Enhancing 5G Network Slicing: Slice Isolation via Actor-Critic Reinforcement Learning with Optimal Graph Features
IEEE CONFERENCE ON GLOBAL COMMUNICATIONS, GLOBECOM(2023)
摘要
Network slicing within 5G networks encounters two significant challenges: catering to a maximum number of requests while ensuring slice isolation. To address these challenges, we present an innovative actor-critic Reinforcement Learning (RL) model named 'Slice Isolation based on RL' (SIRL). This model employs five optimal graph features to construct the problem environment, the structure of which is adapted using a ranking scheme. This scheme effectively reduces feature dimensionality and enhances learning performance. SIRL was assessed through a comparative analysis with nine state-of-the-art RL models, utilizing four evaluation metrics. The average results demonstrate that SIRL outperforms other models with a 70% higher coverage rate of requests and an 8% reduction in damage resulting from DoS/DDoS attacks.
更多查看译文
关键词
5G,beyond 5G,network slice,slice isolation,Reinforcement Learning (RL),Distributed Denial of Service (DDoS),and security.
AI 理解论文
溯源树
样例
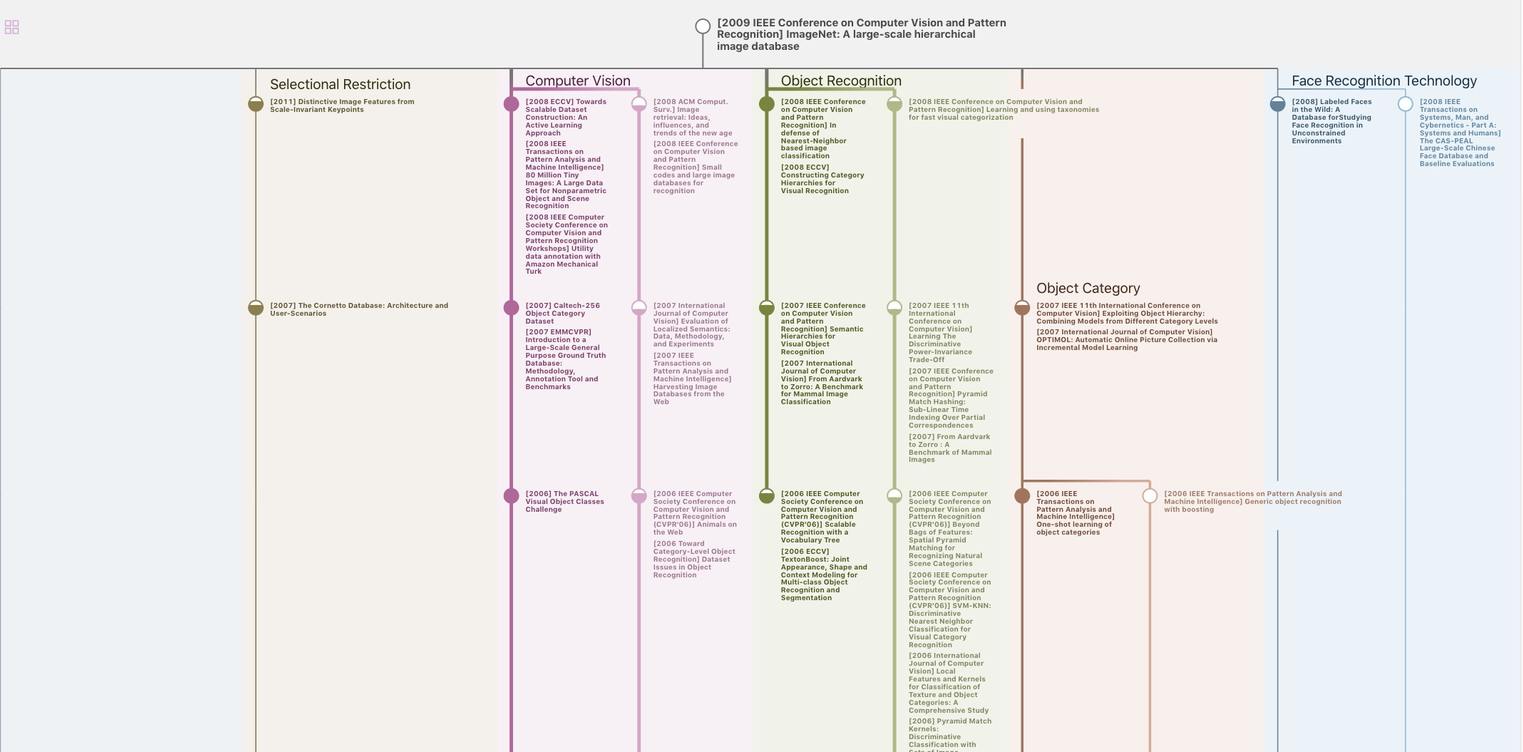
生成溯源树,研究论文发展脉络
Chat Paper
正在生成论文摘要