A Graph-based Spatial-Temporal Deep Reinforcement Learning Model for Edge Caching
IEEE CONFERENCE ON GLOBAL COMMUNICATIONS, GLOBECOM(2023)
摘要
With increased Internet users, backhaul links are experiencing unprecedented traffic burdens. Meanwhile, edge caching is emerging to reduce the burden. In order to optimize edge caching efficiency, this paper proposes an intelligent caching strategy called "spatial-temporal graph attention network-soft actor-critic" (STGAN-SAC). STGAN-SAC is fully decentralized and makes caching decisions without prior knowledge of the content popularity. In addition, it takes user mobility into account and enables cooperative caching between neighbouring base stations (BSs). Our paper is the first to apply spatial-temporal models to the caching problem, and experimental results have demonstrated their importance in solving this problem. STGAN-SAC achieves at least a 22.4% higher cache hit ratio, 2.1% lower latency and 2.5% lower backhaul link load compared to the state-of-the-art caching policy DDRQN. Moreover, STGAN-SAC achieves at least a 51.4% higher cache hit ratio, 3.7% lower latency and 2.1% lower backhaul link load than the state-of-the-art caching strategy DDGARQN.
更多查看译文
关键词
edge caching,spatial-temporal graph attention network,multi-agent reinforcement learning,soft actor-critic
AI 理解论文
溯源树
样例
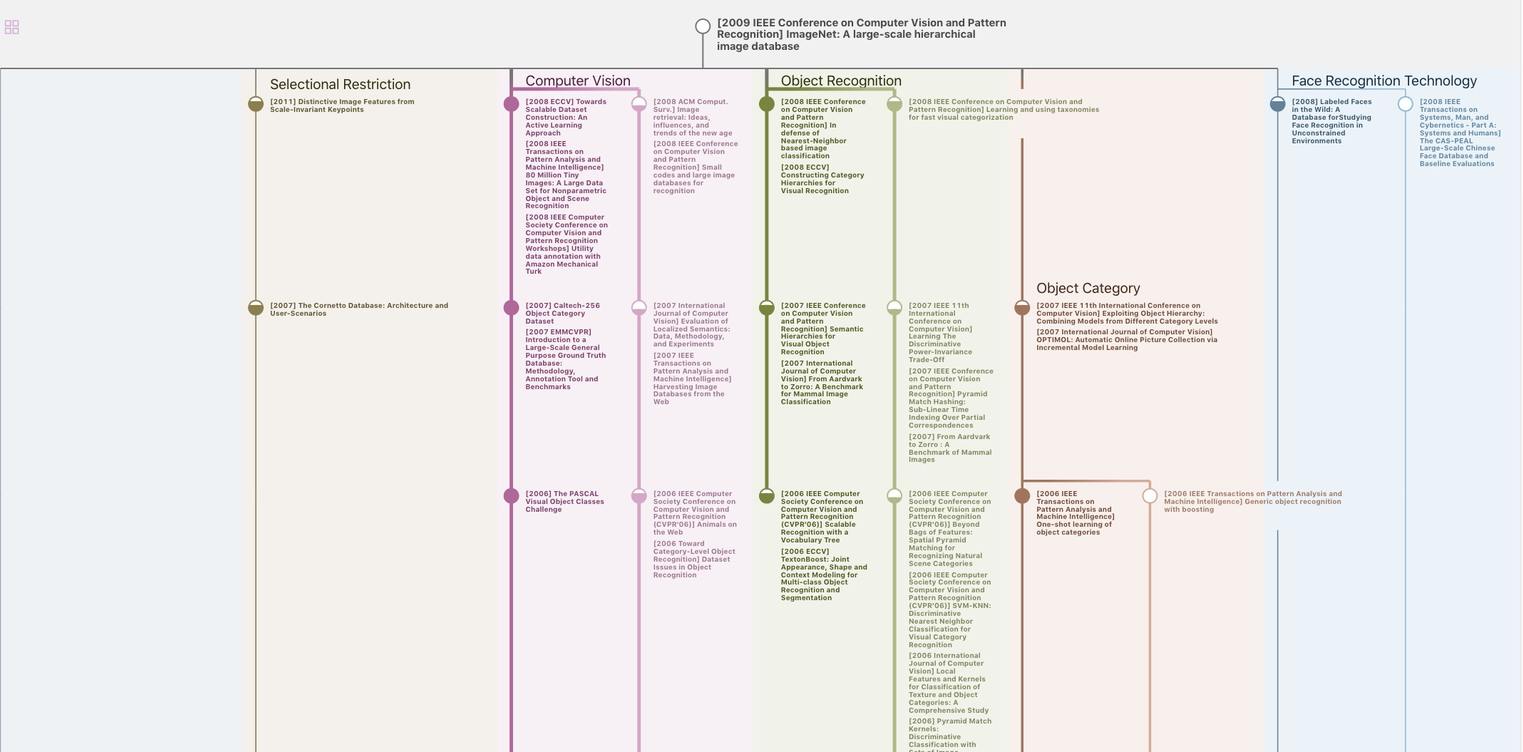
生成溯源树,研究论文发展脉络
Chat Paper
正在生成论文摘要