A Lightweight Few-Shot Attack Detection Scheme for Industrial Cognitive Radio Networks
IEEE CONFERENCE ON GLOBAL COMMUNICATIONS, GLOBECOM(2023)
摘要
Industrial cognitive radio networks (ICRNs) have been a promising spectrum-sharing solution for massive resource-constrained wireless devices in the industrial Internet of things (IIoT). However, ICRNs are raising new opportunities for malicious users, wherein the threat landscape is compounded with few-shot attacks due to the insufficiency of high-quality examples. In this paper, we propose a novel lightweight intrusion detection system focusing on few-shot attacks for ICRNs, called KDFSIDS. Specifically, we first develop a teacher-student model based hierarchical intrusion detection framework for ICRNs. Second, we design a convolutional neural network-based intrusion detection model as the fundamental model for identifying few-shot attacks. Third, a knowledge distillation strategy is crafted to obtain a lightweight but sufficiently accurate model for KDFSIDS. Extensive experiments on three public datasets demonstrate the superiorities of our proposed scheme in detecting few-shot attacks for ICRNs, in terms of both effectiveness and accuracy.
更多查看译文
关键词
Few-shot attack detection,knowledge distillation,industrial cognitive radio networks,IIoT
AI 理解论文
溯源树
样例
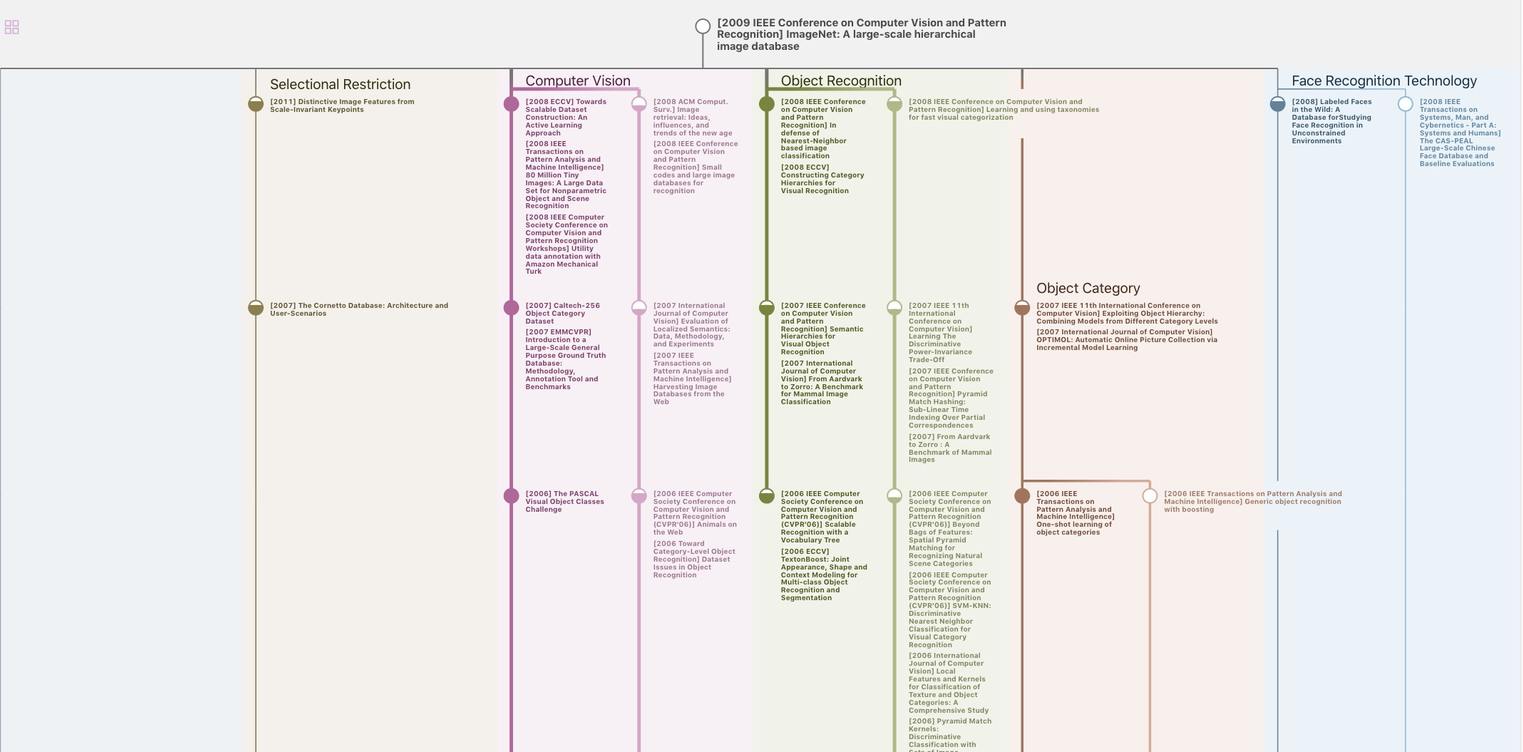
生成溯源树,研究论文发展脉络
Chat Paper
正在生成论文摘要