An Effective Mixture-Of-Experts Approach For Code-Switching Speech Recognition Leveraging Encoder Disentanglement
ICASSP 2024 - 2024 IEEE International Conference on Acoustics, Speech and Signal Processing (ICASSP)(2024)
摘要
With the massive developments of end-to-end (E2E) neural networks, recent
years have witnessed unprecedented breakthroughs in automatic speech
recognition (ASR). However, the codeswitching phenomenon remains a major
obstacle that hinders ASR from perfection, as the lack of labeled data and the
variations between languages often lead to degradation of ASR performance. In
this paper, we focus exclusively on improving the acoustic encoder of E2E ASR
to tackle the challenge caused by the codeswitching phenomenon. Our main
contributions are threefold: First, we introduce a novel disentanglement loss
to enable the lower-layer of the encoder to capture inter-lingual acoustic
information while mitigating linguistic confusion at the higher-layer of the
encoder. Second, through comprehensive experiments, we verify that our proposed
method outperforms the prior-art methods using pretrained dual-encoders,
meanwhile having access only to the codeswitching corpus and consuming half of
the parameterization. Third, the apparent differentiation of the encoders'
output features also corroborates the complementarity between the
disentanglement loss and the mixture-of-experts (MoE) architecture.
更多查看译文
关键词
Automatic speech recognition,code-switching,mixture-of-experts,disentanglement loss
AI 理解论文
溯源树
样例
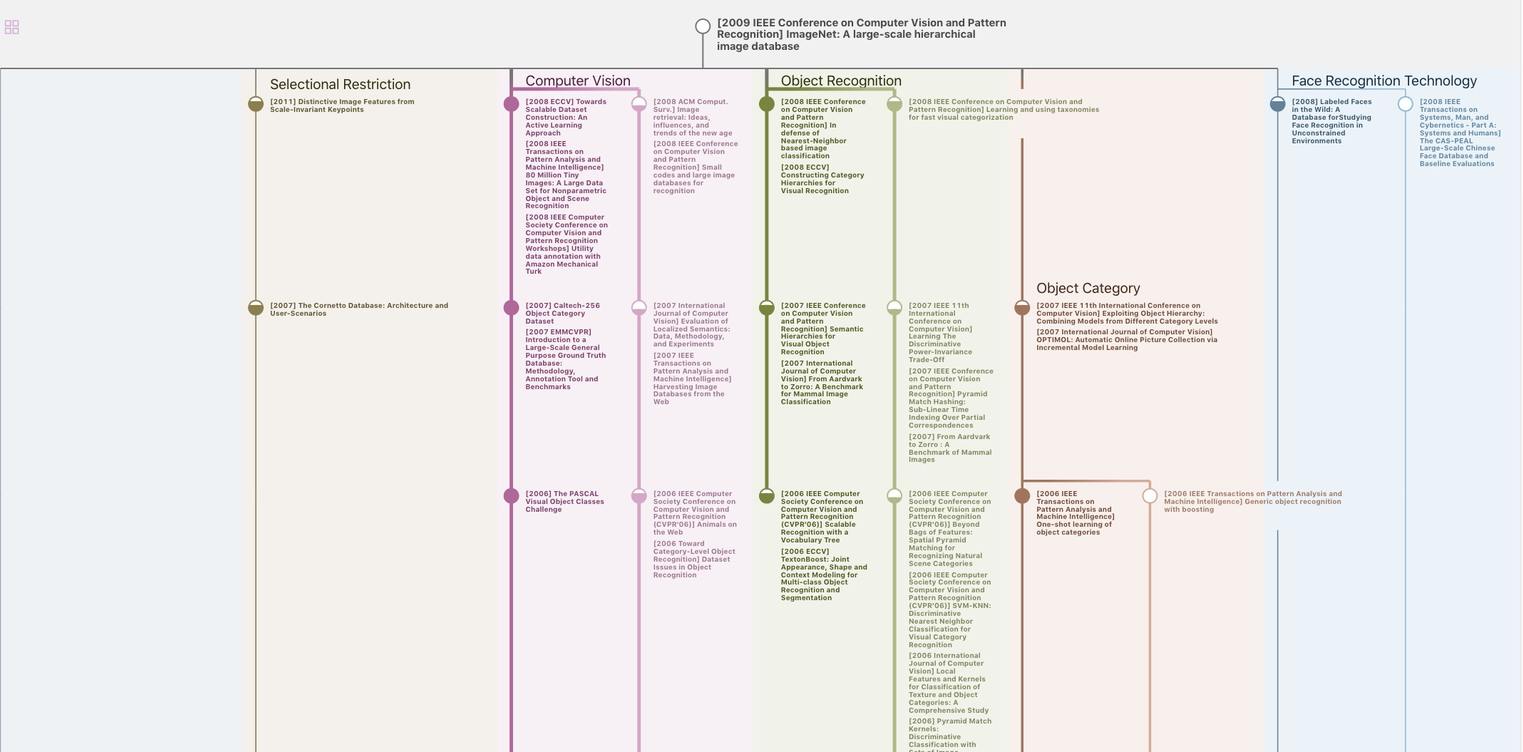
生成溯源树,研究论文发展脉络
Chat Paper
正在生成论文摘要