A From-Scratch Approach to Deep Unfolding-Aided Bilinear Gaussian Belief Propagation for Correlated Large MIMO
IEEE CONFERENCE ON GLOBAL COMMUNICATIONS, GLOBECOM(2023)
摘要
This paper proposes a trainable bilinear Gaussian belief propagation (BiGaBP) aided by deep unfolding built from scratch. In Massive MIMO scenarios, joint channel and data estimation (JCDE) based on belief propagation (BP) has been achieved with low computational complexity through bilinear generalized approximate message passing (BiGAMP) and bilinear Gaussian belief propagation (BiGaBP). However, although BiGaBP has a lower dependence on the large-system limit approximation compared to BiGAMP, both message-passing algorithms are still derived based on the large system assuming independent and identically distributed (i.i.d.) observations with mean zero, resulting in significant performance degradation in the presence of spatial fading correlation. To tackle this issue, we introduce the parameters in BiGaBP for belief design, which were previously set manually in conventional method, as trainable parameters and improve performance by conducting data-driven tuning under spatially correlated fading. We conduct computer simulations from scratch based on a theoretically derived iterative error backpropagation algorithm from the signal flow graph, demonstrating the effectiveness of the proposed method in terms of bit error rate (BER) and achieving transparency in deep unfolding.
更多查看译文
关键词
Belief Propagation,Multi-input Multi-output,Gaussian Belief Propagation,Computational Complexity,Computer Simulations,Iterative Algorithm,Performance Degradation,Trainable Parameters,Bit Error Rate,Bit Error,Channel Estimation,Degradation In The Presence,Signal Detection,Discrete-time,Probability Density Function,Scale Parameter,Additive Noise,Stochastic Gradient Descent,Weight Parameters,User Equipment,Posterior Probability Density Function,Factor Graph,Antenna Array,Central Limit Theorem,Maximum A Posteriori,Variable Nodes,Data Symbols,Multi-input Multi-output Systems,Least Squares Estimation
AI 理解论文
溯源树
样例
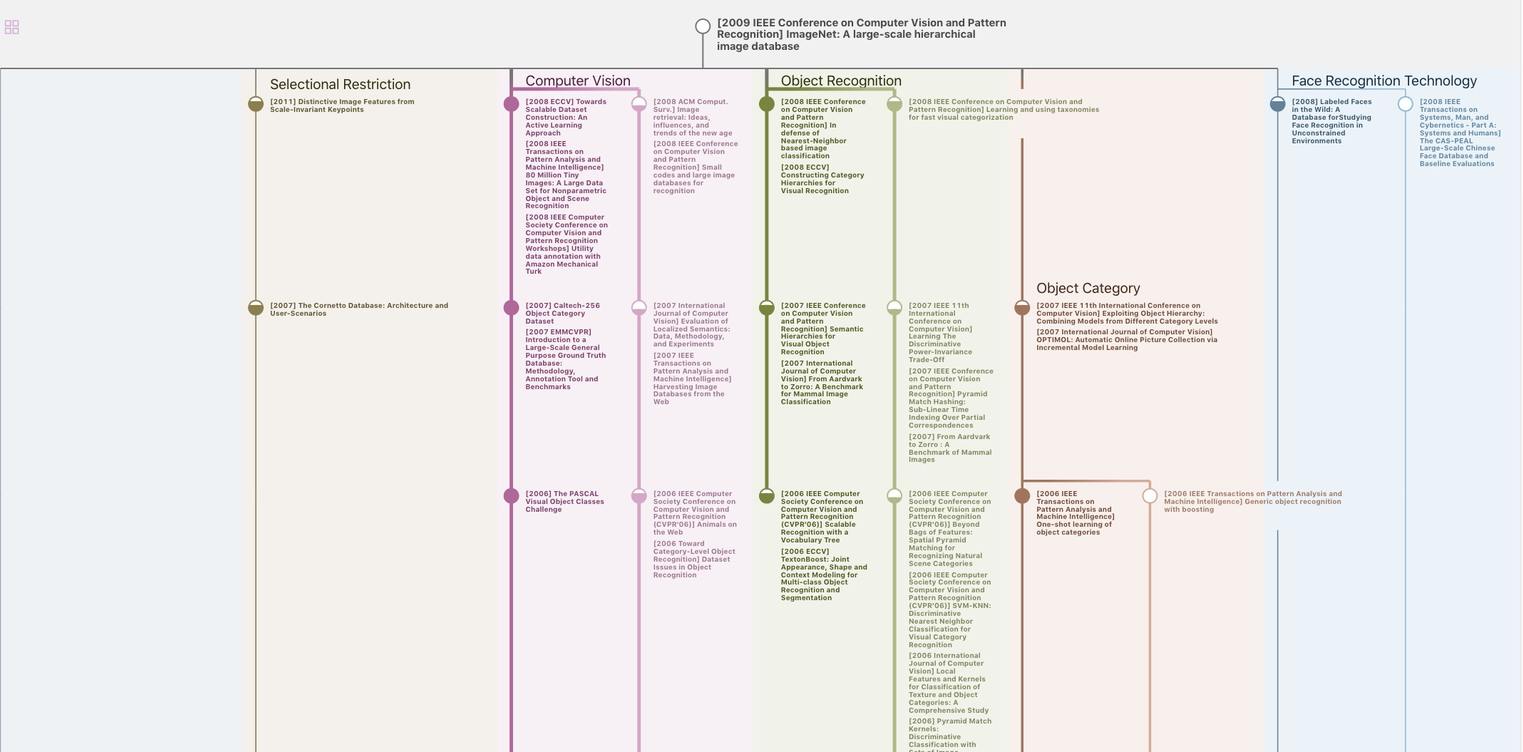
生成溯源树,研究论文发展脉络
Chat Paper
正在生成论文摘要