Deep-learning-based joint rigid and deformable contour propagation for magnetic resonance imaging-guided prostate radiotherapy
MEDICAL PHYSICS(2024)
摘要
BackgroundDeep learning-based unsupervised image registration has recently been proposed, promising fast registration. However, it has yet to be adopted in the online adaptive magnetic resonance imaging-guided radiotherapy (MRgRT) workflow.PurposeIn this paper, we design an unsupervised, joint rigid, and deformable registration framework for contour propagation in MRgRT of prostate cancer.MethodsThree-dimensional pelvic T2-weighted MRIs of 143 prostate cancer patients undergoing radiotherapy were collected and divided into 110, 13, and 20 patients for training, validation, and testing. We designed a framework using convolutional neural networks (CNNs) for rigid and deformable registration. We selected the deformable registration network architecture among U-Net, MS-D Net, and LapIRN and optimized the training strategy (end-to-end vs. sequential). The framework was compared against an iterative baseline registration. We evaluated registration accuracy (the Dice and Hausdorff distance of the prostate and bladder contours), structural similarity index, and folding percentage to compare the methods. We also evaluated the framework's robustness to rigid and elastic deformations and bias field perturbations.ResultsThe end-to-end trained framework comprising LapIRN for the deformable component achieved the best median (interquartile range) prostate and bladder Dice of 0.89 (0.85-0.91) and 0.86 (0.80-0.91), respectively. This accuracy was comparable to the iterative baseline registration: prostate and bladder Dice of 0.91 (0.88-0.93) and 0.86 (0.80-0.92). The best models complete rigid and deformable registration in 0.002 (0.0005) and 0.74 (0.43) s (Nvidia Tesla V100-PCIe 32 GB GPU), respectively. We found that the models are robust to translations up to 52 mm, rotations up to 15 circle$<^>\circ$, elastic deformations up to 40 mm, and bias fields.ConclusionsOur proposed unsupervised, deep learning-based registration framework can perform rigid and deformable registration in less than a second with contour propagation accuracy comparable with iterative registration.
更多查看译文
关键词
contour propagation,deep-learning-based image registration,MRI-guided radiotherapy
AI 理解论文
溯源树
样例
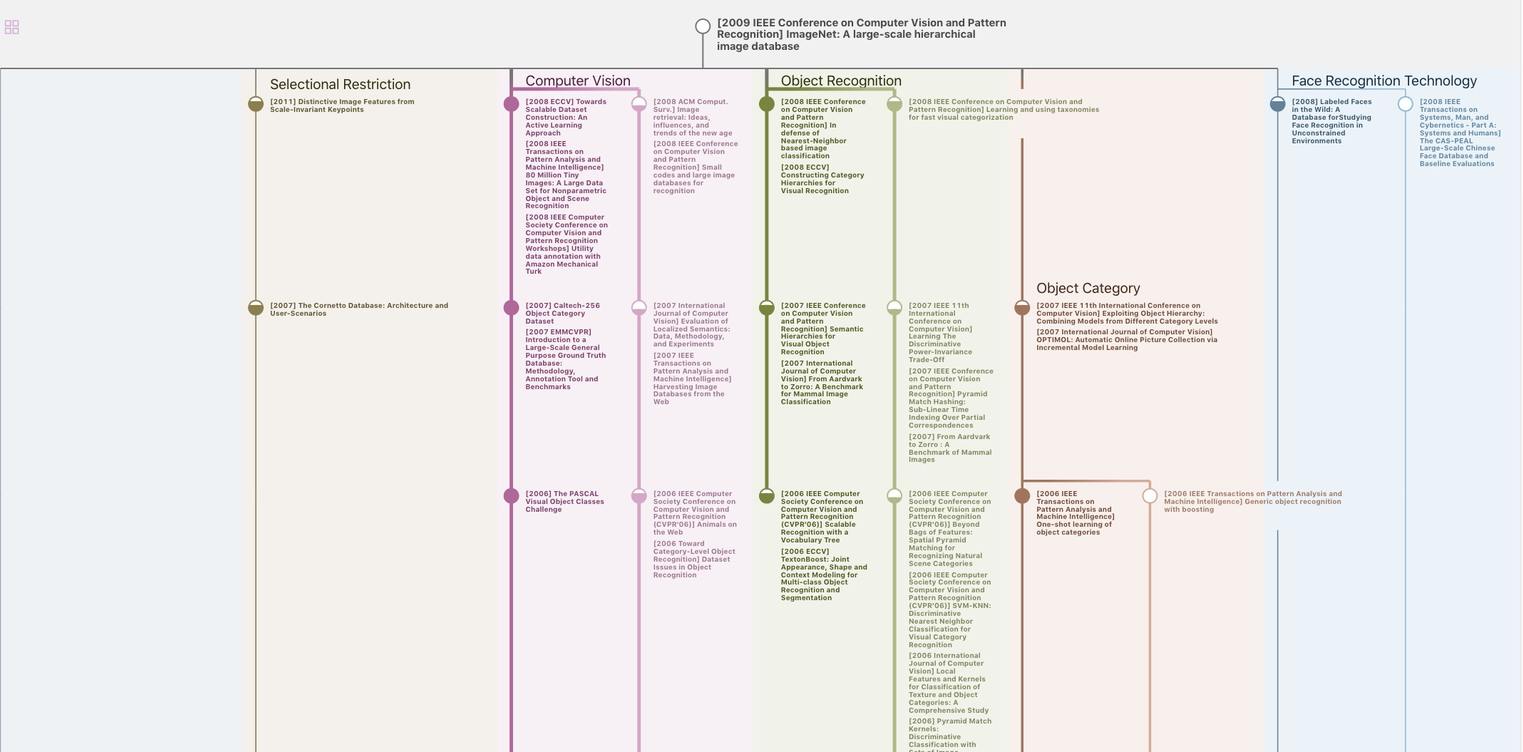
生成溯源树,研究论文发展脉络
Chat Paper
正在生成论文摘要