Data and Model Driven Task Offloading Strategy in the Dynamic Mobile Edge Computing System
Journal of Systems Science and Complexity(2024)
摘要
Mobile Edge Computing (MEC) provides communication and computational capabilities for the industrial Internet, meeting the demands of latency-sensitive tasks. Nevertheless, traditional model-driven task offloading strategies face challenges in adapting to situations with unknown network communication status and computational capabilities. This limitation becomes notably significant in complex industrial networks of high-speed railway. Motivated by these considerations, a data and model-driven task offloading problem is proposed in this paper. A redundant communication network is designed to adapt to anomalous channel states when tasks are offloaded to edge servers. The link switching mechanism is executed by the train according to the attributes of the completed task. The task offloading optimization problem is formulated by introducing data-driven prediction of communication states into the traditional model. Furthermore, the optimal strategy is achieved by employing the informer-based prediction algorithm and the quantum particle swarm optimization method, which effectively tackle real-time optimization problems due to their low time complexity. The simulations illustrate that the data and model-driven task offloading strategy can predict the communication state in advance, thus reducing the cost of the system and improving its robustness.
更多查看译文
关键词
Data driven model,informer,mobile edge computing,quantum particle swarm optimization,task offloading
AI 理解论文
溯源树
样例
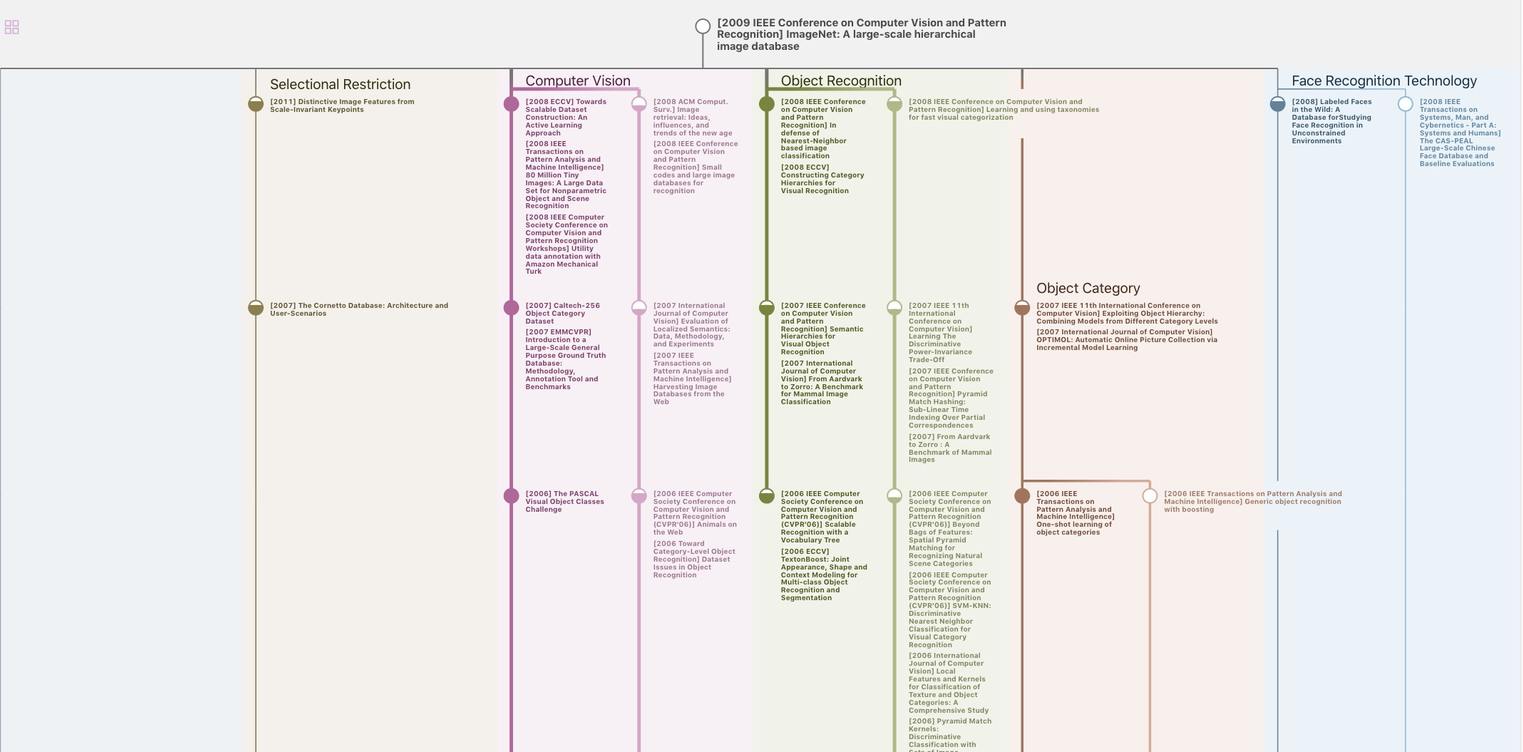
生成溯源树,研究论文发展脉络
Chat Paper
正在生成论文摘要