Offline Learning of Decision Functions in Multiplayer Games with Expectation Constraints
CoRR(2024)
摘要
We explore a class of stochastic multiplayer games where each player in the
game aims to optimize its objective under uncertainty and adheres to some
expectation constraints. The study employs an offline learning paradigm,
leveraging a pre-existing dataset containing auxiliary features. While prior
research in deterministic and stochastic multiplayer games primarily explored
vector-valued decisions, this work departs by considering function-valued
decisions that incorporate auxiliary features as input. We leverage the law of
large deviations and degree theory to establish the almost sure convergence of
the offline learning solution to the true solution as the number of data
samples increases. Finally, we demonstrate the validity of our method via a
multi-account portfolio optimization problem.
更多查看译文
AI 理解论文
溯源树
样例
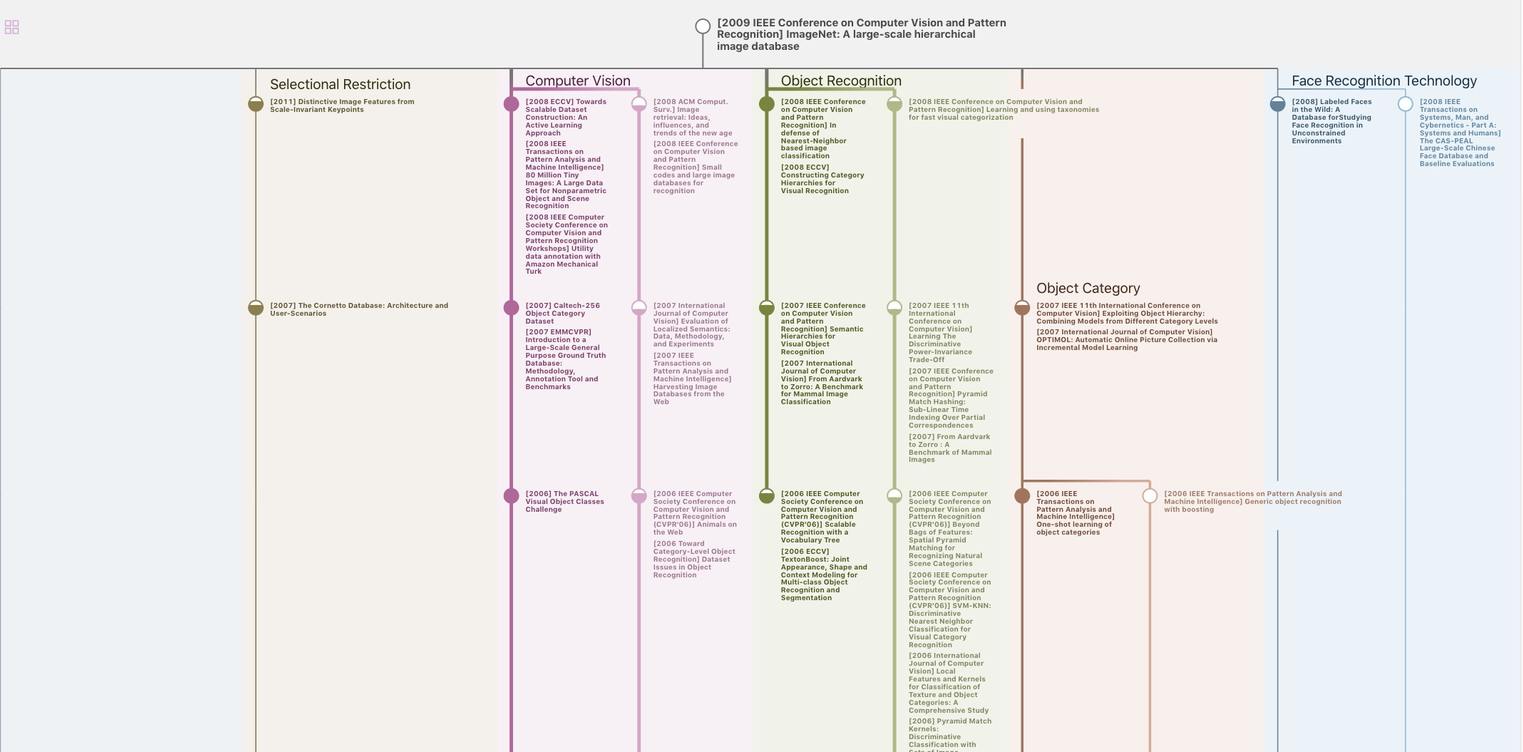
生成溯源树,研究论文发展脉络
Chat Paper
正在生成论文摘要