Learn More and Learn Usefully: Truncation Compensation Network for Semantic Segmentation of High-Resolution Remote Sensing Images
IEEE TRANSACTIONS ON GEOSCIENCE AND REMOTE SENSING(2024)
摘要
Semantic segmentation of high-resolution remote-sensing images (HR-RSIs) focuses on classifying each pixel of input images. Recent methods have incorporated a downscaled global image as supplementary input to alleviate global context loss from cropping. Nonetheless, these methods encounter two key challenges: diminished detail in features due to downsampling of the global auxiliary image (GAI) and noise from the same image that reduces the network's discriminability of useful and useless information. To overcome these challenges, we propose a truncation compensation network (TCNet) for HR-RSI semantic segmentation. TCNet features three pivotal modules: the guidance feature extraction module (GFM), the related-category semantic enhancement module (RSEM), and the global-local contextual cross-fusion module (CFM). GFM focuses on compensating for truncated features in the local image and minimizing noise to emphasize learning of useful information. RSEM enhances discernment of global semantic information by predicting spatial positions of related categories and establishing spatial mappings for each. CFM facilitates local image semantic segmentation with extensive contextual information by transferring information from global to local feature maps. Extensive testing on the ISPRS, BLU, and GID datasets confirms the superior efficiency of TCNet over other approaches.
更多查看译文
关键词
Semantic segmentation,Semantics,Remote sensing,Feature extraction,Aggregates,Transformers,Decoding,Context fusion,remote-sensing images,semantic enhancement,semantic segmentation,useful information
AI 理解论文
溯源树
样例
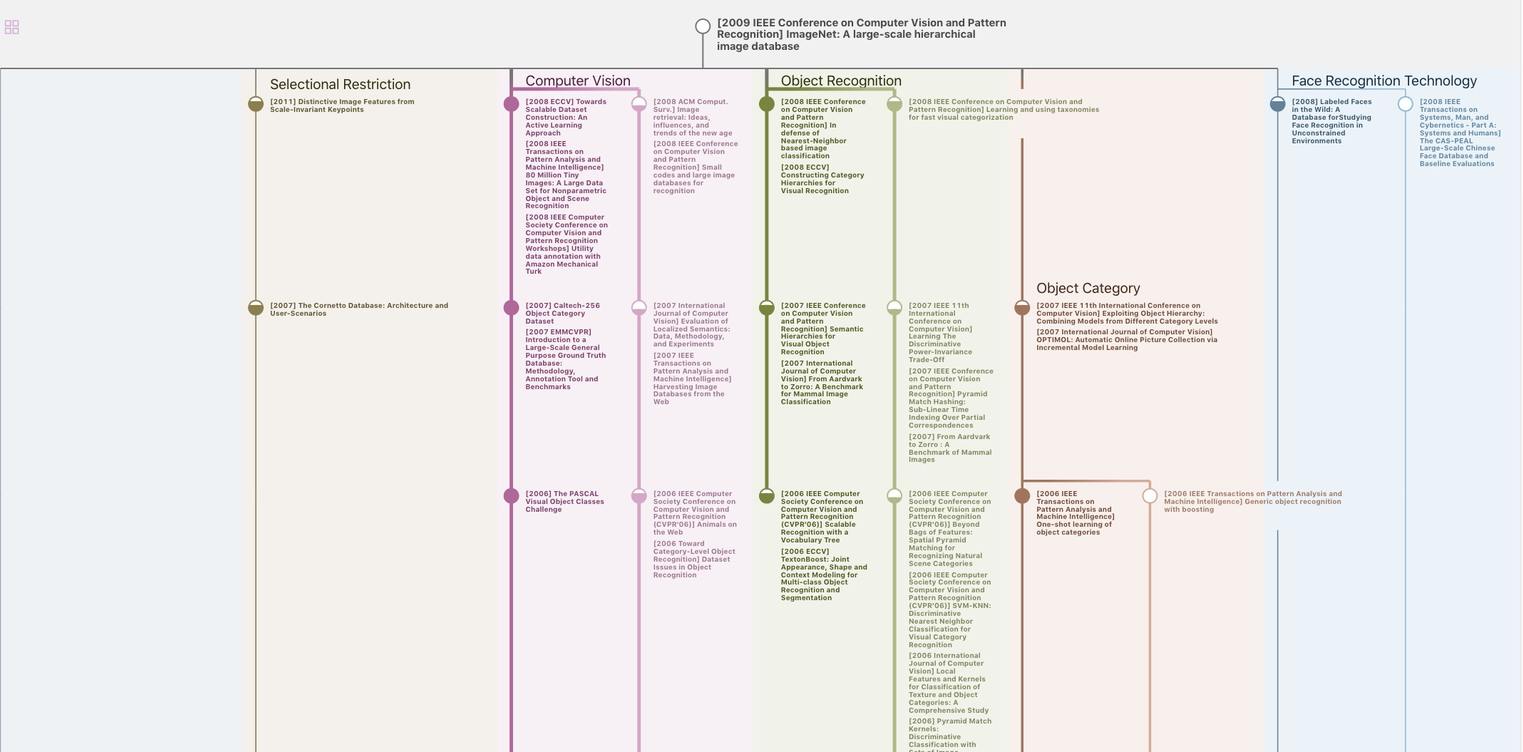
生成溯源树,研究论文发展脉络
Chat Paper
正在生成论文摘要