Joint Optimization for RIS-Aided Hybrid FSO SAGINs with Deep Reinforcement Learning
IEEE CONFERENCE ON GLOBAL COMMUNICATIONS, GLOBECOM(2023)
摘要
The trend of integrated satellite-HAP-ground networks (IS-HAP-GNs) as an critical directions for the future development of next generation network technology is widely recognized by academia and industry. Besides, utilizing reconfigurable intelligent surfaces (RIS) as a green paradigm, unmanned aerial vehicles (UAVs) can be equipped to reflect uplink signals from vehicle transmitters (VTs) to high altitude platforms (HAPs). Acting as relays, HAPs then forward these signals to satellites via hybrid free-space optical (FSO) links to enable rapid link deployment. In this paper, we firstly investigate a novel uplink signal transmission mode to maximize the system ergodic sum rate. Then, to tackle the high-dimensional non-convex optimization problems, we propose an asymmetric long short-term memory (LSTM)-deep deterministic policy gradient (DDPG) (AL-DDPG) algorithm builds on the deep reinforcement learning (DRL) framework. The numerical results demonstrate the superiority of the AL-DDPG algorithm over traditional optimization algorithms and reveal the effect of different system parameter settings on the performance.
更多查看译文
关键词
Integrated satellite-HAP-ground networks,hybrid FSO/RF links,reconfigurable intelligent surface (RIS),long short-term memory-deep deterministic policy gradient (LSTM-DDPG),deep reinforcement learning (DRL)
AI 理解论文
溯源树
样例
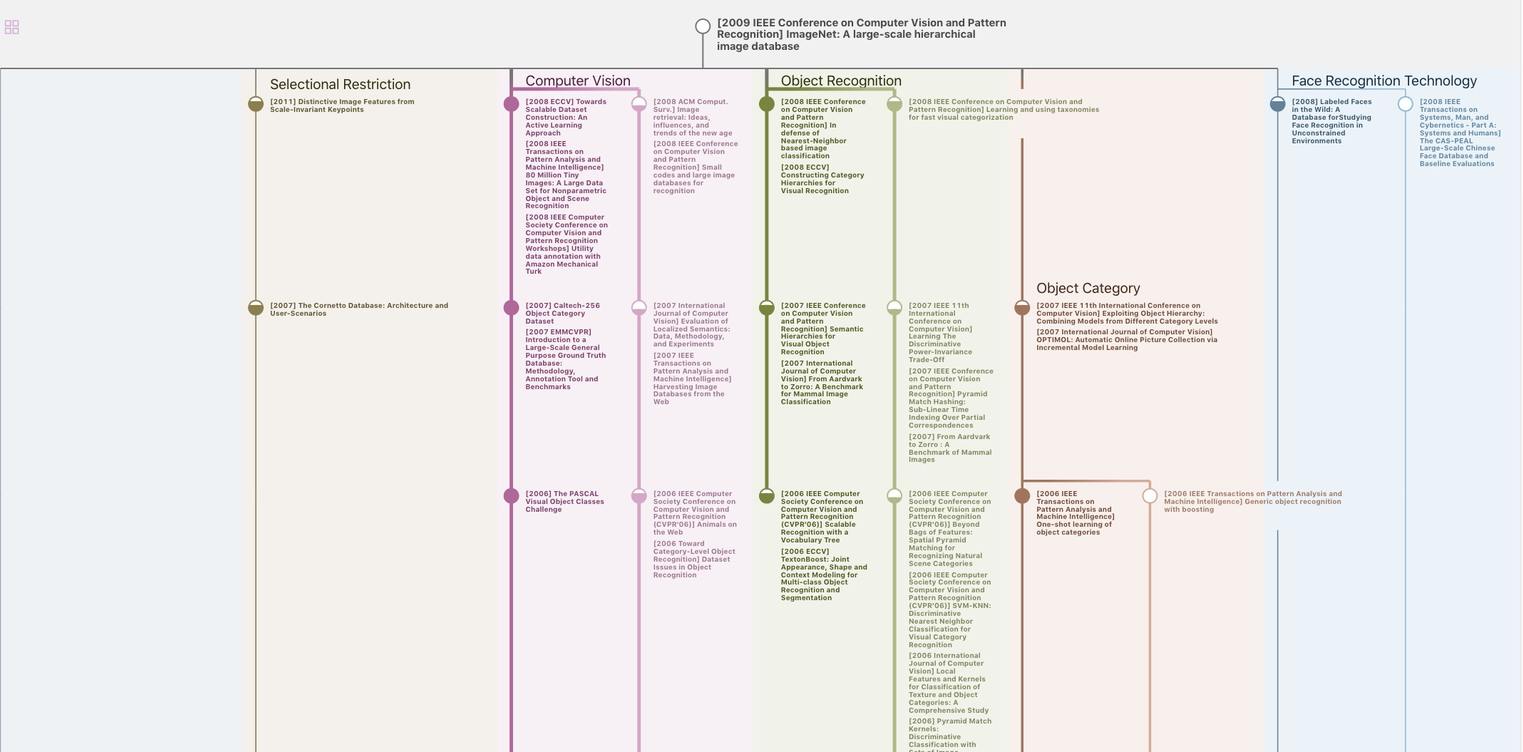
生成溯源树,研究论文发展脉络
Chat Paper
正在生成论文摘要