Defense against adversarial attacks based on color space transformation
NEURAL NETWORKS(2024)
摘要
Deep Learning algorithms have achieved state -of -the -art performance in various important tasks. However, recent studies have found that an elaborate perturbation may cause a network to misclassify, which is known as an adversarial attack. Based on current research, it is suggested that adversarial examples cannot be eliminated completely. Consequently, it is always possible to determine an attack that is effective against a defense model. We render existing adversarial examples invalid by altering the classification boundaries. Meanwhile, for valid adversarial examples generated against the defense model, the adversarial perturbations are increased so that they can be distinguished by the human eye. This paper proposes a method for implementing the abovementioned concepts through color space transformation. Experiments on CIFAR-10, CIFAR-100, and MiniImageNet demonstrate the effectiveness and versatility of our defense method. To the best of our knowledge, this is the first defense model based on the amplification of adversarial perturbations.
更多查看译文
关键词
Adversarial defense,Adversarial attack,Deep learning,Robustness
AI 理解论文
溯源树
样例
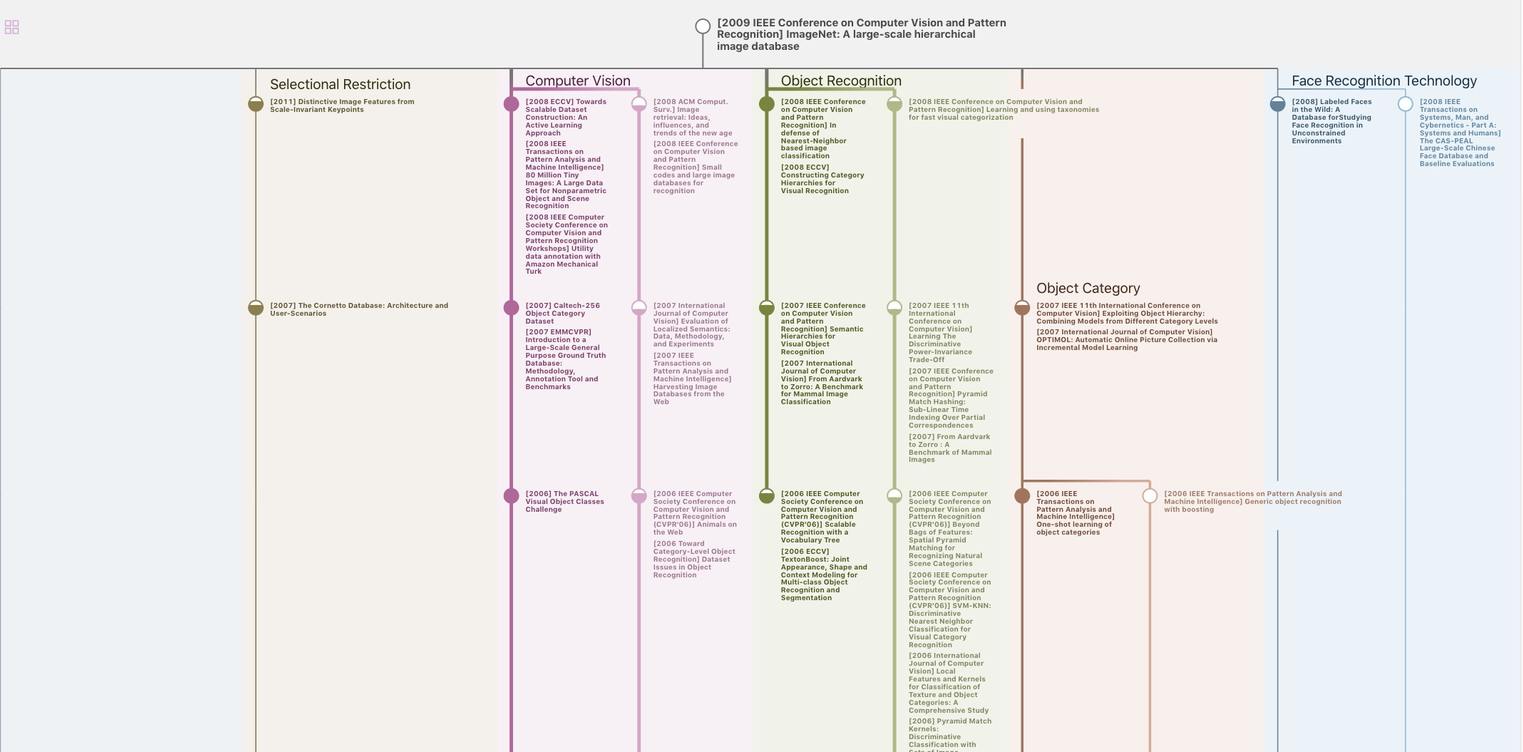
生成溯源树,研究论文发展脉络
Chat Paper
正在生成论文摘要