Imagine, Initialize, and Explore: an Effective Exploration Method in Multi-Agent Reinforcement Learning
Proceedings of the AAAI Conference on Artificial Intelligence(2024)
摘要
Effective exploration is crucial to discovering optimal strategies formulti-agent reinforcement learning (MARL) in complex coordination tasks.Existing methods mainly utilize intrinsic rewards to enable committedexploration or use role-based learning for decomposing joint action spacesinstead of directly conducting a collective search in the entireaction-observation space. However, they often face challenges obtainingspecific joint action sequences to reach successful states in long-horizontasks. To address this limitation, we propose Imagine, Initialize, and Explore(IIE), a novel method that offers a promising solution for efficientmulti-agent exploration in complex scenarios. IIE employs a transformer modelto imagine how the agents reach a critical state that can influence eachother's transition functions. Then, we initialize the environment at this stateusing a simulator before the exploration phase. We formulate the imagination asa sequence modeling problem, where the states, observations, prompts, actions,and rewards are predicted autoregressively. The prompt consists oftimestep-to-go, return-to-go, influence value, and one-shot demonstration,specifying the desired state and trajectory as well as guiding the actiongeneration. By initializing agents at the critical states, IIE significantlyincreases the likelihood of discovering potentially important under-exploredregions. Despite its simplicity, empirical results demonstrate that our methodoutperforms multi-agent exploration baselines on the StarCraft Multi-AgentChallenge (SMAC) and SMACv2 environments. Particularly, IIE shows improvedperformance in the sparse-reward SMAC tasks and produces more effectivecurricula over the initialized states than other generative methods, such asCVAE-GAN and diffusion models.
更多查看译文
关键词
Reinforcement Learning,Multi-Agent Systems,Curiosity-Driven Exploration
AI 理解论文
溯源树
样例
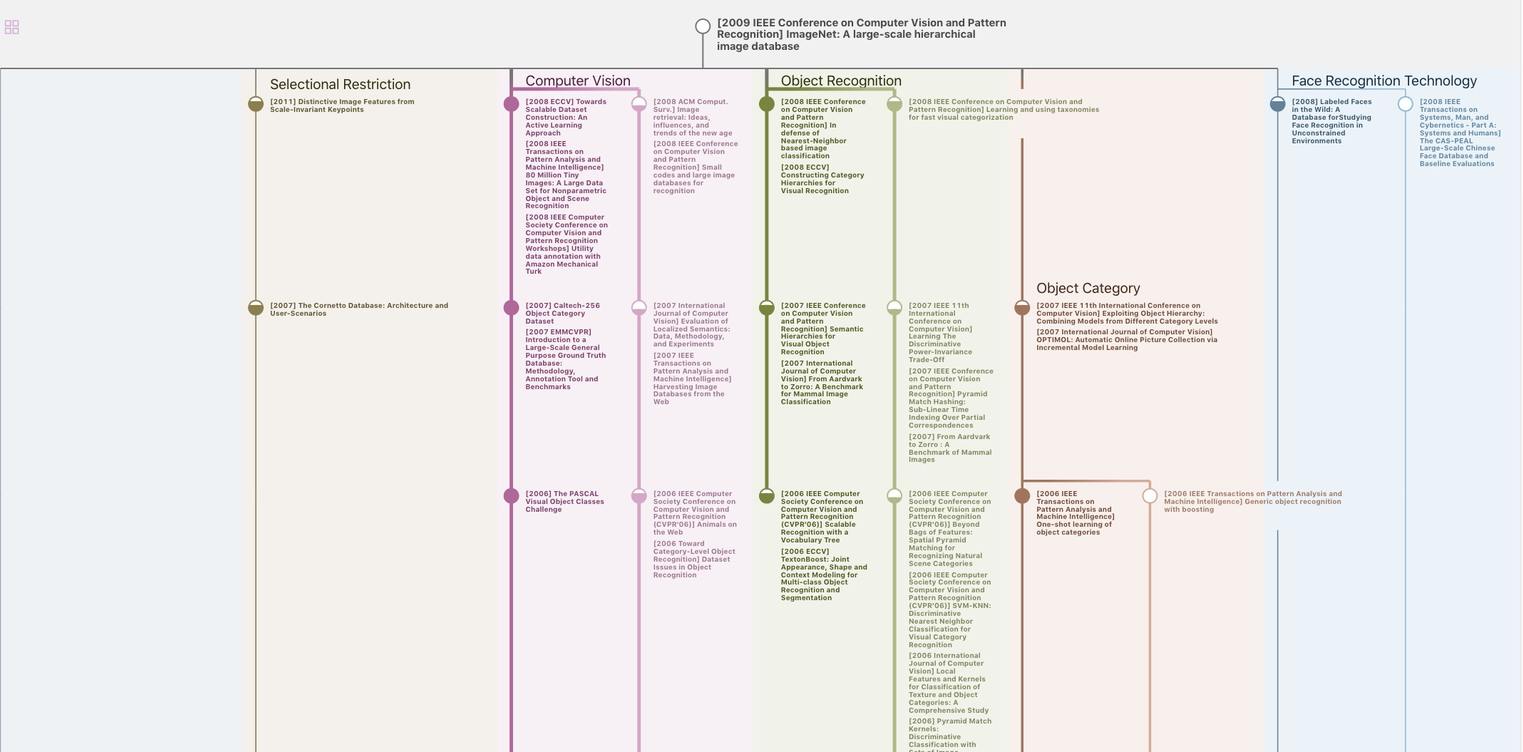
生成溯源树,研究论文发展脉络
Chat Paper
正在生成论文摘要