On the Selection of Item Scores or Composite Scores for Clinical Prediction
MULTIVARIATE BEHAVIORAL RESEARCH(2023)
摘要
Recent shifts to prioritize prediction, rather than explanation, in psychological science have increased applications of predictive modeling methods. However, composite predictors, such as sum scores, are still commonly used in practice. The motivations behind composite test scores are largely intertwined with reducing the influence of measurement error in answering explanatory questions. But this may be detrimental for predictive aims. The present paper examines the impact of utilizing composite or item-level predictors in linear regression. A mathematical examination of the bias-variance decomposition of prediction error in the presence of measurement error is provided. It is shown that prediction bias, which may be exacerbated by composite scoring, drives prediction error for linear regression. This may be particularly salient when composite scores are comprised of heterogeneous items such as in clinical scales where items correspond to symptoms. With sufficiently large training samples, the increased prediction variance associated with item scores becomes negligible even when composite scores are sufficient. Practical implications of predictor scoring are examined in an empirical example predicting suicidal ideation from various depression scales. Results show that item scores can markedly improve prediction particularly for symptom-based scales. Cross-validation methods can be used to empirically justify predictor scoring decisions.
更多查看译文
关键词
Measure scoring,prediction,regression,reliability,suicide
AI 理解论文
溯源树
样例
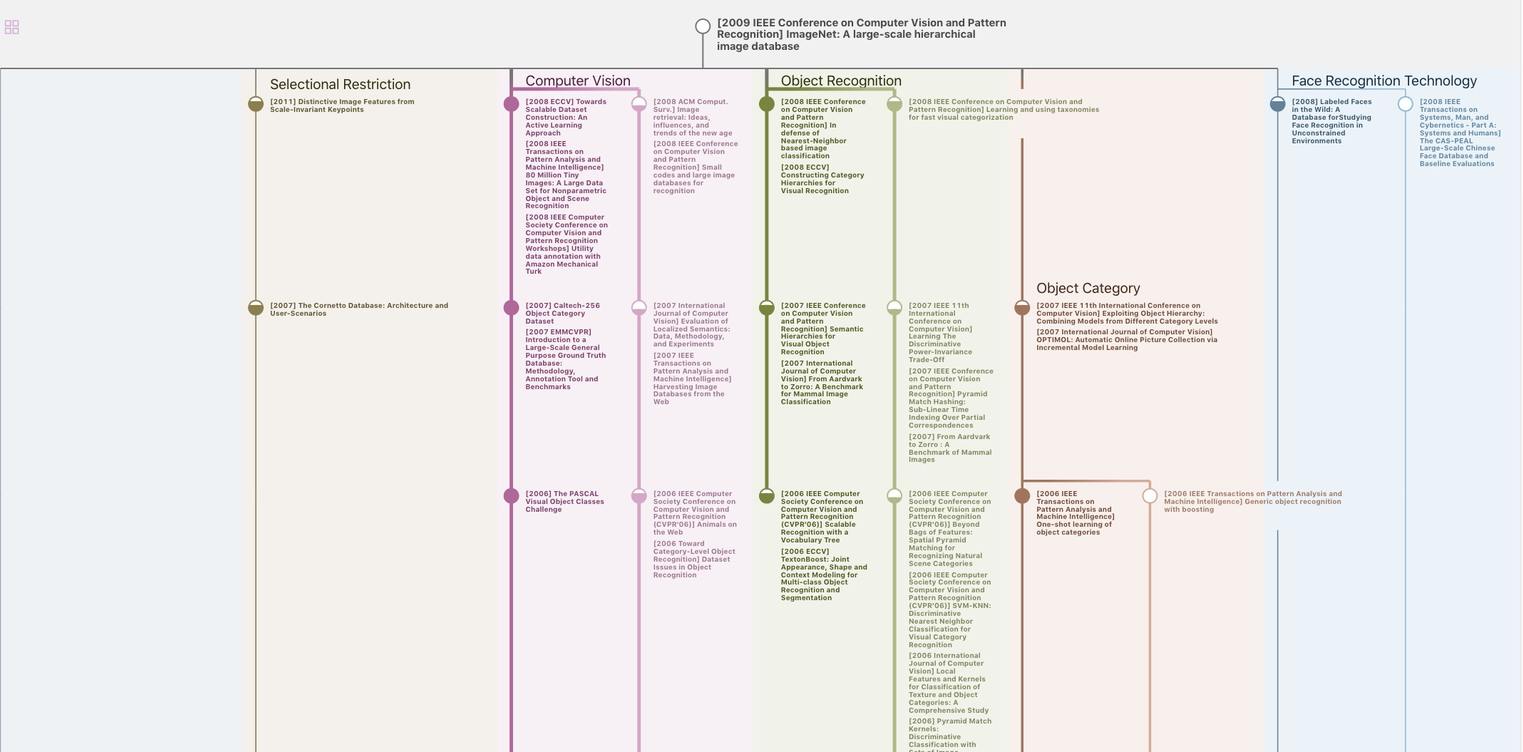
生成溯源树,研究论文发展脉络
Chat Paper
正在生成论文摘要