Mitigating the curse of dimensionality using feature projection techniques on electroencephalography datasets: an empirical review
Artificial Intelligence Review(2024)
摘要
Electroencephalography (EEG) is commonly employed to diagnose and monitor brain disorders, however, manual analysis is time-consuming. Hence, researchers nowadays are increasingly leveraging artificial intelligence (AI) techniques for automatic analysis of EEG, involving task-specific feature extraction and classification. Extracting multiple non-linear features from multiple EEG channels enhances the performance of classification, but it also results in high-dimensional features. However, the presence of the "curse of dimensionality" poses challenges for classifiers in AI-based EEG analyzers, leading to overfitting and complexity in classification. Therefore, in this empirical review, the effectiveness of the existing dimensionality reduction techniques to mitigate the curse of dimensionality in EEG feature sets is analyzed. We begin this study, by overviewing the extracted high-dimensional features from EEG signals related to disorders such as schizophrenia, alcoholism, focal seizures, focal seizures with deep features, and depression. Subsequently, 23 reduction techniques were reviewed, which transform the high-dimensional EEG features into a new reduced feature space to improve the classification. The impact of the reduced features was evaluated using traditional AI-based classifiers (support vector machine (SVM) and k -nearest neighbor ( k -NN)). 10-fold cross-validation was performed for training and testing, and the performance was evaluated using accuracy, selected features, and dimensionality reduction rate (DRR) metrics. Comprehensive analysis of projection techniques on diverse EEG datasets offers valuable insights to assist researchers in selecting the most suitable technique. The hybrid projection technique termed principal component analysis-based t -distributed stochastic neighbor embedding (PCA+ t -SNE) achieved an impressive average accuracy of 93.36%, surpassing the k -NN classifier without reduction techniques.
更多查看译文
关键词
Dimensionality reduction,EEG data,High dimension features,PCA+t-SNE
AI 理解论文
溯源树
样例
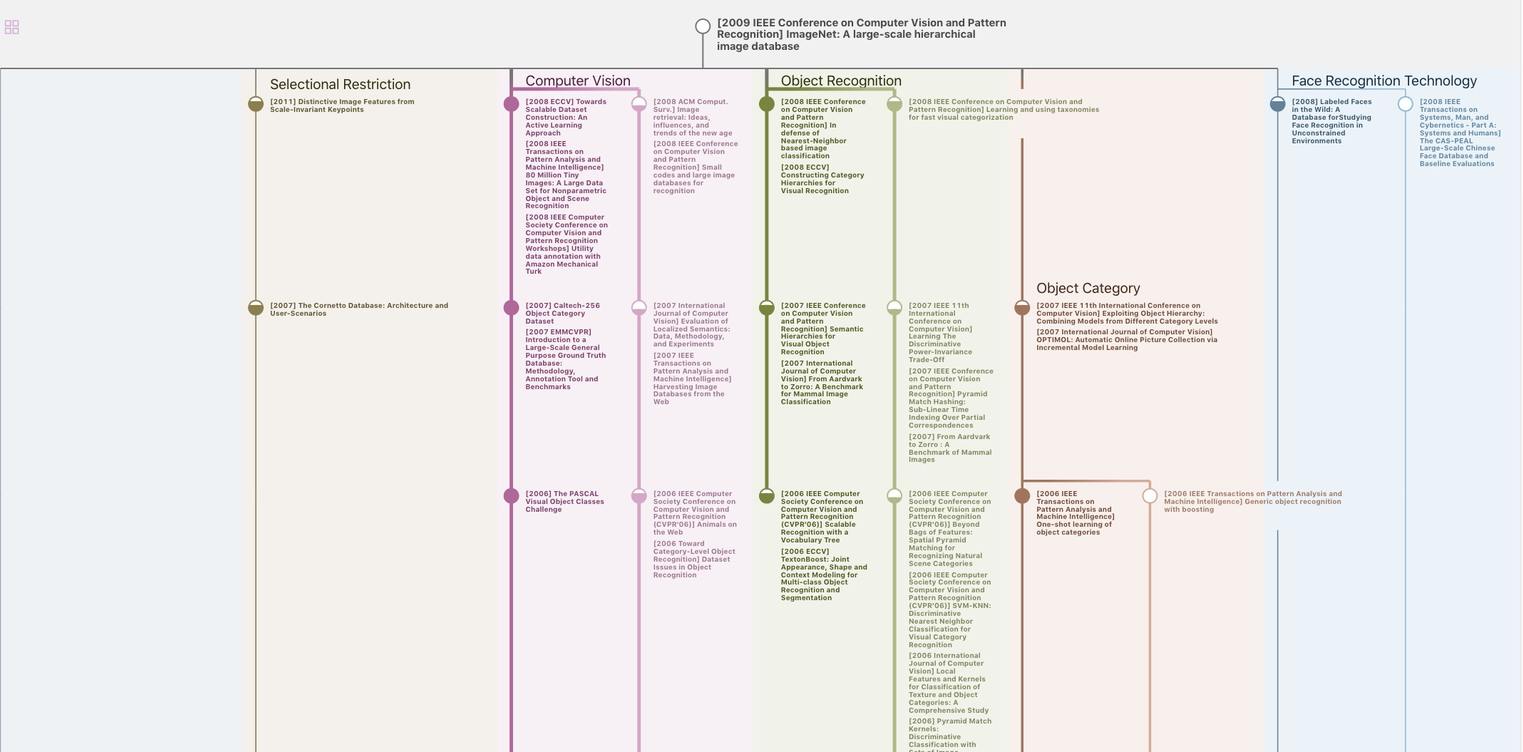
生成溯源树,研究论文发展脉络
Chat Paper
正在生成论文摘要