Split Learning for Sensing-Aided Single and Multi-Level Beam Selection in Multi-Vendor RAN
IEEE CONFERENCE ON GLOBAL COMMUNICATIONS, GLOBECOM(2023)
摘要
Proper and efficient beam selection is of great importance to unleash the full potential of mmWave communications. Traditionally, each candidate beam is evaluated using reference signals (beam sweeping), however, the exhaustive search method can be time-consuming with high signaling overhead. To avoid such problems, in B5G and 6G, sensing information is considered to be used, as in Integrated Sensing and Communication (ISAC) solutions, and Machine Learning (ML) methods can be applied to map sensing data inputs to an optimal beam index. When using sensing information sources external to the Radio Access Network (RAN) in a multi-vendor disaggregated environment, those methods need to account for issues such as privacy and data ownership. In this work, we apply multi-modal sensing information to the beam selection task. Specifically, we propose a multi-modal sensing-aided ML strategy based on Split Learning (SL) that can cope with deployment challenges in novel RAN architectures. Moreover, the method is applied to single and multi-level beam selection decisions, where the latter considers the case of hierarchical codebook structures. With the proposed approach, accuracy levels above 90% can be achieved while overhead diminishes by 85% or more. SL achieves comparable performance with centralized learning-based strategies, with the added value of accounting for privacy and data ownership issues. We also show that sensing-aided ML-based beam selection decisions in multi-level codebooks are more effective when applied to their first level.
更多查看译文
关键词
beam selection,multi-modal sensing,split learning,integrated sensing and communication (ISAC),B5G,6G
AI 理解论文
溯源树
样例
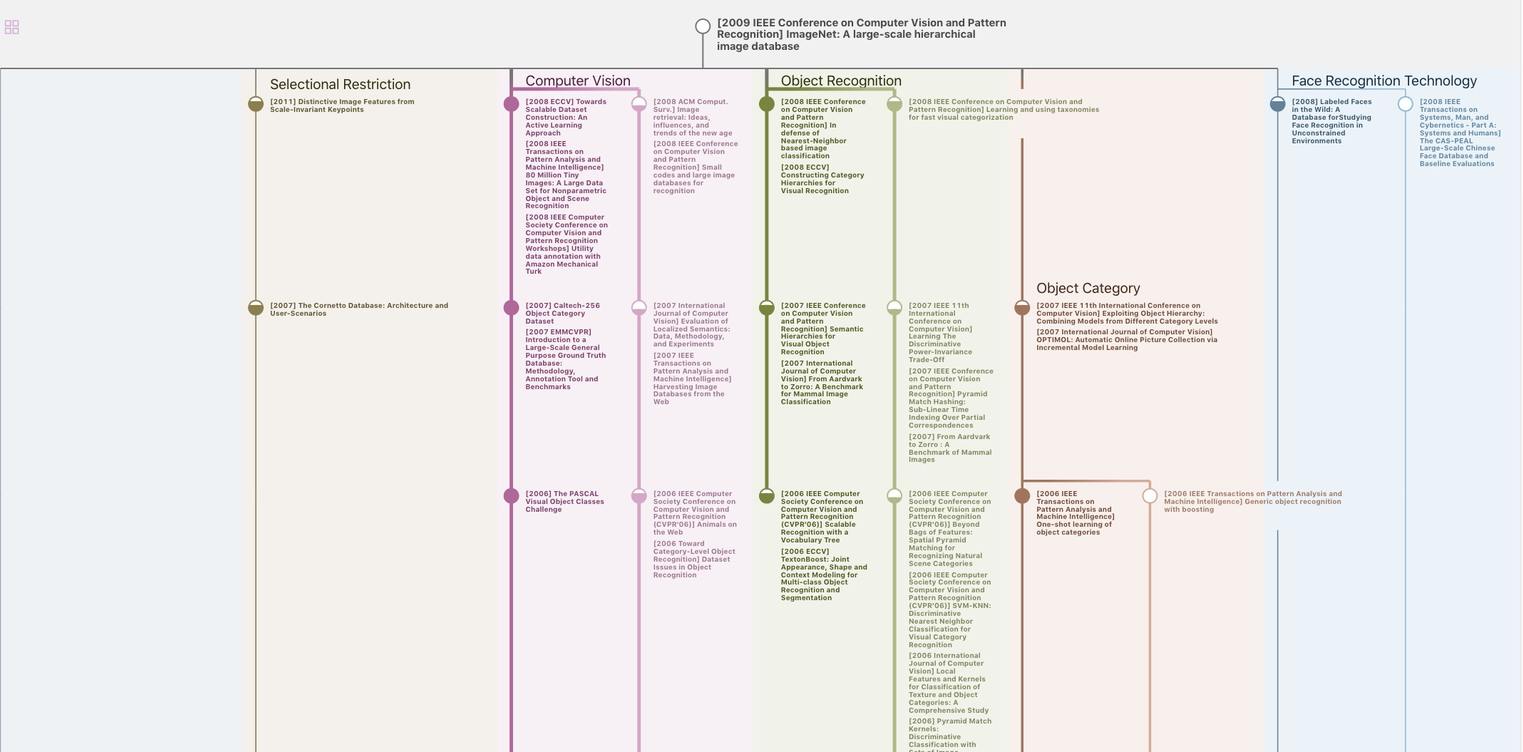
生成溯源树,研究论文发展脉络
Chat Paper
正在生成论文摘要