FLDetect: An API-Based Ransomware Detection Using Federated Learning
IEEE CONFERENCE ON GLOBAL COMMUNICATIONS, GLOBECOM(2023)
摘要
Ransomware, a malicious piece of software responsible for several high-profile attacks in recent years, poses a significant threat to organizations of all sizes. Such attacks can cause significant operational and financial harm, including system interruptions and compromises of system integrity. By developing the ability to detect and prevent ransomware attacks, we can contribute to the creation of a more secure and safe digital ecosystem. In this research, we propose FLDetect, a unique Federated Learning (FL)-based method for identifying ransomware on Windows machines. Windows machines, integral to Internet of Things (IoT) networks, can act as brokers to other sensor nodes, rendering them susceptible to such attacks. Our approach utilizes distributed computing to train a Machine Learning (ML) model using data from various devices without relying on centralized data storage. The API-call-pattern-based detection method is the preferred approach for detecting ransomware in this paper. We made use of an open-source dataset, known as ransomwaredataset2016, for a comparable objective. The global model's accuracy was 93.1% after we trained it with twenty different devices. Our results demonstrate that our method is effective in identifying ransomware while maintaining the privacy and security of the training data by utilizing FL.
更多查看译文
关键词
Federated Learning (FL),Ransomware Detection,API,Windows Security
AI 理解论文
溯源树
样例
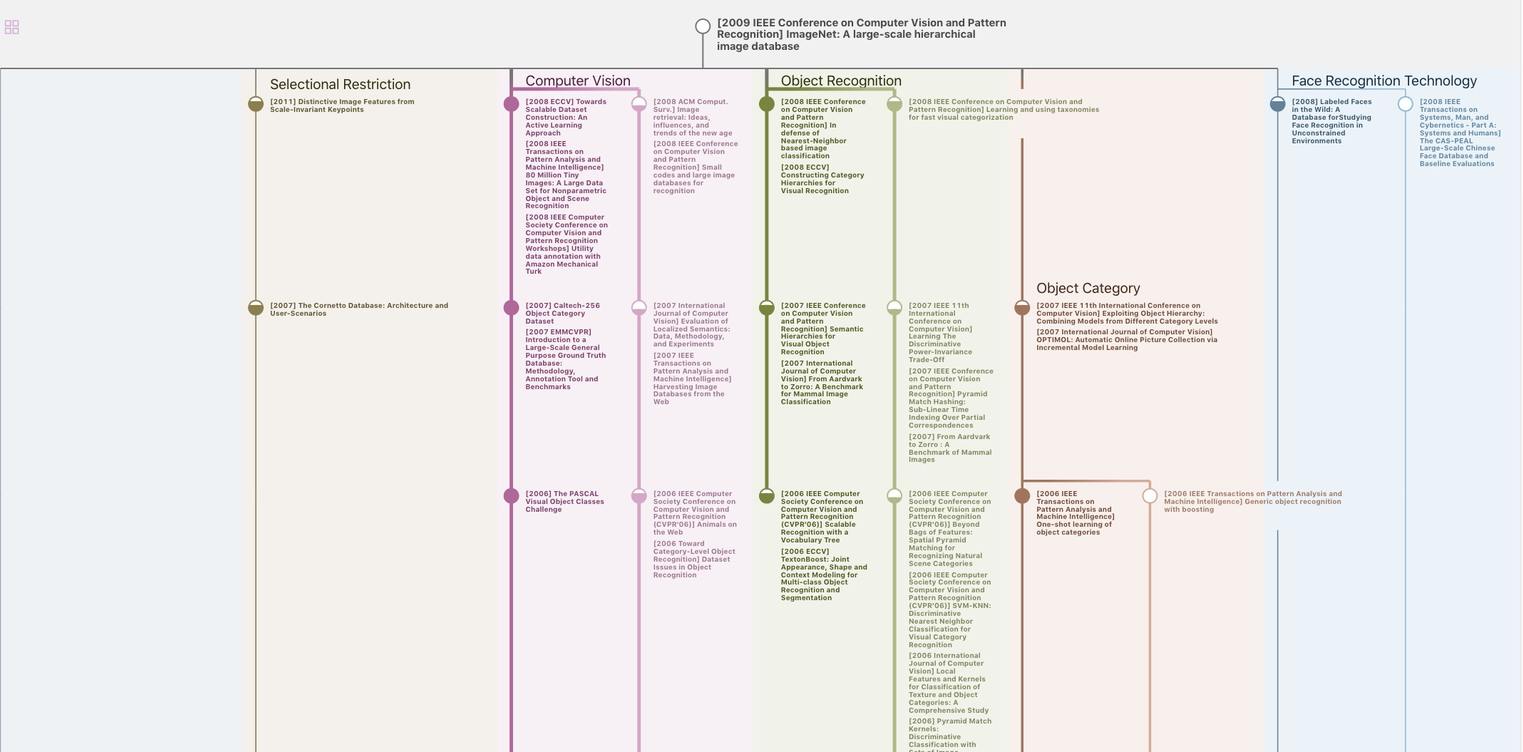
生成溯源树,研究论文发展脉络
Chat Paper
正在生成论文摘要