Predicting O-GlcNAcylation Sites in Mammalian Proteins with Transformers and RNNs Trained with a New Loss Function
CoRR(2024)
摘要
Glycosylation, a protein modification, has multiple essential functional and
structural roles. O-GlcNAcylation, a subtype of glycosylation, has the
potential to be an important target for therapeutics, but methods to reliably
predict O-GlcNAcylation sites had not been available until 2023; a 2021 review
correctly noted that published models were insufficient and failed to
generalize. Moreover, many are no longer usable. In 2023, a considerably better
RNN model with an F_1 score of 36.17
was published. This article first sought to improve these metrics using
transformer encoders. While transformers displayed high performance on this
dataset, their performance was inferior to that of the previously published
RNN. We then created a new loss function, which we call the weighted focal
differentiable MCC, to improve the performance of classification models. RNN
models trained with this new function display superior performance to models
trained using the weighted cross-entropy loss; this new function can also be
used to fine-tune trained models. A two-cell RNN trained with this loss
achieves state-of-the-art performance in O-GlcNAcylation site prediction with
an F_1 score of 38.82
更多查看译文
AI 理解论文
溯源树
样例
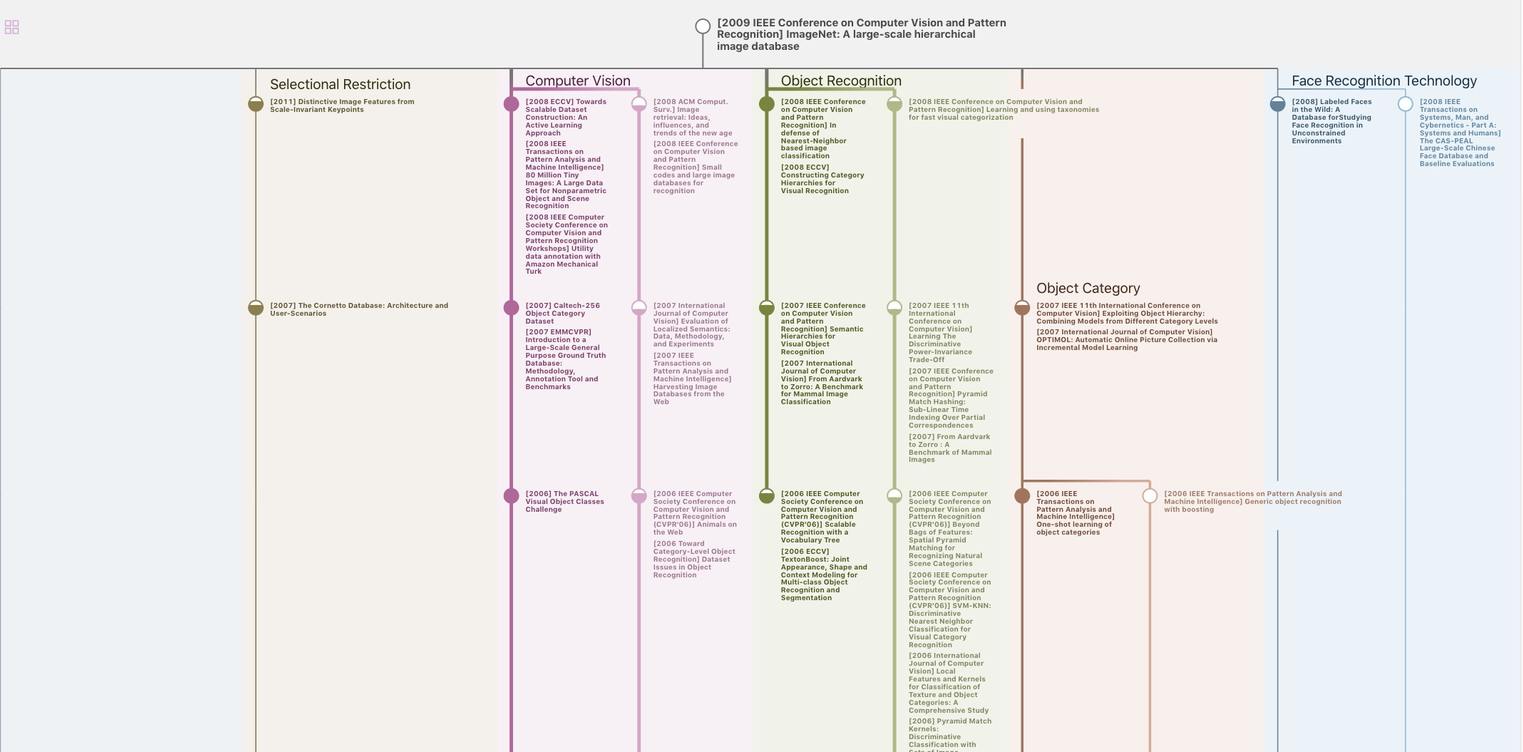
生成溯源树,研究论文发展脉络
Chat Paper
正在生成论文摘要