Conformalized-DeepONet: A Distribution-Free Framework for Uncertainty Quantification in Deep Operator Networks
CoRR(2024)
Abstract
In this paper, we adopt conformal prediction, a distribution-free uncertainty quantification (UQ) framework, to obtain confidence prediction intervals with coverage guarantees for Deep Operator Network (DeepONet) regression. Initially, we enhance the uncertainty quantification frameworks (B-DeepONet and Prob-DeepONet) previously proposed by the authors by using split conformal prediction. By combining conformal prediction with our Prob- and B-DeepONets, we effectively quantify uncertainty by generating rigorous confidence intervals for DeepONet prediction. Additionally, we design a novel Quantile-DeepONet that allows for a more natural use of split conformal prediction. We refer to this distribution-free effective uncertainty quantification framework as split conformal Quantile-DeepONet regression. Finally, we demonstrate the effectiveness of the proposed methods using various ordinary, partial differential equation numerical examples, and multi-fidelity learning.
MoreTranslated text
AI Read Science
Must-Reading Tree
Example
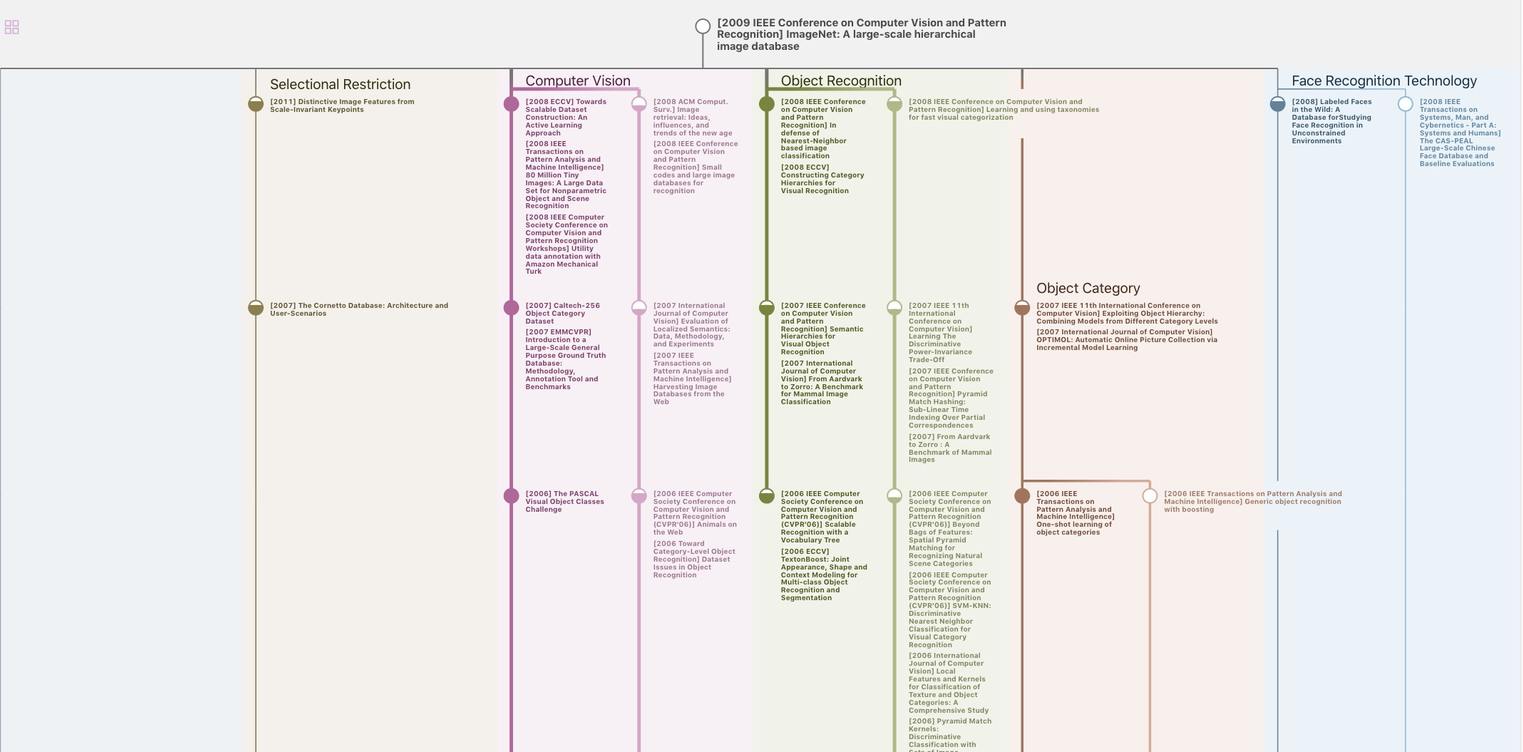
Generate MRT to find the research sequence of this paper
Chat Paper
Summary is being generated by the instructions you defined