Rateless Deep Graph Joint Source Channel Coding for Holographic-Type Communication.
Global Communications Conference(2023)
摘要
A key challenge in holographic-type communication is transmitting point cloud signals to users across diverse channel quality and bandwidths. Digital based point cloud coding efficiently reduces point cloud traffic. In contrast, the quantization and entropy coding in digital-based schemes causes quality degradation owing to channel quality fluctuations and diversity. This study proposes a novel scheme for point cloud delivery over wireless channels. The proposed scheme consists of a graph auto-encoder (GAE) architecture to compress the point cloud into coded symbols and restore the point cloud from the received symbols. The proposed scheme addresses the quality degradation due to channel quality fluctuations and bandwidth diversity via, the following two steps. First, the coded symbols are directly mapped onto transmission symbols, analog modulation, to ensure that the point cloud quality follows the instantaneous channel quality of each user. Second, a non-uniform dropout is introduced to realize a rateless property in the GAE architecture for gradually improving the point cloud quality according to the available bandwidth. Evaluation results demonstrate that the proposed GAE architecture can yield better point cloud quality than digital-based and analog-based schemes, even when users have varying available bandwidths.
更多查看译文
关键词
Forward Error Correction,Joint Source-channel Coding,Point Cloud,Quality Degradation,Channel Bandwidth,Channel Quality,Autoencoder Architecture,User Quality,Entropy Coding,Decoding,Training Phase,Additive Noise,Deep Convolutional Neural Network,Multiple Users,3D Coordinates,3D Point,Vertices,Graph Convolutional Network,Reconstruction Quality,Bitstream,Chamfer Distance,Point Cloud Reconstruction,Graph Signal,Graph Convolution,Random Feature,Modulation Symbols,Graph Neural Networks,Quadrature Phase Shift Keying,Multicast,Binary Phase Shift Keying
AI 理解论文
溯源树
样例
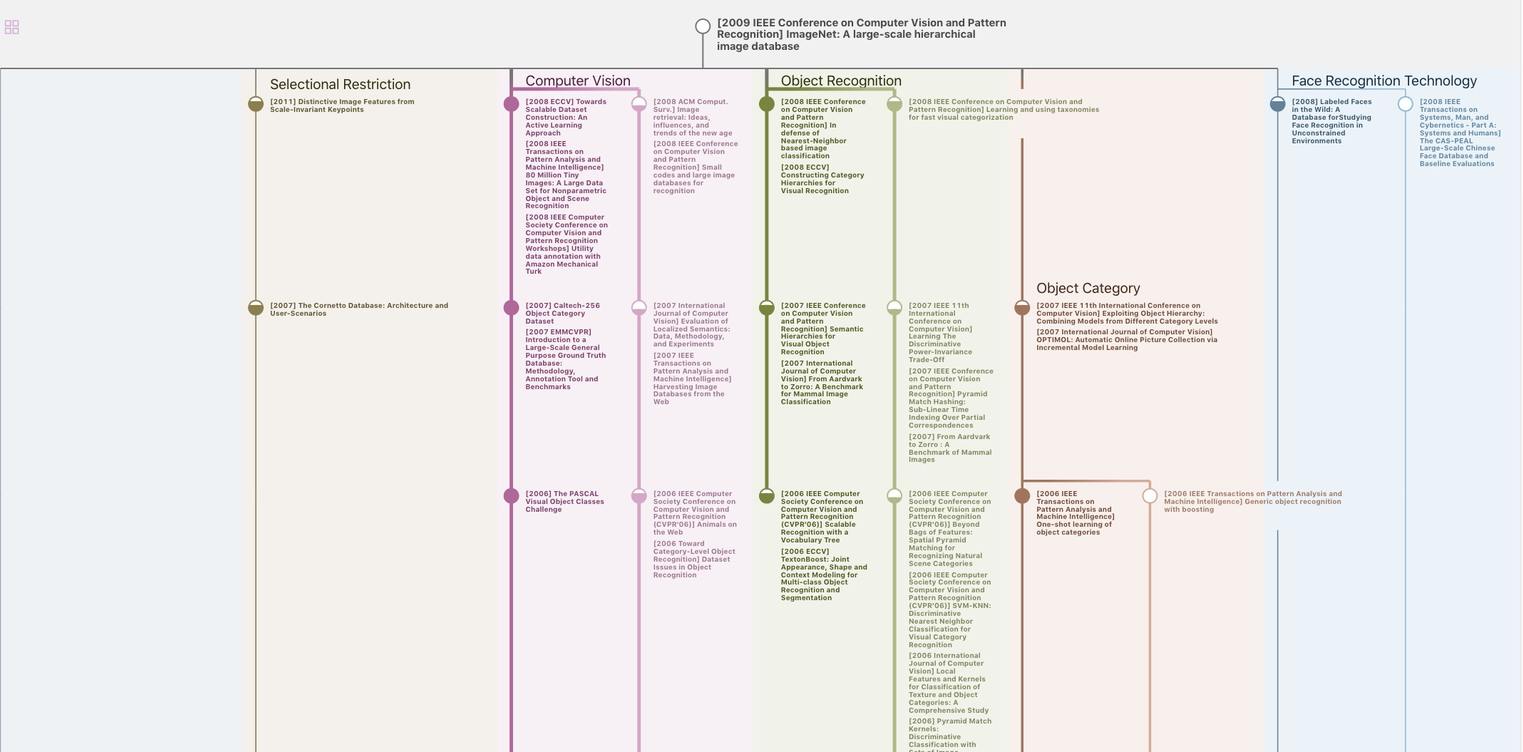
生成溯源树,研究论文发展脉络
Chat Paper
正在生成论文摘要