Self-paced and Reweighting PU Learning for Imbalanced Malicious Traffic Detection
IEEE CONFERENCE ON GLOBAL COMMUNICATIONS, GLOBECOM(2023)
摘要
With the proliferation of information and rapid network development, network attacks and threats are on the rise, and identifying malicious traffic is crucial for network security. However, the distribution of malicious and benign traffic in real-world networks is unbalanced, and the identification of malicious traffic in a real-world environment with a large amount of unbalanced and less known labelled malicious traffic is still a pressing problem. To tackle this issue, the paper presents a self-paced and reweighting Positive-Unlabeled learning algorithm (SRPU) for detecting imbalanced distribution traffic. This approach includes a self-paced training strategy that flexibly identifies assured positive/negative instances while taking the regret data loss into account, and a double-loss reweighting strategy to classify imbalanced malevolent traffic. We present the performance of SRPU by performing real-world experiments on three widely available datasets. These datasets include both common network attacks and APT attacks. The experimental results show that SRPU achieves the highest F1 and MCC scores at different label frequencies. It significantly outperforms existing methods.
更多查看译文
关键词
Imbalanced malicious traffic detection,PU learning,Class imbalance,Self-paced learning,Reweighting
AI 理解论文
溯源树
样例
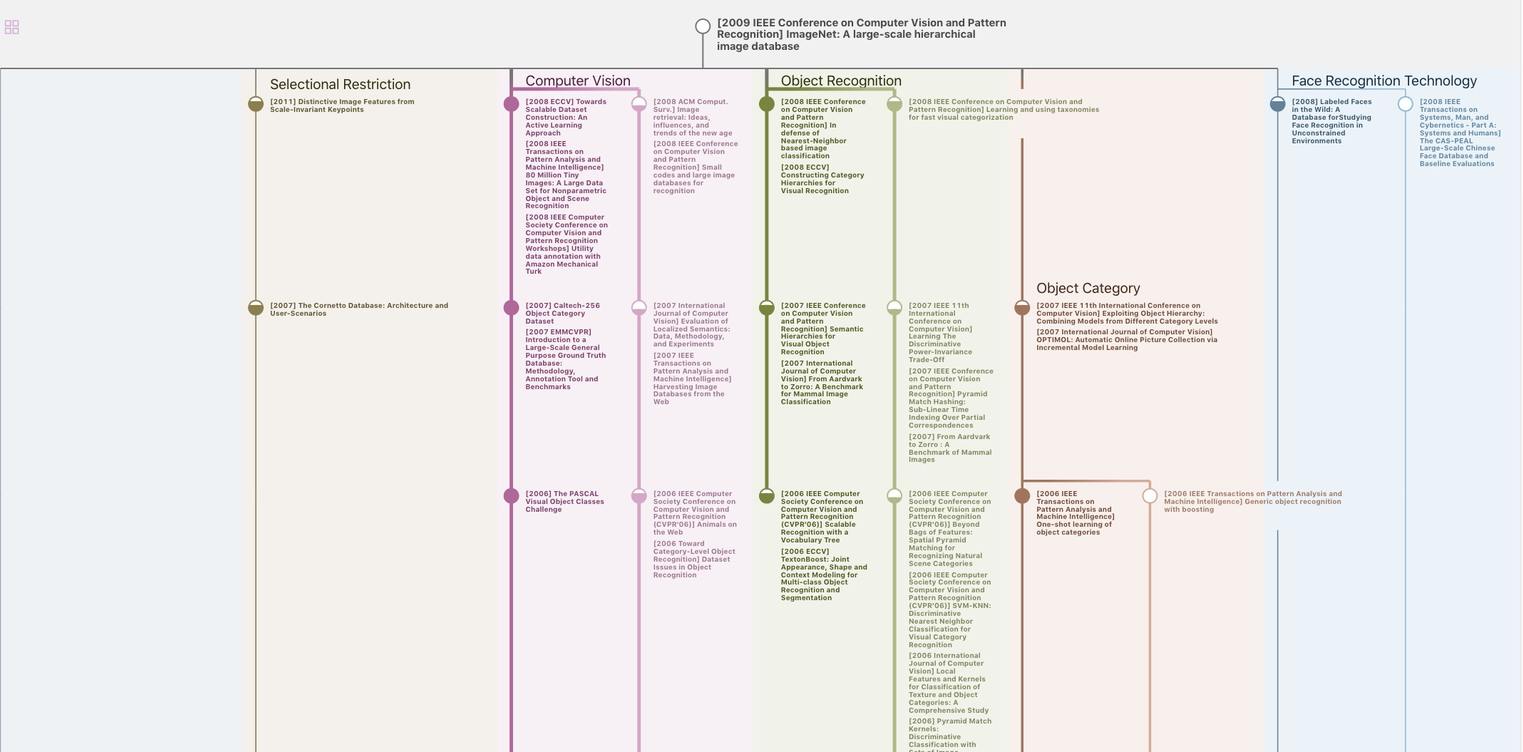
生成溯源树,研究论文发展脉络
Chat Paper
正在生成论文摘要