Neural Video Compression with Feature Modulation
2024 IEEE/CVF Conference on Computer Vision and Pattern Recognition (CVPR)(2024)
摘要
The emerging conditional coding-based neural video codec (NVC) showssuperiority over commonly-used residual coding-based codec and the latest NVCalready claims to outperform the best traditional codec. However, there stillexist critical problems blocking the practicality of NVC. In this paper, wepropose a powerful conditional coding-based NVC that solves two criticalproblems via feature modulation. The first is how to support a wide qualityrange in a single model. Previous NVC with this capability only supports about3.8 dB PSNR range on average. To tackle this limitation, we modulate the latentfeature of the current frame via the learnable quantization scaler. During thetraining, we specially design the uniform quantization parameter samplingmechanism to improve the harmonization of encoding and quantization. Thisresults in a better learning of the quantization scaler and helps our NVCsupport about 11.4 dB PSNR range. The second is how to make NVC still workunder a long prediction chain. We expose that the previous SOTA NVC has anobvious quality degradation problem when using a large intra-period setting. Tothis end, we propose modulating the temporal feature with a periodicallyrefreshing mechanism to boost the quality. problems, we also design a single model that can support both RGB and YUVcolorspaces. Notably, under single intra-frame setting, our codec can achieve29.7% bitrate saving over previous SOTA NVC with 16% MACs reduction. Ourcodec serves as a notable landmark in the journey of NVC evolution. The codesare at https://github.com/microsoft/DCVC.
更多查看译文
关键词
Neural video compression
AI 理解论文
溯源树
样例
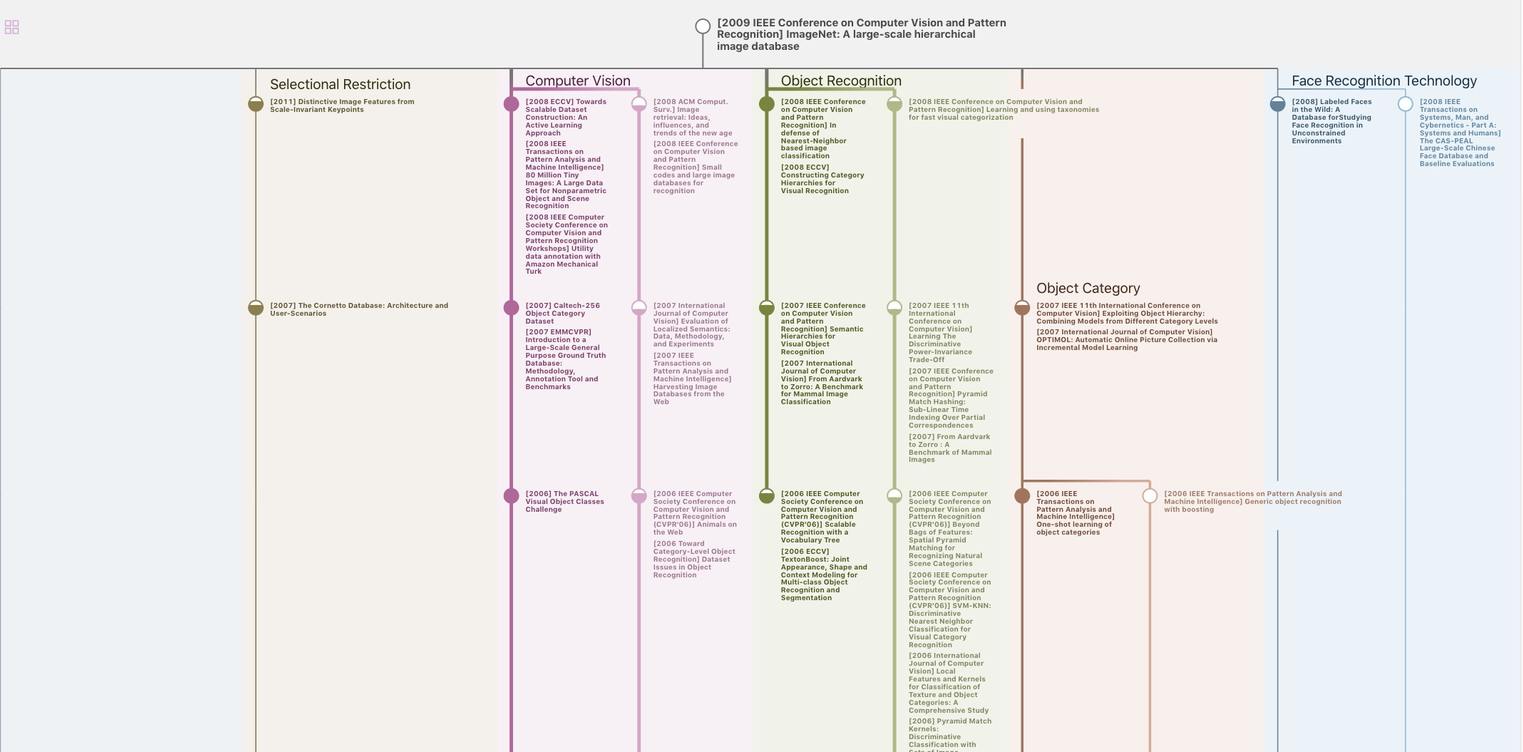
生成溯源树,研究论文发展脉络
Chat Paper
正在生成论文摘要