Inertial Accelerated Stochastic Mirror Descent for Large-Scale Generalized Tensor CP Decomposition
arxiv(2024)
摘要
The majority of classic tensor CP decomposition models are designed for
squared loss, employing Euclidean distance as a local proximal term. However,
the Euclidean distance is unsuitable for the generalized loss function
applicable to various types of real-world data, such as integer and binary
data. Consequently, algorithms developed under the squared loss are not easily
adaptable to handle these generalized losses, partially due to the lack of the
gradient Lipschitz continuity. This paper considers the generalized tensor CP
decomposition. We use the Bregman distance as the proximal term and propose an
inertial accelerated block randomized stochastic mirror descent algorithm
(iTableSMD). Within a broader multi-block variance reduction and inertial
acceleration framework, we demonstrate the sublinear convergence rate for the
subsequential sequence produced by the iTableSMD algorithm. We further show
that iTableSMD requires at most O(ϵ^-2) iterations in expectation to
attain an ϵ-stationary point and establish the global convergence of
the sequence. Numerical experiments on real datasets demonstrate that our
proposed algorithm is efficient and achieve better performance than the
existing state-of-the-art methods.
更多查看译文
AI 理解论文
溯源树
样例
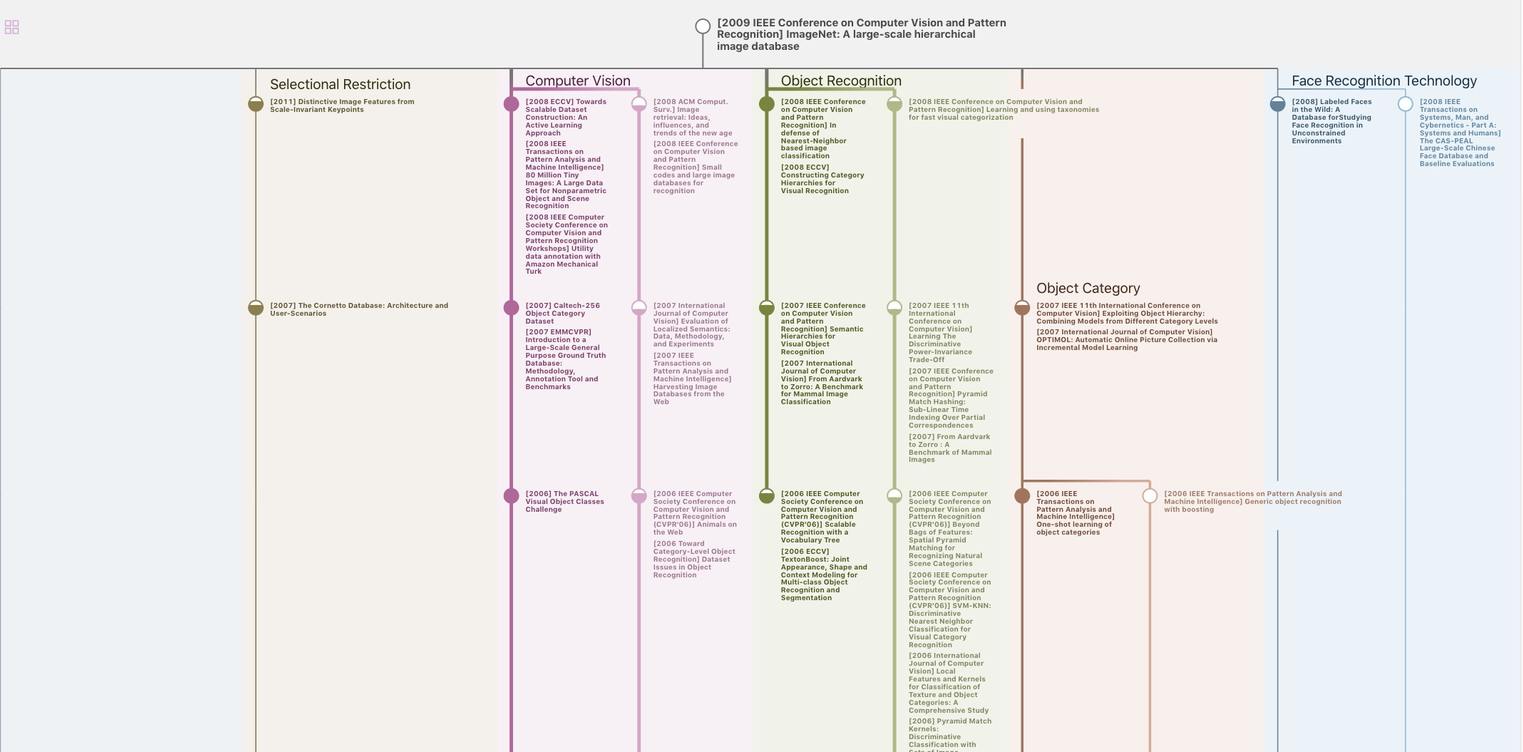
生成溯源树,研究论文发展脉络
Chat Paper
正在生成论文摘要