Optimization of foreground moment deprojection for semi-blind CMB polarization reconstruction
arxiv(2024)
摘要
Upcoming Cosmic Microwave Background (CMB) experiments, aimed at measuring
primordial CMB B-modes, require exquisite control of Galactic foreground
contamination. Minimum-variance techniques, like the Needlet Internal Linear
Combination (NILC), have proven effective in reconstructing the CMB
polarization signal and mitigating foregrounds across diverse sky models
without suffering from mismodelling errors. Still, residual contamination may
bias the recovered CMB polarization at large angular scales when confronted
with the most complex foreground scenarios. By adding constraints to NILC to
deproject moments of the Galactic emission, the Constrained Moment ILC (cMILC)
method has proven to enhance foreground subtraction, albeit with an associated
increase in overall noise variance. Faced with this trade-off between
foreground bias reduction and overall variance minimization, there is still no
recipe on which moments to deproject and which are better suited for blind
variance minimization. To address this, we introduce the optimized cMILC
(ocMILC) pipeline, which performs full optimization of the required number and
set of foreground moments to deproject, pivot parameter values, and
deprojection coefficients across the sky and angular scales, depending on the
actual sky complexity, available frequency coverage, and experiment
sensitivity. The optimal number of deprojected moments, before paying
significant noise penalty, is determined through a data diagnosis inspired by
the Generalized NILC (GNILC) method. Validated on B-mode simulations of the
PICO space mission concept with four challenging foreground models, ocMILC
exhibits lower foreground contamination compared to NILC and cMILC at all
angular scales, with limited noise penalty. This multi-layer optimization
enables the ocMILC pipeline to achieve unbiased posteriors of the
tensor-to-scalar ratio, regardless of foreground complexity.
更多查看译文
AI 理解论文
溯源树
样例
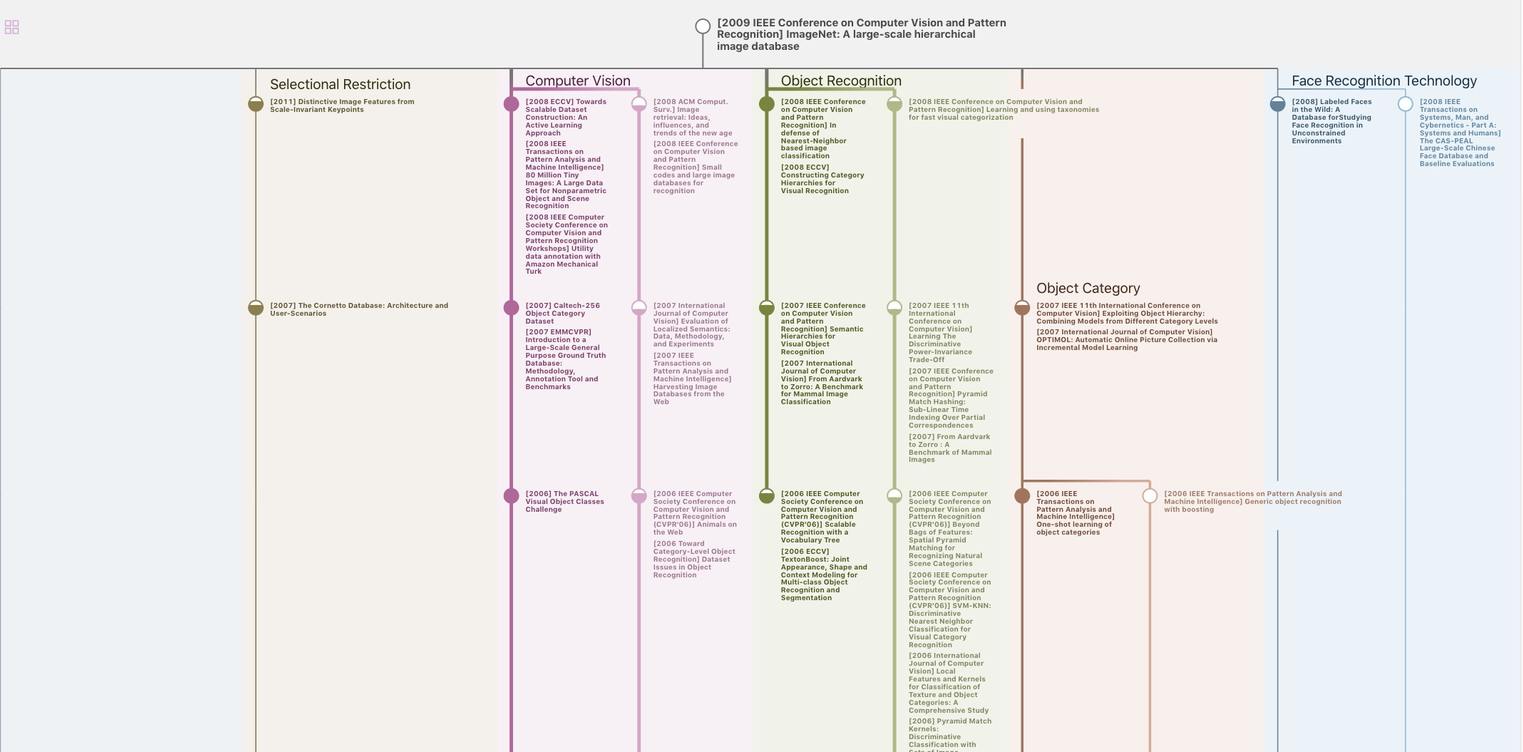
生成溯源树,研究论文发展脉络
Chat Paper
正在生成论文摘要