A data envelopment analysis model for opinion leaders’ identification in social networks
Computers & Industrial Engineering(2024)
摘要
Through Online Social Networks (OSNs) such as Instagram, X (Twitter), and Facebook, employing Opinion Leaders (OLs) is becoming integral to companies’ strategies for influencing the public. The graph-based methods are one of the most important approaches for finding OLs in OSNs. Social Network Analysis (SNA)-based OLs finding methods deal with a considerable amount of data due to using entire relationships between all of the users in a network, which makes the algorithms time-consuming. Our main goal is to introduce a new method of OLs discovery that works with fewer data and maintains or improves performance metrics. Consequently, a new application of the Data Envelopment Analysis (DEA) method is presented here for OLs identification in social media. Another contribution of this paper is introducing a new framework (OL-Finder Evaluator or OLFE) for validating the OLs’ detection algorithms under imbalanced datasets. DEA methods, when compared with SNA methods, have the advantage of being able to apply over non-graph-based datasets and to work with substantially smaller datasets. In contrast, SNA methods require transparent relationships between people. In this study, we compare both DEA (including CCR and BCC) and SNA measures (including “Betweenness (BC),” “Degree (DC),” “Page Rank (PRC),” “Closeness (CC),” and “Eigenvector (EC)” centralities) on a real Instagram network for OLs detection. Compared with SNA, our proposed method can identify OLs with considerably fewer data. Besides the advantages of DEA for time-saving, close competition exists between the DEA and the SNA methods. On average, DEA performs better in accuracy, precision, recall, and F1-score performance metrics.
更多查看译文
关键词
Data Envelopment Analysis (DEA),Opinion Leaders (OLs) Detection,Online Social Networks (OSNs),Influencer Marketing,Instagram
AI 理解论文
溯源树
样例
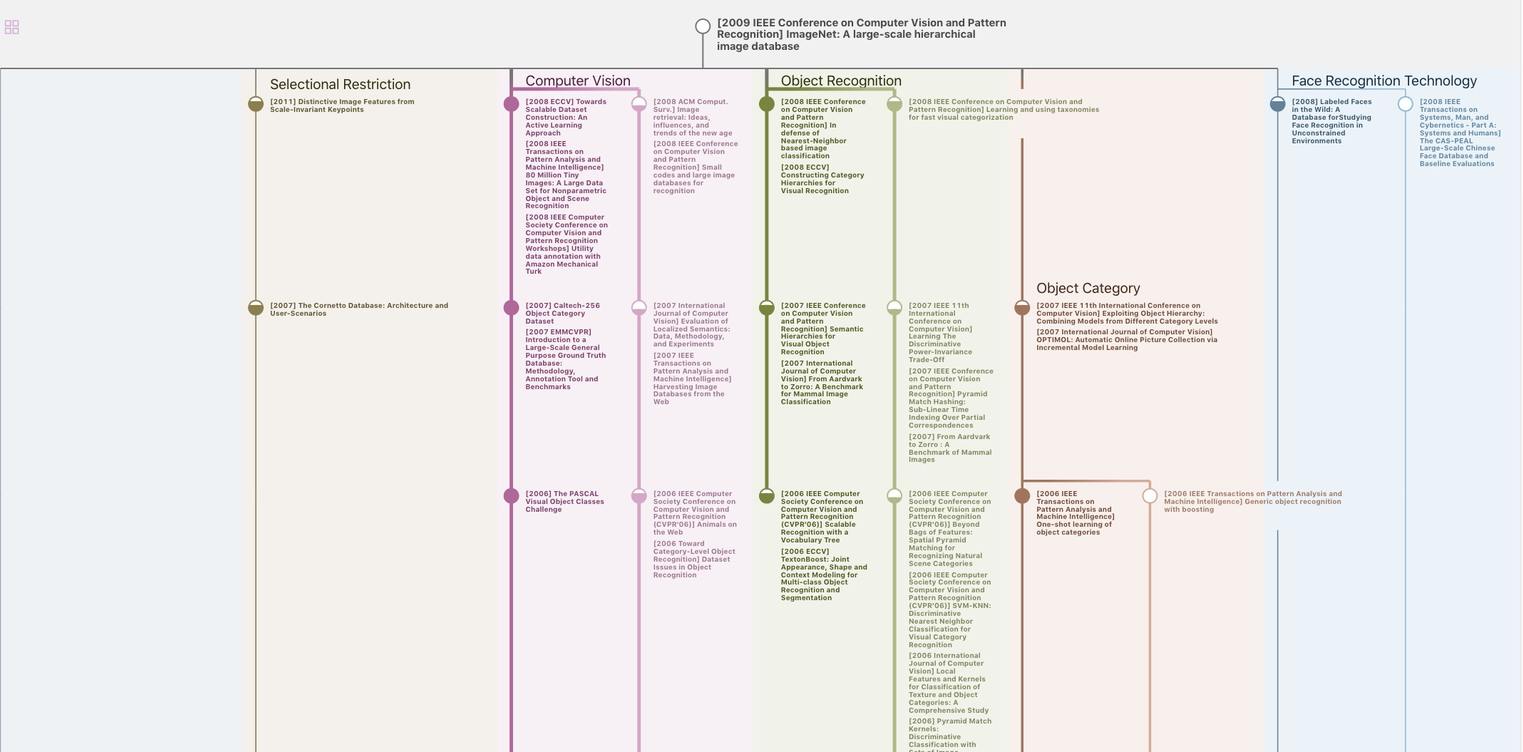
生成溯源树,研究论文发展脉络
Chat Paper
正在生成论文摘要