LEARN: Selecting Samples Without Training Verification for Communication-Efficient Vertical Federated Learning
IEEE CONFERENCE ON GLOBAL COMMUNICATIONS, GLOBECOM(2023)
摘要
In the classical vertical federated learning (VFL) framework, feature maps and corresponding gradient information of all samples are transferred between the server and clients, which causes a significant communication burden. Therefore, to enable efficient VFL in resource-constrained wireless networks, we propose to select a part of the samples from the large training set to train models with minimal accuracy degradation. To this end, we propose LEARN, i.e., seLecting Efficient sAmples without tRaining verificatioN, to select efficient training samples for VFL. Particularly, LEARN integrates two major components named label distribution smoothing and feature center-based vertical sample filtering. The number of samples selected for each class is determined by the label distribution smoothing mechanism. Then the feature center-based vertical sample filtering component calculates the features centers and performs sample selection based on the distance between the samples and their corresponding feature center. Extensive experiments under various settings are carried out to corroborate the efficacy and robustness of LEARN.
更多查看译文
关键词
Vertical Federated Learning,Data Sampling,Communication Overhead,Wireless Network
AI 理解论文
溯源树
样例
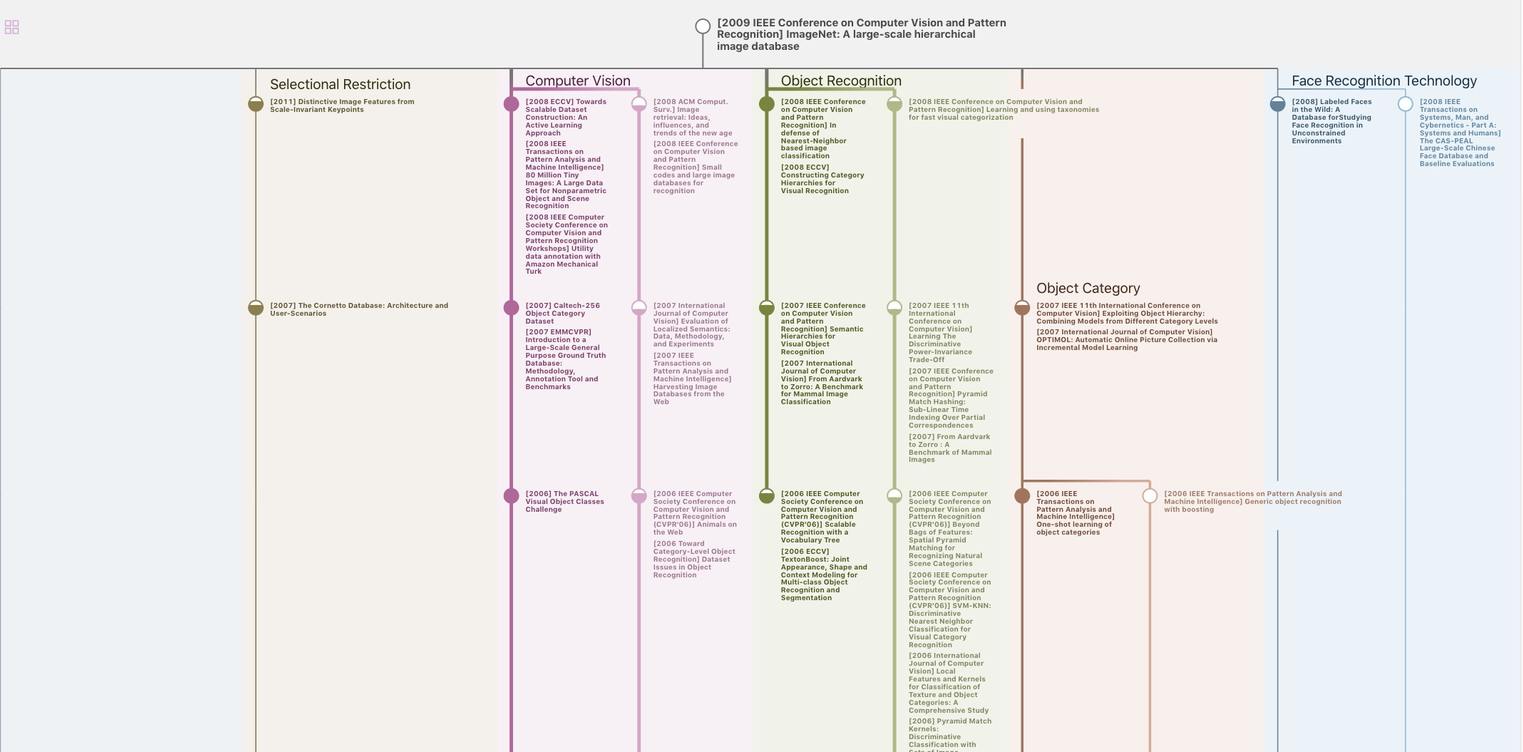
生成溯源树,研究论文发展脉络
Chat Paper
正在生成论文摘要