Asynchronous Federated Learning via Over-the-air Computation
IEEE CONFERENCE ON GLOBAL COMMUNICATIONS, GLOBECOM(2023)
摘要
The emerging field of federated learning (FL) provides great potential for edge intelligence while protecting data privacy. However, as the system grows in scale or becomes more heterogeneous, new challenges, such as the spectrum shortage and stragglers issues, arise. These issues can potentially be addressed by over-the-air computation (AirComp) and asynchronous FL, respectively, however, their combination is difficult due to their conflicting requirements. In this paper, we propose a novel asynchronous FL with AirComp in a time-triggered manner (async-AirFed). The conventional async aggregation requests the historical data to be used for model updates, which can cause the accumulation of channel noise and interference when AirComp is applied. To address this issue, we propose a simple but effective truncation method which retains a limited length of historical data. Convergence analysis presents that our proposed async-AirFed converges on non-convex optimality function with sublinear rate. Simulation results show that our proposed scheme achieves more than 34% faster convergence than the benchmarks, by achieving an accuracy of 85%, which also improves the time utilization efficiency and reduces the impact of staleness and the channel.
更多查看译文
关键词
Asynchronous federated learning,over-the-air computation,error accumulation
AI 理解论文
溯源树
样例
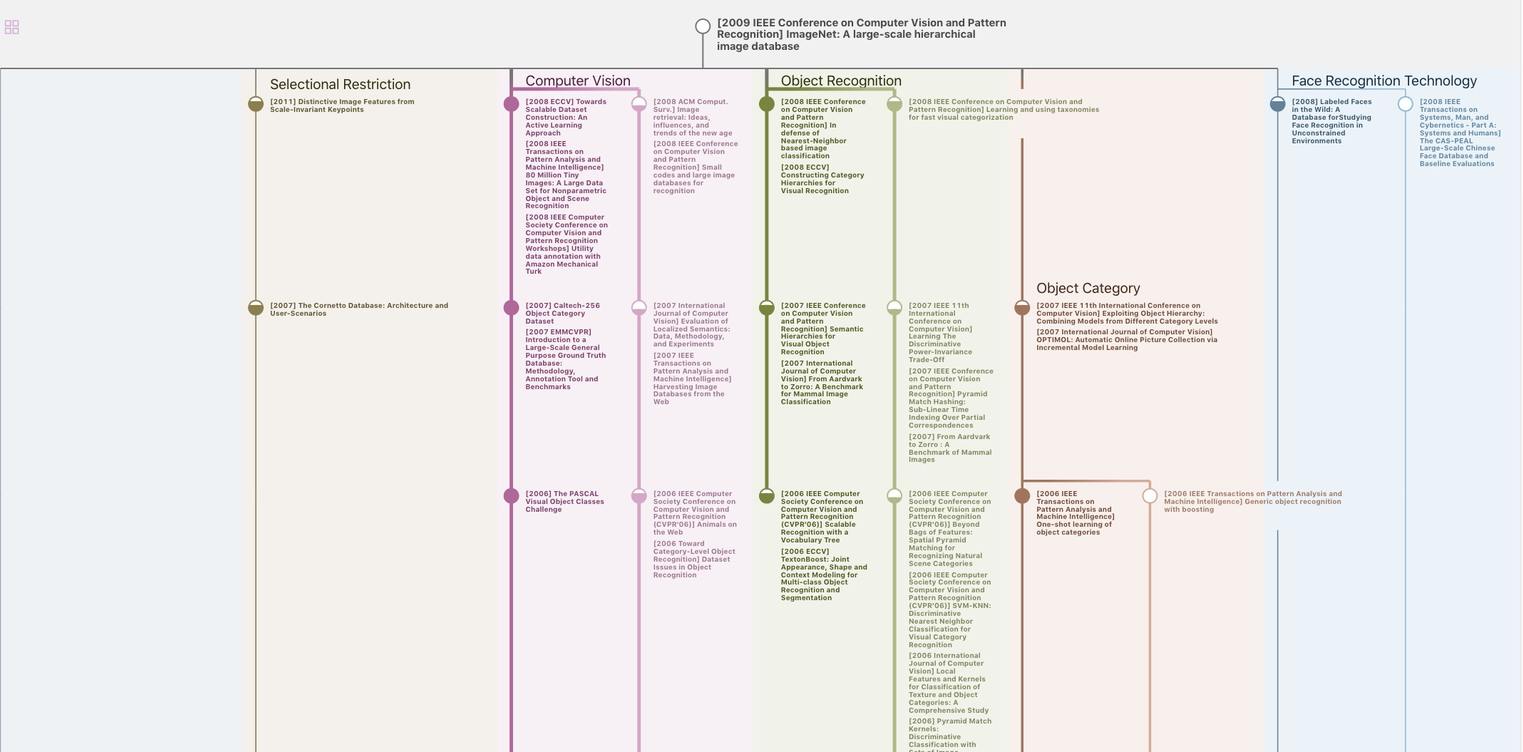
生成溯源树,研究论文发展脉络
Chat Paper
正在生成论文摘要