Autonomous MEC Selection in Federated Next-Gen Networks via Deep Reinforcement Learning
IEEE CONFERENCE ON GLOBAL COMMUNICATIONS, GLOBECOM(2023)
摘要
The increasing demand for cellular networks has prompted vendors and service providers to concentrate on establishing a more autonomous network infrastructure. Among numerous emerging paradigms, network service federation has gained prominence. This model facilitates collaboration between Virtual Network Operators (VNO) and Infrastructure Providers in a Multi-Domain Network, enabling resource sharing, enhanced quality of service, and expanded coverage areas. Nevertheless, the varying service level agreements (SLA) among network operators can influence several aspects for customers attempting to offload a task to a network, particularly in roaming scenarios. Factors such as cost and service performance become crucial considerations, potentially creating complexities for end users. Herein, to automate this process, a Deep Reinforcement Learning (DRL) model was devised for efficient MEC selection. The DRL model introduced on the Multi-Access Edge Computing (MEC) finds the optimal policy for the user offloading the task. Through simulation, the DRL model was able to reduce cost by 38.84% and had an accuracy of 97.6% during training.
更多查看译文
关键词
B5G/6G Networks,Federation,DRL,SLA,VNO,Next-G Networks,MEC
AI 理解论文
溯源树
样例
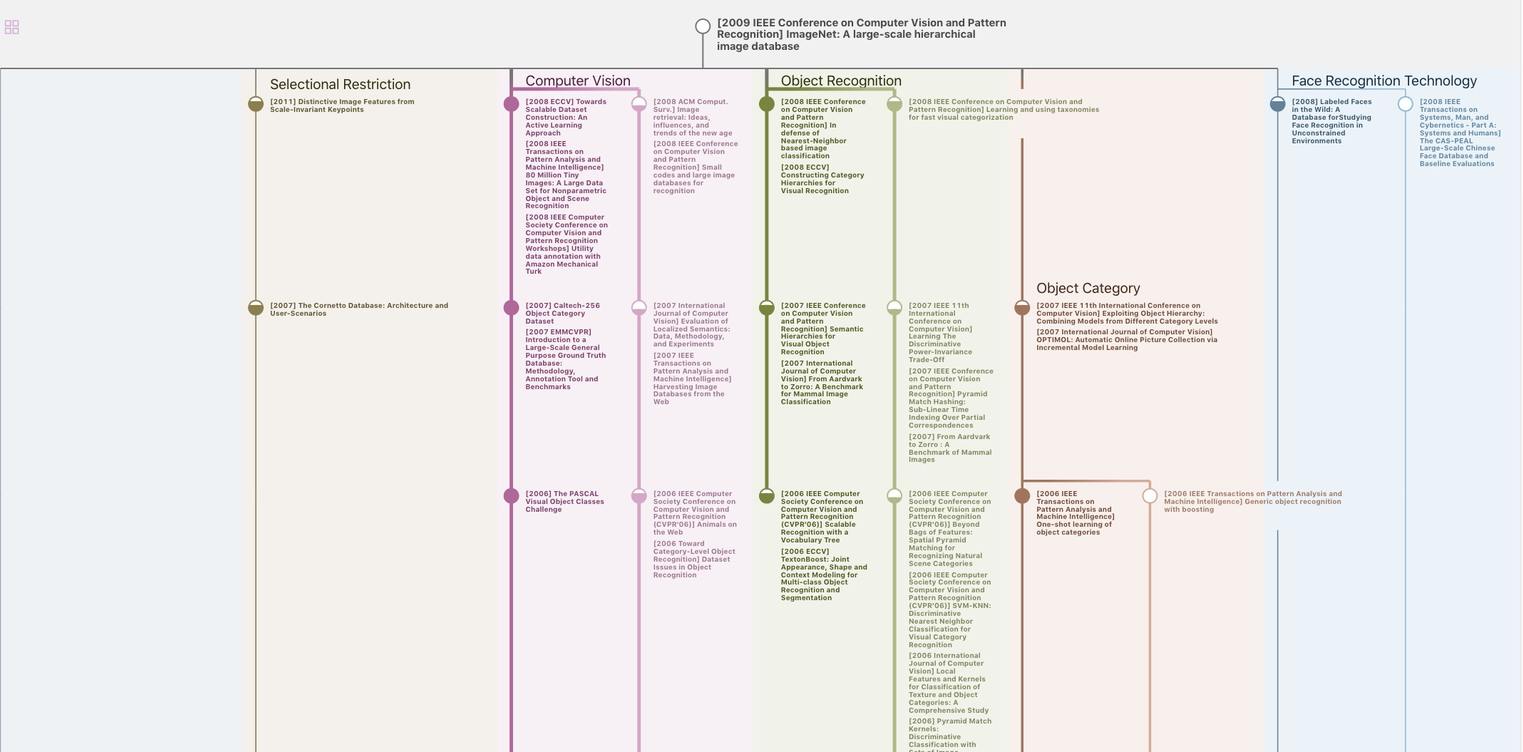
生成溯源树,研究论文发展脉络
Chat Paper
正在生成论文摘要