What postpones degree completion? Discovering key predictors of undergraduate degree completion through explainable artificial intelligence (XAI)
Journal of Marketing Analytics(2024)
摘要
The timing of degree completion for students taking post-secondary courses has been a constant source of angst for administrators wanting the best outcomes for their students. Most methods for predicting student degree completion extensions are completed by analog methods using human effort to analyze data. The majority of data analysis reporting of degree completion extension variables and impacts has, for decades, been done manually. Administrators primarily forecast the factors based on their expertise and intuition to evaluate implications and repercussions. The variables are large, varied, and situational to each individual and complex. We used machine learning (automated processes using predictive algorithms) to predict undergraduate extensions for at least 2 years beyond a standard 4 years to complete a bachelor's degree. The study builds a machine learning-based education understanding XAI model (ED-XAI) to examine students’ dependent and independent variables and accurately predict/explain degree extension. The study utilized Random Forest, Support Vector Machines, and Deep Learning Machine learning algorithms. XAI used Information Fusion, SHapley Additive exPlanations (SHAP), and Local Interpretable Model-Agnostic Explanations (LIME) models to explain the findings of the Machine Learning models. The ED-XAI model explained multiple scenarios and discovered variables influencing students’ degree completion linked to their status and funding source. The Random Forest model gave supreme predictive results with 89.1
更多查看译文
关键词
Explainable Artificial Intelligence (XAI),Higher Education,Prediction,Machine Learning,Predictive Analytics,Undergraduate Degree Completion,Educational Data Mining
AI 理解论文
溯源树
样例
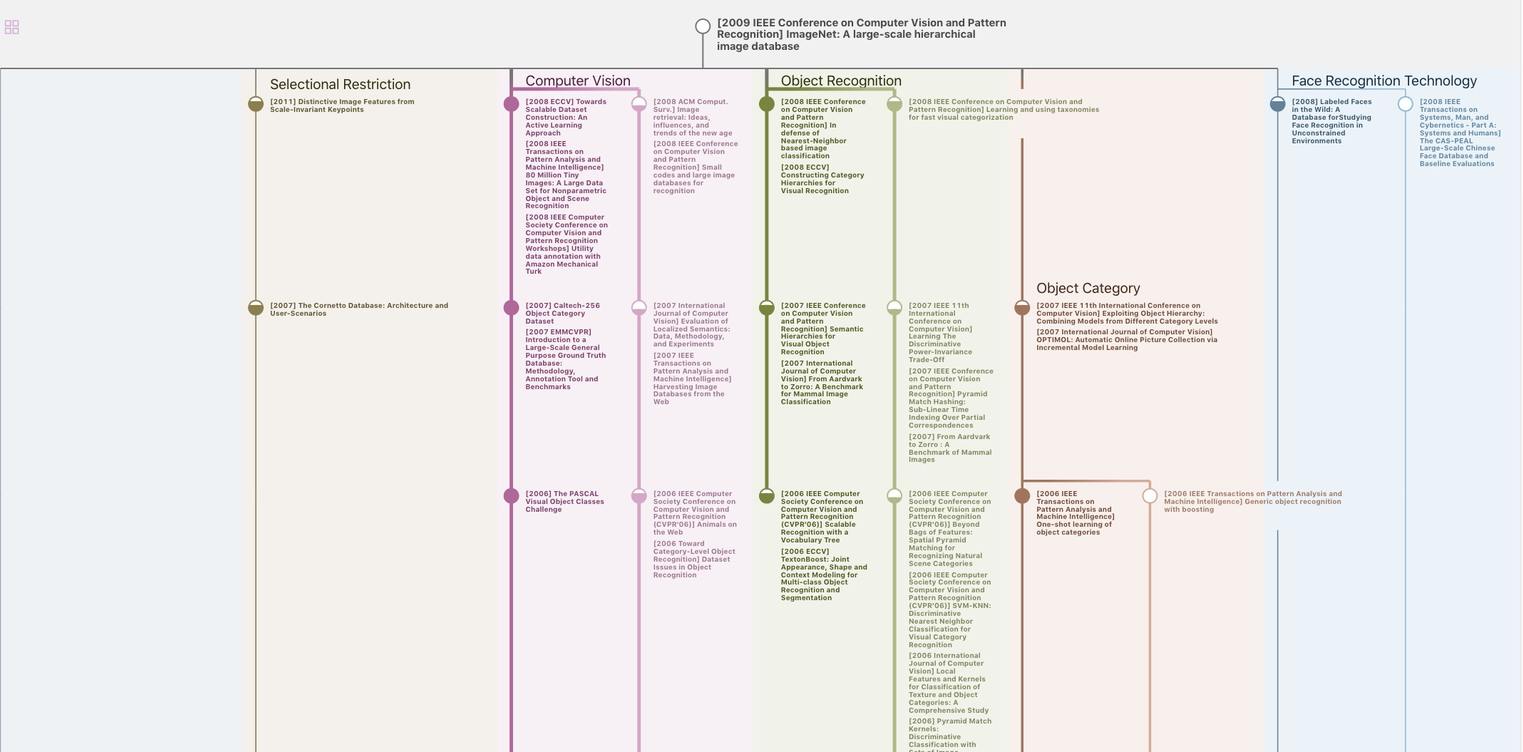
生成溯源树,研究论文发展脉络
Chat Paper
正在生成论文摘要