How good are large language models for automated data extraction from randomized trials?
medrxiv(2024)
摘要
In evidence synthesis, data extraction is a crucial procedure, but it is time intensive and prone to human error. The rise of large language models (LLMs) in the field of artificial intelligence (AI) offers a solution to these problems through automation. In this case study, we evaluated the performance of two prominent LLM-based AI tools for use in automated data extraction. Randomized trials from two systematic reviews were used as part of the case study. Prompts related to each data extraction task (e.g., extract event counts of control group) were formulated separately for binary and continuous outcomes. The percentage of correct responses ( Pcorr ) was tested in 39 randomized controlled trials reporting 10 binary outcomes and 49 randomized controlled trials reporting one continuous outcome. The Pcorr and agreement across three runs for data extracted by two AI tools were compared with well-verified metadata. For the extraction of binary events in the treatment group across 10 outcomes, the Pcorr ranged from 40% to 87% and from 46% to 97% for ChatPDF and for Claude, respectively. For continuous outcomes, the Pcorr ranged from 33% to 39% across six tasks (Claude only). The agreement of the response between the three runs of each task was generally good, with Cohen’s kappa statistic ranging from 0.78 to 0.96 and from 0.65 to 0.82 for ChatPDF and Claude, respectively. Our results highlight the potential of ChatPDF and Claude for automated data extraction. Whilst promising, the percentage of correct responses is still unsatisfactory and therefore substantial improvements are needed for current AI tools to be adopted in research practice.
What is already known
What is new
Potential impact for Research Synthesis Methods readers outside the authors’ field
### Competing Interest Statement
The authors have declared no competing interest.
### Funding Statement
National Natural Science Foundation of China (72204003) Teachers Research Foundation Project of Nanjing University of Posts and Telecommunications (NYY222042)
### Author Declarations
I confirm all relevant ethical guidelines have been followed, and any necessary IRB and/or ethics committee approvals have been obtained.
Yes
I confirm that all necessary patient/participant consent has been obtained and the appropriate institutional forms have been archived, and that any patient/participant/sample identifiers included were not known to anyone (e.g., hospital staff, patients or participants themselves) outside the research group so cannot be used to identify individuals.
Yes
I understand that all clinical trials and any other prospective interventional studies must be registered with an ICMJE-approved registry, such as ClinicalTrials.gov. I confirm that any such study reported in the manuscript has been registered and the trial registration ID is provided (note: if posting a prospective study registered retrospectively, please provide a statement in the trial ID field explaining why the study was not registered in advance).
Yes
I have followed all appropriate research reporting guidelines, such as any relevant EQUATOR Network research reporting checklist(s) and other pertinent material, if applicable.
Yes
All data produced in the present work are contained in the manuscript
更多查看译文
AI 理解论文
溯源树
样例
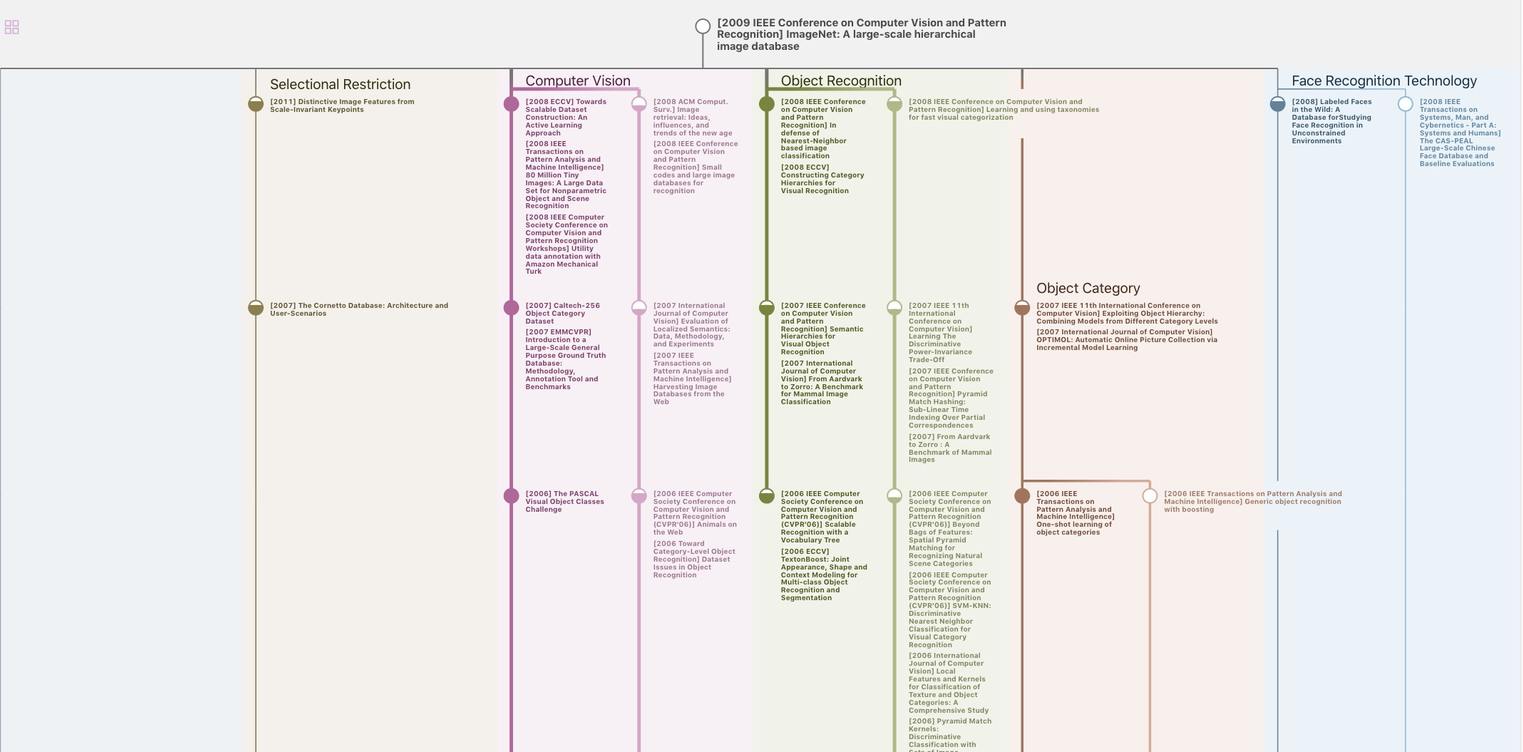
生成溯源树,研究论文发展脉络
Chat Paper
正在生成论文摘要