Learning-based Energy Efficiency Optimization in Cell-free Massive MIMO
IEEE CONFERENCE ON GLOBAL COMMUNICATIONS, GLOBECOM(2023)
摘要
Cell-free massive multiple-input multiple-output (MIMO) deploys a large number of distributed access points (APs) without cell edges, offering seamless connectivity with significantly increased spectral efficiency and system capacity, but suffering degraded energy efficiency. In this paper, we develop a green energy scheme by simultaneously optimizing power allocation and AP selection. We formulate it as a non-convex mixed-integer nonlinear programming problem (MINLP), which is NP-hard. To address this challenging problem, we propose a learning-based algorithm that embeds non-convex optimization into contemporary deep reinforcement learning (DRL), referred to as optimization-embedded soft actor-critic with graph transformer networks (OSAC-G). OSAC-G enjoys the benefits of directly online inferring solutions for the non-convex problem with a much lower computational complexity compared to conventional non-convex optimization. Simulation results demonstrate that the green energy scheme significantly decreases energy consumption compared to the existing ones.
更多查看译文
关键词
Energy Efficiency,Massive Multiple-input Multiple-output,Energy Consumption,Deep Learning,Renewable Energy,Low Complexity,Capacity Of System,Non-convex Problem,Deep Reinforcement Learning,Non-convex Optimization,Optimal Power Allocation,Mixed-integer Nonlinear Programming Problem,Power Consumption,Attention Mechanism,Trainable Parameters,Actor Network,Penalty Function,Reward Function,Node Features,Channel Coefficients,User Equipment,Backhaul Links,Deep Reinforcement Learning Algorithm,Heterogeneous Graph,Pilot Sequences,Minimum Mean Square Error Estimator,Average Power Consumption,Quality Of Service Requirements,Critic Network,System Parameter Variations
AI 理解论文
溯源树
样例
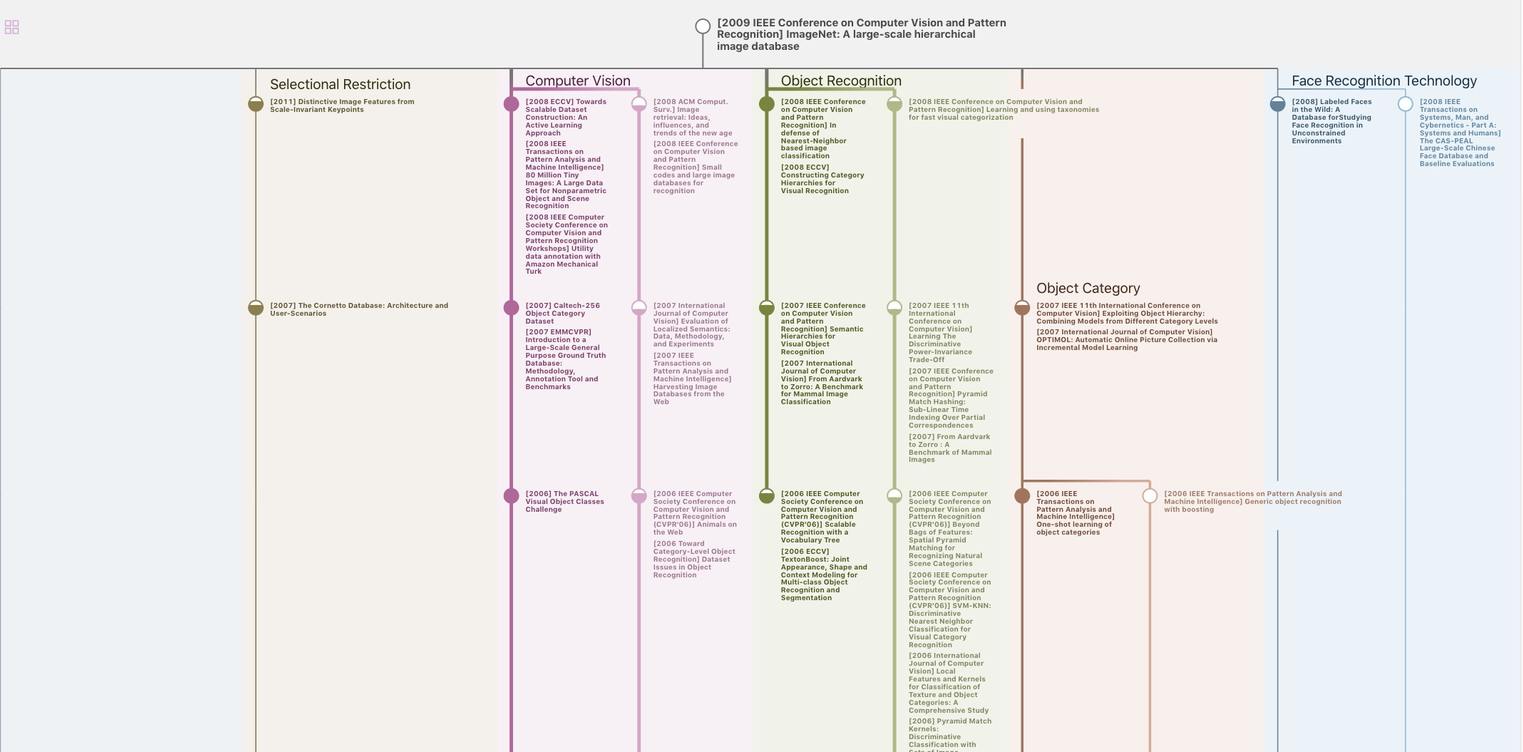
生成溯源树,研究论文发展脉络
Chat Paper
正在生成论文摘要