Understanding Missingness in Time-series Electronic Health Records for Individualized Representation
CoRR(2024)
摘要
With the widespread of machine learning models for healthcare applications,
there is increased interest in building applications for personalized medicine.
Despite the plethora of proposed research for personalized medicine, very few
focus on representing missingness and learning from the missingness patterns in
time-series Electronic Health Records (EHR) data. The lack of focus on
missingness representation in an individualized way limits the full utilization
of machine learning applications towards true personalization. In this brief
communication, we highlight new insights into patterns of missingness with
real-world examples and implications of missingness in EHRs. The insights in
this work aim to bridge the gap between theoretical assumptions and practical
observations in real-world EHRs. We hope this work will open new doors for
exploring directions for better representation in predictive modelling for true
personalization.
更多查看译文
AI 理解论文
溯源树
样例
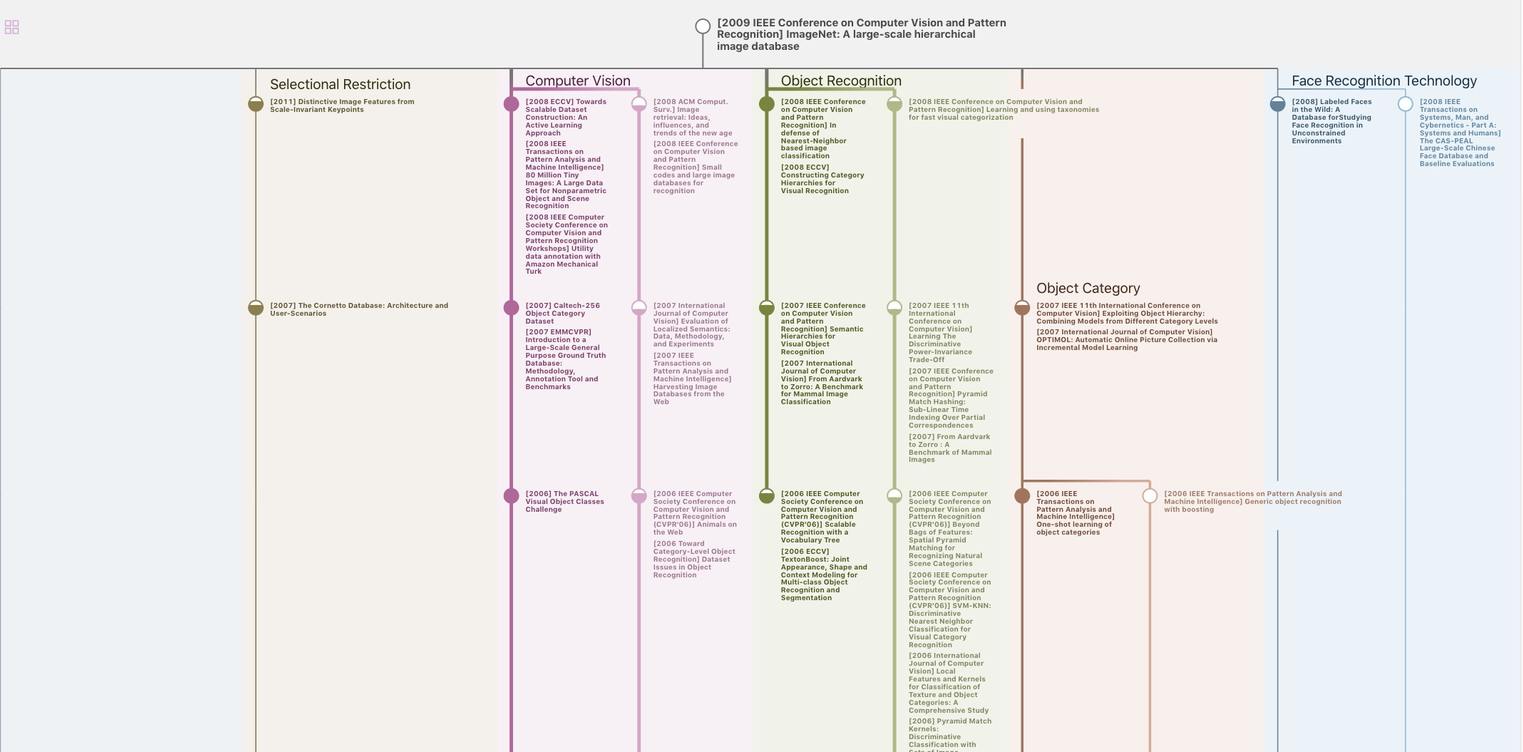
生成溯源树,研究论文发展脉络
Chat Paper
正在生成论文摘要