An automated quality assurance system with deep learning for small cube-ball phantom localization in noisy megavoltage images
Journal of the Korean Physical Society(2024)
摘要
To enhance efficiency and minimize errors, we automated the quality assurance (QA) process in radiation oncology, specifically laser localization. Additionally, we explored the use of a convolutional neural network (CNN) to enhance the detection of small cube-ball phantoms in noisy images. Laser localizations were measured manually on the acquired images. To automate the QA workflow, we developed a Linux server equipped with database and web servers. Digital Imaging and Communications in Medicine (DICOM) files were retrieved 40 times for 10 linear accelerators (LINACs). The center of the cube-ball phantoms was estimated through Gaussian fitting. We applied CNN using 6,968 stored results to improve the estimation performance in noisy megavoltage (MV) images. Subsequently, both analysis time and accuracy were compared. Our hospital has been employing the automated QA system since 2018, notably reducing the time for laser localization from 30 min to just 1 min. The average and standard deviation (SD) of inter-observer variability in the X- and Y-axes were 0.06 ± 0.01 mm and 0.05 ± 0.01 mm, respectively. Absolute differences between manual assessment and Gaussian fitting presented average and SD values of 0.40 ± 0.51 mm and 0.23 ± 0.24 mm, respectively. In contrast, absolute differences between manual assessment and CNN presented average and SD values of 0.12 ± 0.10 mm and 0.11 ± 0.09 mm, respectively. Overall, the automated QA system significantly hastened procedures in our large hospital and improved the estimation of the cube-ball phantom’s position in noisy images through deep learning.
更多查看译文
关键词
Radiation oncology,Automated QA system,Machine learning,Image processing,Medical physics
AI 理解论文
溯源树
样例
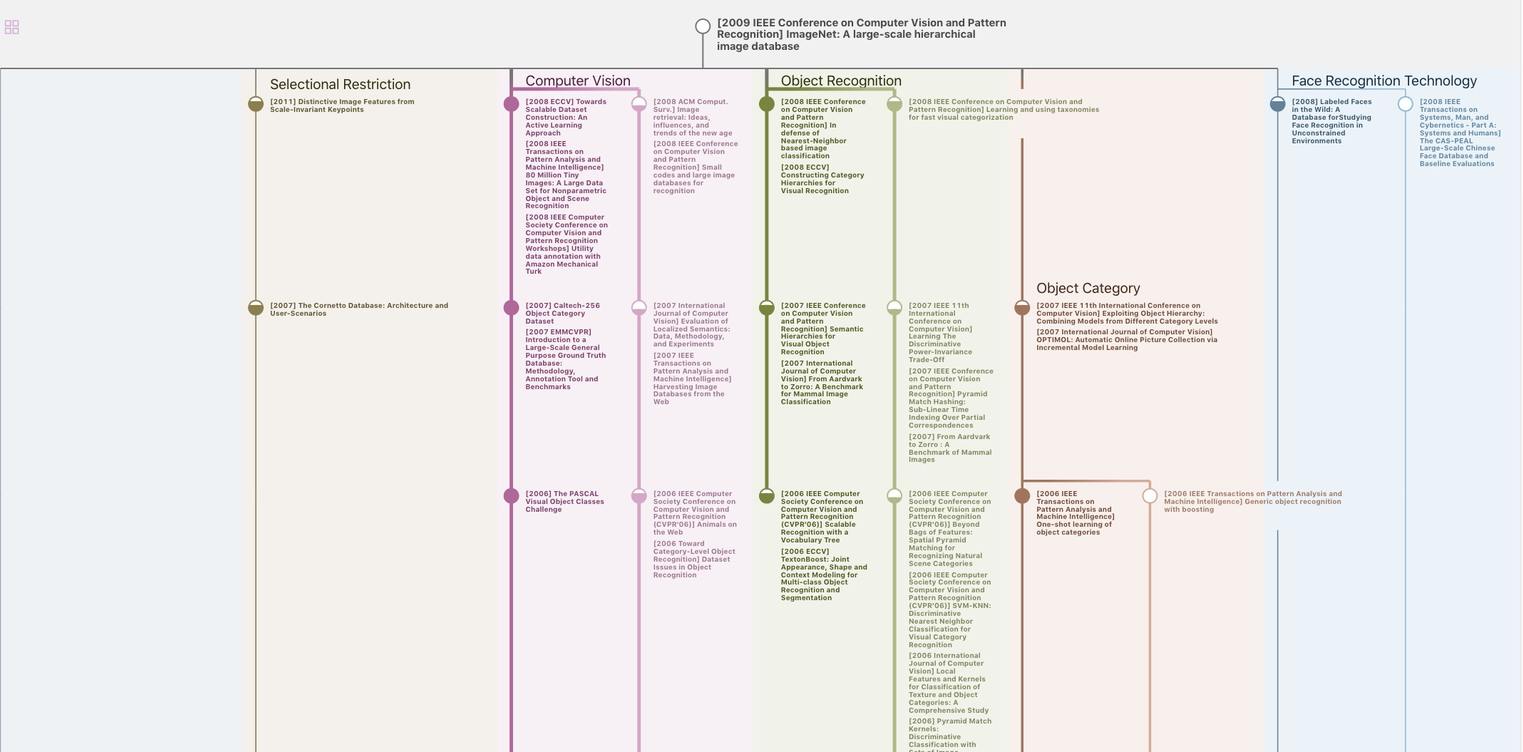
生成溯源树,研究论文发展脉络
Chat Paper
正在生成论文摘要