A deep-learning-based genomic status estimating framework for homologous recombination deficiency detection from low-pass whole genome sequencing
HELIYON(2024)
摘要
Genome-wide sequencing allows for prediction of clinical treatment responses and outcomes by estimating genomic status. Here, we developed Genomic Status scan (GSscan), a long short-term memory (LSTM)-based deep-learning framework, which utilizes low-pass whole genome sequencing (WGS) data to capture genomic instability-related features. In this study, GSscan directly surveys homologous recombination deficiency (HRD) status independent of other existing biomarkers. In breast cancer, GSscan achieved an AUC of 0.980 in simulated low-pass WGS data, and obtained a higher HRD risk score in clinical BRCA-deficient breast cancer samples (p = 1.3 x 10-4, compared with BRCA-intact samples). In ovarian cancer, GSscan obtained higher HRD risk scores in BRCA-deficient samples in both simulated data and clinical samples (p = 2.3 x 10-5 andp = 0.039, respectively, compared with BRCA-intact samples). Moreover, HRD-positive patients predicted by GSscan showed longer progression-free intervals in TCGA datasets (p = 0.0011) treated with platinum-based adjuvant chemotherapy, outperforming existing low-pass WGS-based methods. Furthermore, GSscan can accurately predict HRD status using only 1 ng of input DNA and a minimum sequencing coverage of 0.02 x , providing a reliable, accessible, and cost-effective approach. In summary, GSscan effectively and accurately detected HRD status, and provide a broadly applicable framework for disease diagnosis and selecting appropriate disease treatment.
更多查看译文
关键词
Homologous recombination deficiency,Deep learning,Genome sequencing
AI 理解论文
溯源树
样例
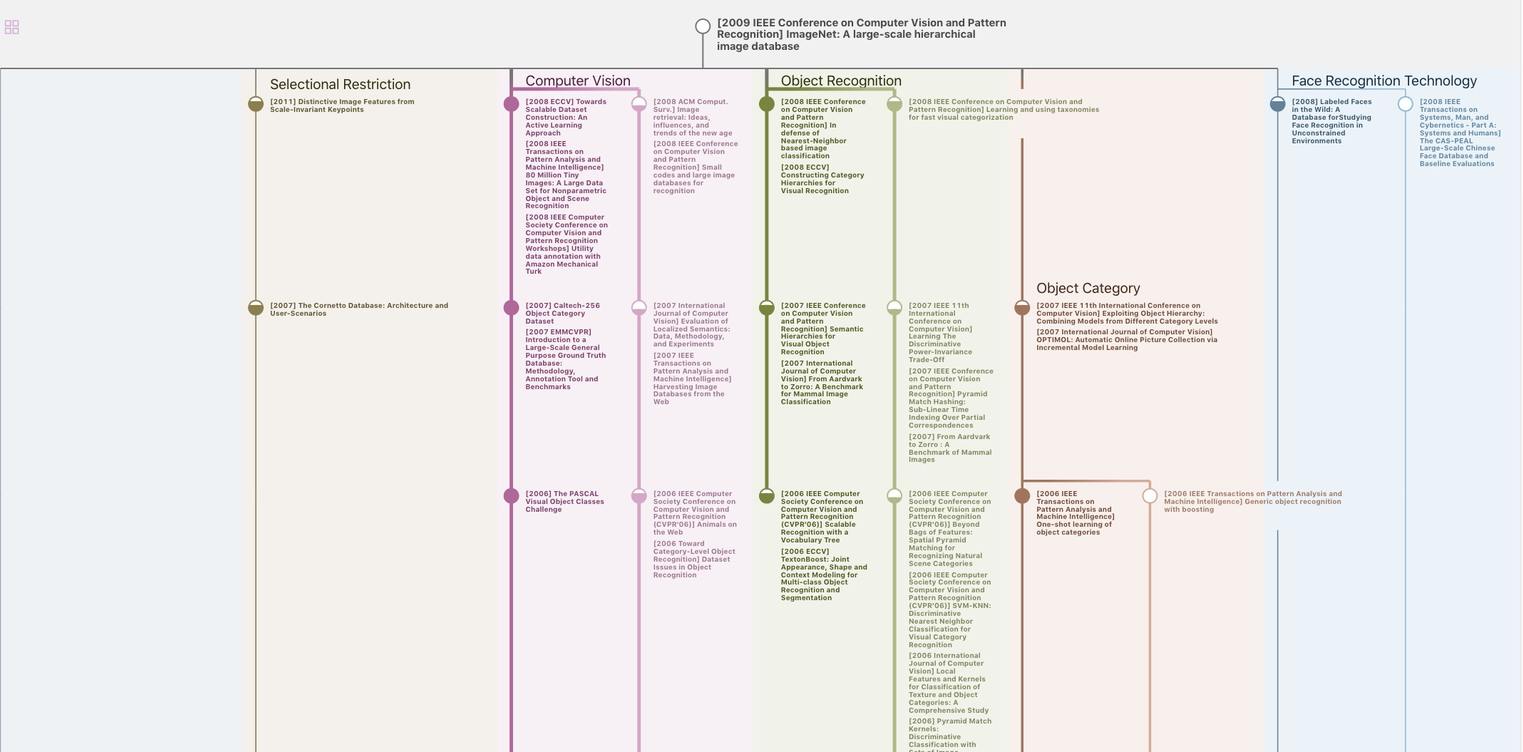
生成溯源树,研究论文发展脉络
Chat Paper
正在生成论文摘要