on the 2020 AAPM OpenKBP
challenge dataset (340 head and neck plans, planned using 9-field static IMRT),
we retrospectively generated dose predictions for 20 patients. These dose
predictions were used to generate deliverable IMRT, VMAT, and Tomotherapy plans
using the fallback plan functionality in Raystation. The deliverable plans were
evaluated against the dose predictions based on primary clinical goals. A new
set of plans was also generated using MCO-based optimization with predicted
dose values as constraints. The mimicking approach accurately replicated the
predicted dose distributions across different modalities, with slight
deviations in spinal cord and external contour maximum doses. MCO customization
significantly reduced doses to OARs prioritized by our institution while
maintaining target coverage. All tested plans met clinical deliverability
standards, evidenced by a delivery QA gamma analysis passing rate above 98
Our findings show that a model trained only on IMRT plans can contribute to
planning across various modalities. Additionally, integrating predictions as
constraints in an MCO-based workflow, rather than direct dose mimicking,
enables a flexible, warm-start approach for treatment planning. Together, these
approaches have the potential to significantly decrease plan turnaround time
and quality variance, both at high resource medical centers that can train
in-house models, and smaller centers that can adapt a model from another
institution with minimal effort.
更多查看译文
AI 理解论文
溯源树
样例
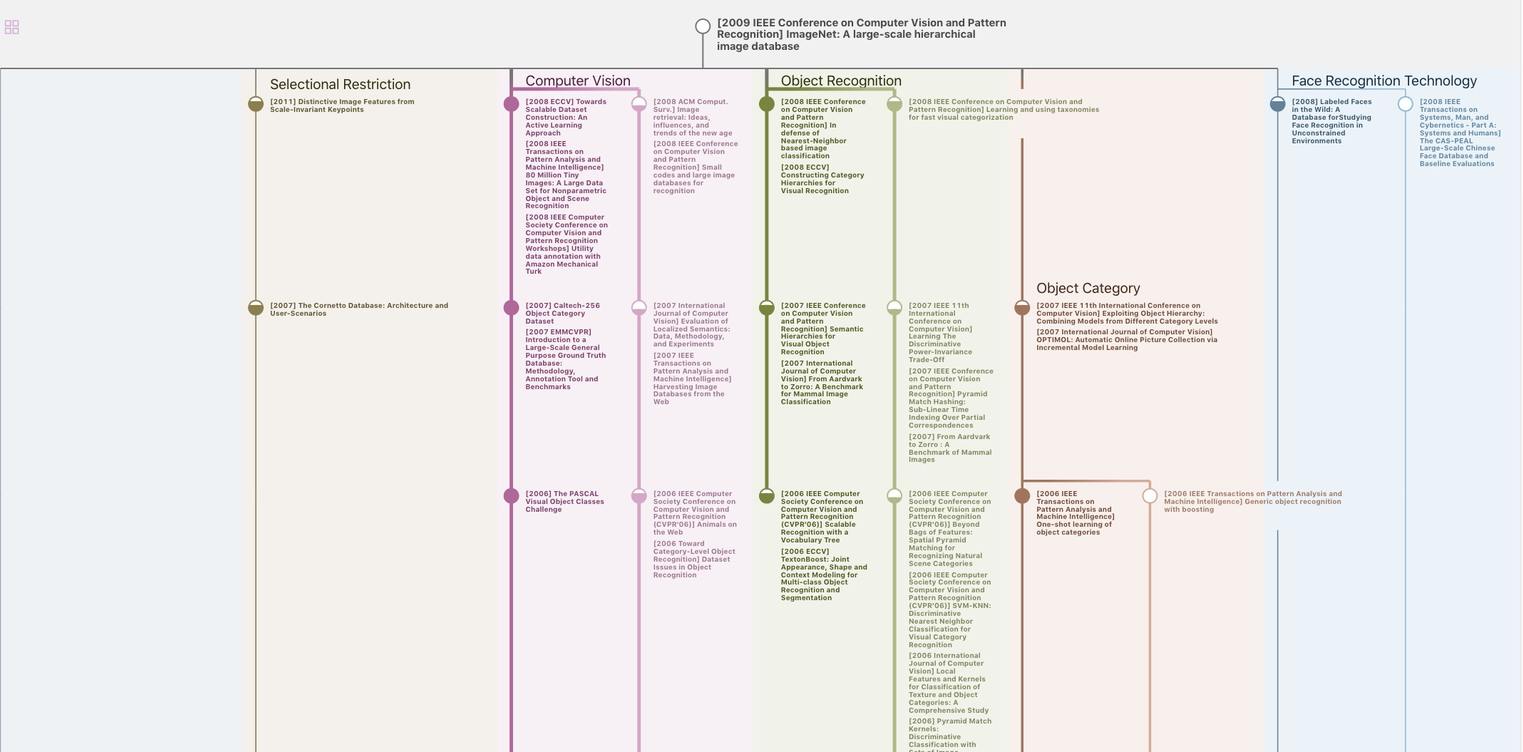
生成溯源树,研究论文发展脉络
Chat Paper
正在生成论文摘要