Learning to Stabilize: Comparative Analysis of Reinforcement Learning and Traditional Methods for Swirling Pendulum Control
2023 NINTH INDIAN CONTROL CONFERENCE, ICC(2023)
摘要
In this paper, we develop a reinforcement learning (RL) based controller capable of stabilizing the swirling pendulum, a novel under-actuated two degrees of freedom system with a number of underlying peculiarities such as non-planar inertial coupling, loss of relative degree, multiple stable and unstable equilibria, etc. These properties make the control and stabilization of the swirling pendulum challenging, especially at the unstable equilibrium points in the upper hemisphere. To the best of our knowledge, the stabilization of the Swirling Pendulum at the upper unstable equilibrium points has not yet been solved using linear, modern, or nonlinear control methods. We present a novel controller that is able to stabilize the swirling pendulum at each of these unstable equilibrium points using the Actor-Critic algorithm. We also present a comparative analysis between reinforcement learning (RL) and traditional control methods for the purpose of stabilizing the swirling pendulum at the unstable equilibrium point in the lower hemisphere. Our results demonstrate that the RL-based approach outperforms the conventional controllers, such as PID and Lead Compensator, while stabilizing the swirling pendulum at these equilibrium points.
更多查看译文
关键词
Learning Methods,Reinforcement Learning Methods,Equilibrium Point,Learning Control,Unstable Point,Actor-critic Algorithm,Degree Of Freedom System,Nonlinear Control Method,Neural Network,Evaluation Of Function,Optimal Control,Linear System,Equations Of Motion,Control Problem,Critical Parameters,Temporal Differences,Single Element,Actor Network,Reward Function,Critic Network,Temporal Difference Learning,Inverted Pendulum,Linear Quadratic Regulator,Pendulum System,Double Deep Q-network,Deep Q-network,Adaptive Elements,Continuous-time Control,Transfer Function,Loss Function
AI 理解论文
溯源树
样例
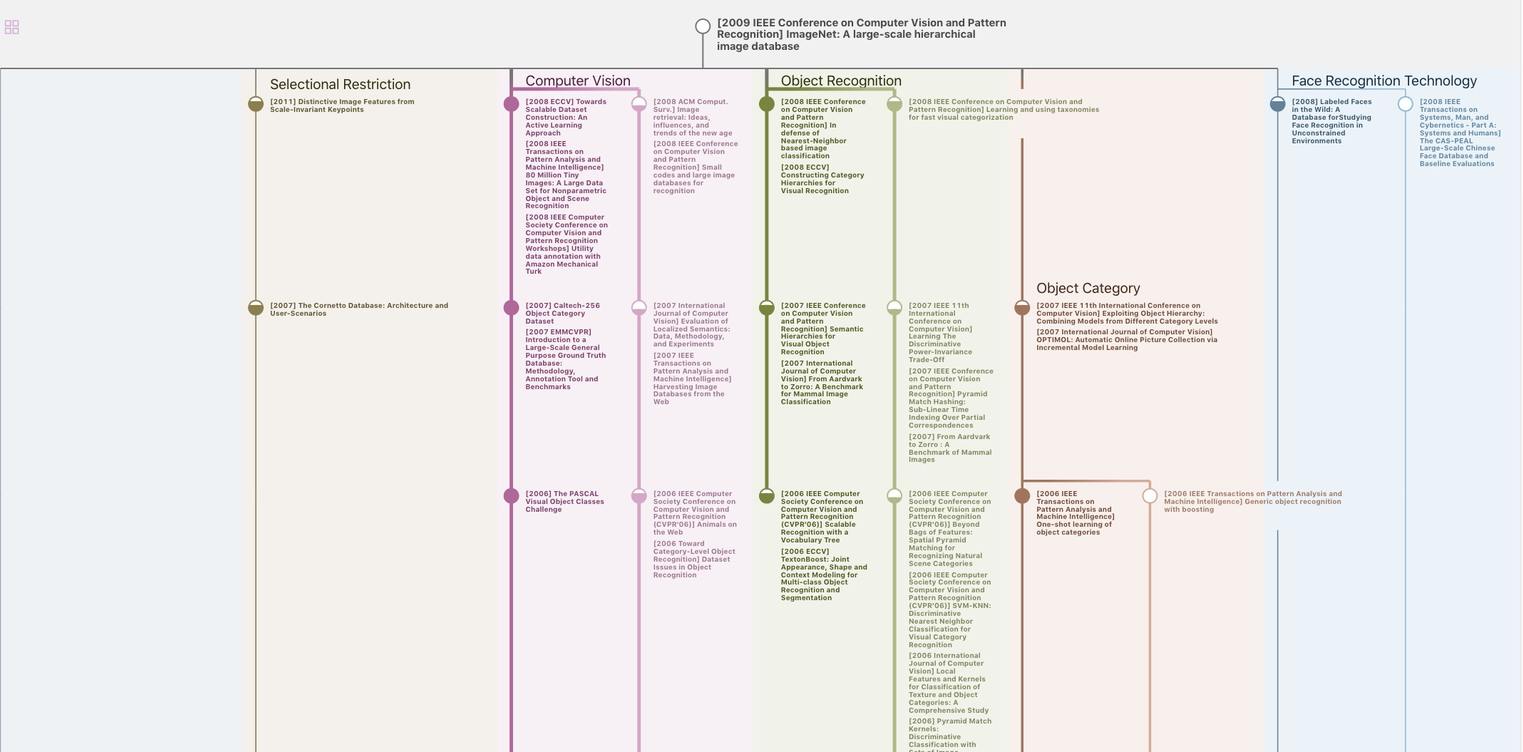
生成溯源树,研究论文发展脉络
Chat Paper
正在生成论文摘要