Radiomics derived from T2-FLAIR: the value of 2- and 3-classification tasks for different lesions in multiple sclerosis
QUANTITATIVE IMAGING IN MEDICINE AND SURGERY(2024)
摘要
Background: White matter (WM) lesions can be classified into contrast enhancement lesions (CELs), iron rim lesions (IRLs), and non -iron rim lesions (NIRLs) based on different pathological mechanism in relapsing -remitting multiple sclerosis (RRMS). The application of radiomics established by T2 -FLAIR to classify WM lesions in RRMS is limited, especially for 3 -class classification among CELs, IRLs, and NIRLs. Methods: A total of 875 WM lesions (92 CELs, 367 IRLs, 416 NIRLs) were included in this study. The 2 -class classification was only performed between IRLs and NIRLs. For the 2- and 3 -class classification tasks, all the lesions were randomly divided into training and testing sets with a ratio of 8:2. We used least absolute shrinkage and selection operator (LASSO), reliefF algorithm, and mutual information (MI) for feature selection, then eXtreme gradient boosting (XGBoost), random forest (RF), and support vector machine (SVM) were used to establish discrimination models. Finally, the area under the curve (AUC), accuracy, sensitivity, specificity, and precision were used to evaluate the performance of the models. Results: For the 2 -class classification model, LASSO classifier with RF model showed the best discrimination performance with the AUC of 0.893 (95% CI: 0.838-0.942), accuracy of 0.813, sensitivity of 0.833, specificity of 0.781, and precision of 0.851. However, the 3 -class classification model of LASSO with XGBoost displayed the highest performance with the AUC of 0.920 (95% CI: 0.887-0.950), accuracy of 0.796, sensitivity of 0.839, specificity of 0.881, and precision of 0.846. Conclusions: Radiomics models based on T2 -FLAIR images have the potential for discriminating among CELs, IRLs, and NIRLs in RRMS.
更多查看译文
关键词
Magnetic resonance imaging (MRI),multiple sclerosis (MS),radiomics
AI 理解论文
溯源树
样例
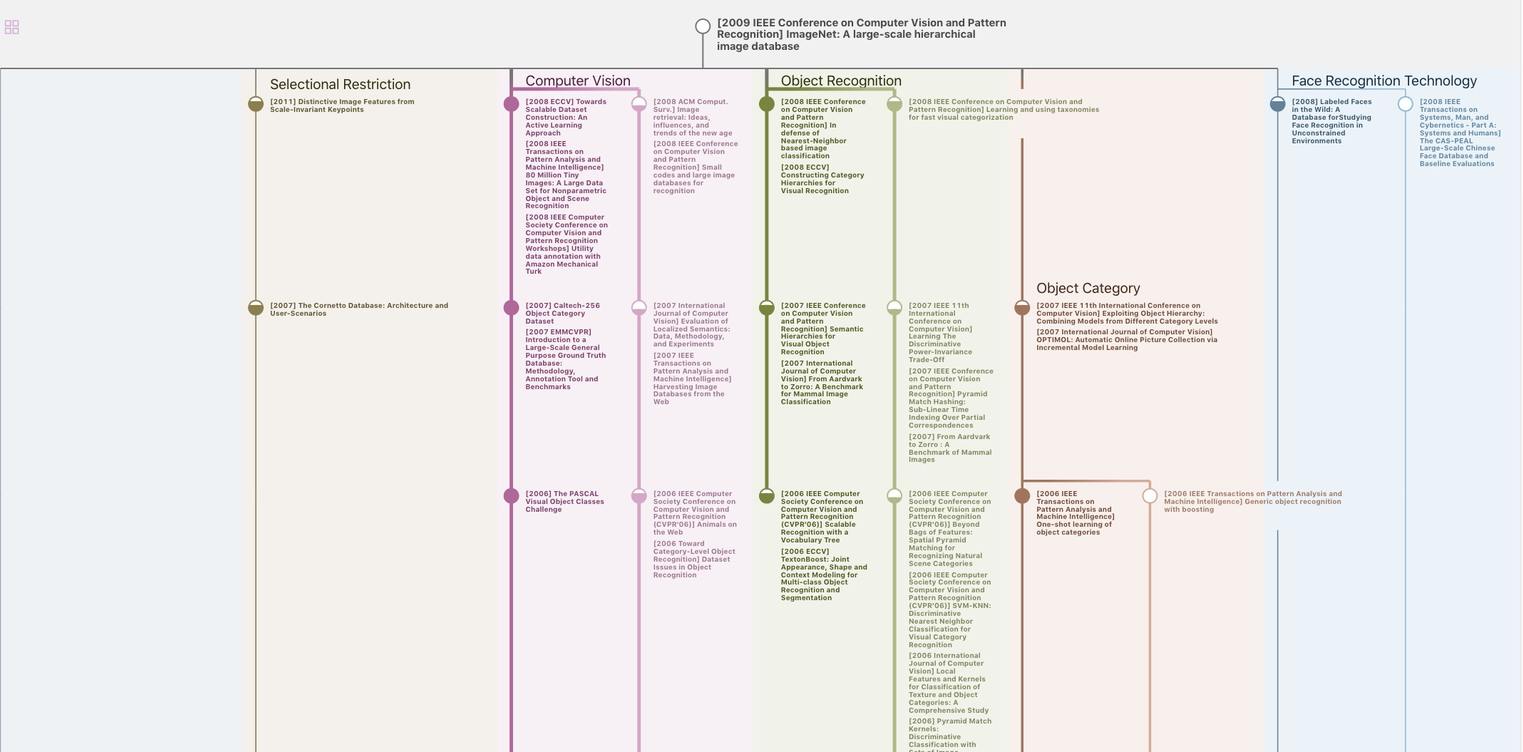
生成溯源树,研究论文发展脉络
Chat Paper
正在生成论文摘要