Lower bounds for quantum-inspired classical algorithms via communication complexity
CoRR(2024)
摘要
Quantum-inspired classical algorithms provide us with a new way to understand
the computational power of quantum computers for practically-relevant problems,
especially in machine learning. In the past several years, numerous efficient
algorithms for various tasks have been found, while an analysis of lower bounds
is still missing. Using communication complexity, in this work we propose the
first method to study lower bounds for these tasks. We mainly focus on lower
bounds for solving linear regressions, supervised clustering, principal
component analysis, recommendation systems, and Hamiltonian simulations. More
precisely, we show that for linear regressions, in the row-sparse case, the
lower bound is quadratic in the Frobenius norm of the underlying matrix, which
is tight. In the dense case, with an extra assumption on the accuracy we obtain
that the lower bound is quartic in the Frobenius norm, which matches the upper
bound. For supervised clustering, we obtain a tight lower bound that is quartic
in the Frobenius norm. For the other three tasks, we obtain a lower bound that
is quadratic in the Frobenius norm, and the known upper bound is quartic in the
Frobenius norm. Through this research, we find that large quantum speedup can
exist for sparse, high-rank, well-conditioned matrix-related problems. Finally,
we extend our method to study lower bounds analysis of quantum query algorithms
for matrix-related problems. Some applications are given.
更多查看译文
AI 理解论文
溯源树
样例
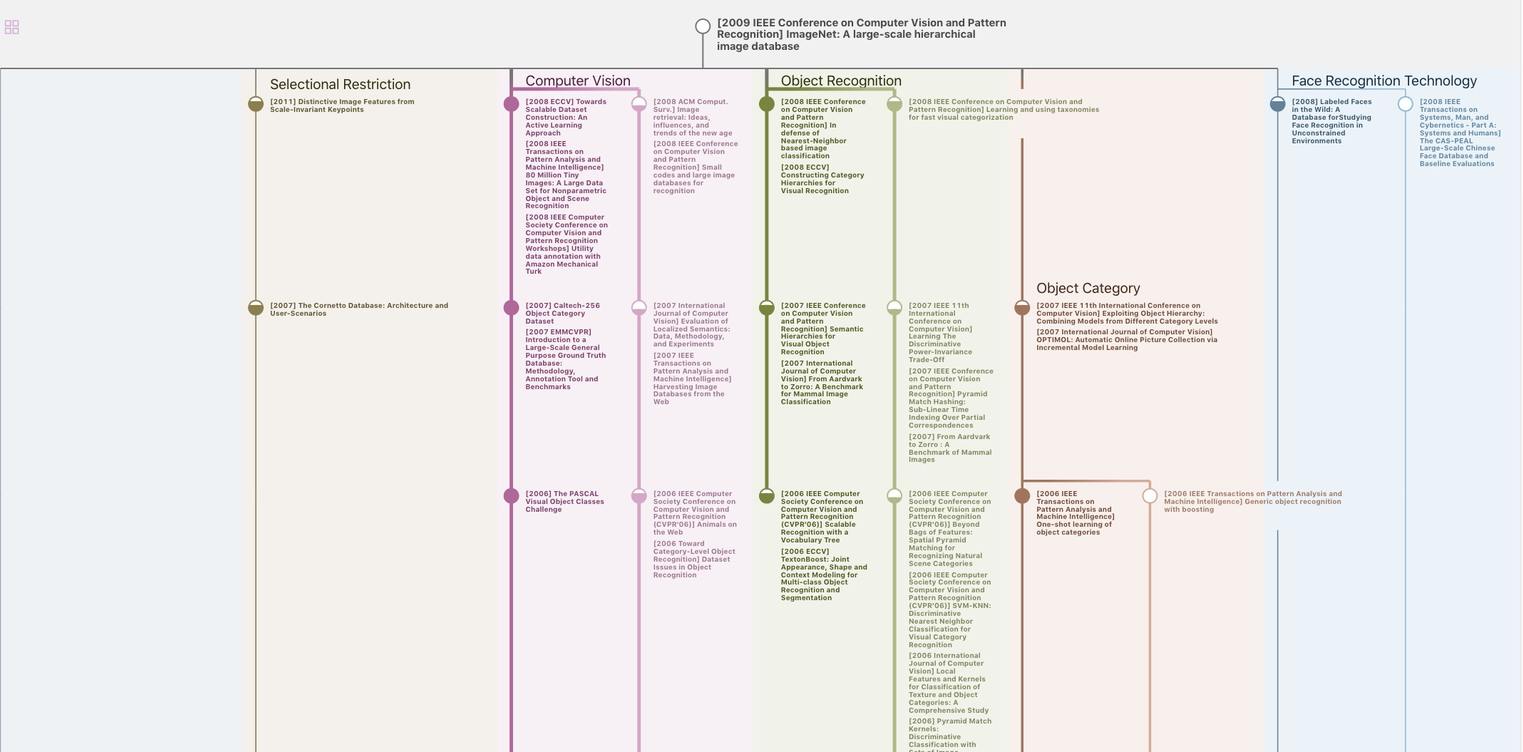
生成溯源树,研究论文发展脉络
Chat Paper
正在生成论文摘要