DTPP-DFL: A Dropout-Tolerated Privacy-Preserving Decentralized Federated Learning Framework
IEEE CONFERENCE ON GLOBAL COMMUNICATIONS, GLOBECOM(2023)
摘要
Federated Learning (FL) enables participants to collaboratively train a global model by sharing their gradients without the need for uploading privacy-sensitive data. Despite certain privacy preservation of FL, local gradients in plaintext may reveal data privacy when gradient-leakage attacks are launched. To further protect local gradients, privacy-preserving FL schemes have been proposed. However, these existing schemes that require a fully trusted central server are vulnerable to a single point of failure and malicious attacks. Although more robust privacy-preserving decentralized FL schemes have recently been proposed on multiple servers, they will fail to aggregate the local gradients with message transmission errors or data packet dropping out due to the instability of the communication network. To address these challenges, we propose a novel privacy-preserving decentralized FL scheme system based on the blockchain and a modified identity-based homomorphic broadcast encryption algorithm. This scheme achieves both privacy protection and error/dropout tolerance. Security analysis shows that the proposed scheme can protect the privacy of the local gradients against both internal and external adversaries, and protect the privacy of the global gradients against external adversaries. Moreover, it ensures the correctness of local gradients' aggregation even when transmission error or data packet dropout happens. Extensive experiments demonstrate that the proposed scheme guarantees model accuracy and achieves performance efficiency.
更多查看译文
关键词
Privacy-Preserving,Dropout-Tolerated,Decentralized,Federated Learning,Blockchain
AI 理解论文
溯源树
样例
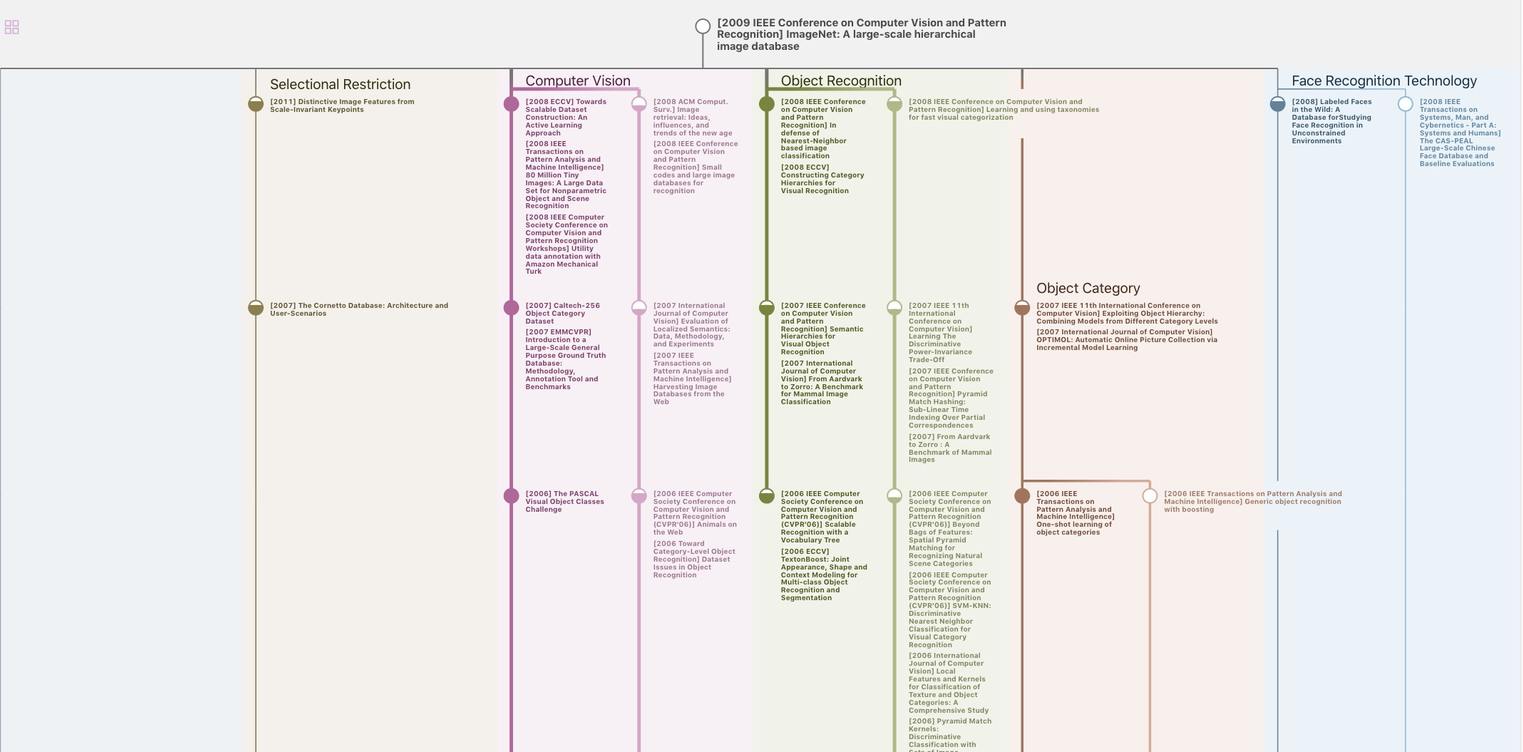
生成溯源树,研究论文发展脉络
Chat Paper
正在生成论文摘要