LLM Task Interference: an Initial Study on the Impact of Task-Switch in Conversational History
EMNLP 2024(2024)
关键词
Spoken Dialogue Systems,Multimodal Interaction,Reinforcement Learning,Dialog Management,Semantic Processing
AI 理解论文
溯源树
样例
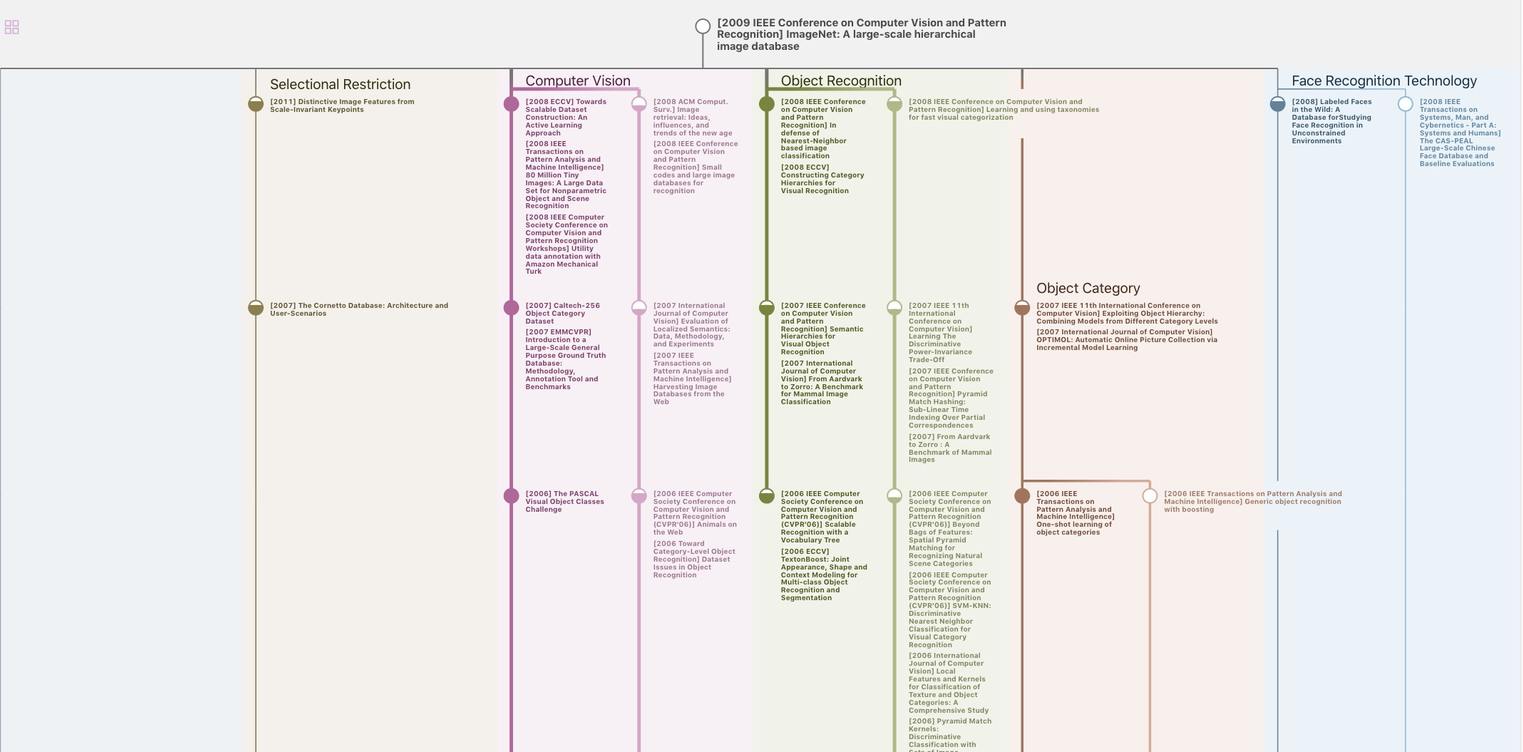
生成溯源树,研究论文发展脉络
Chat Paper
正在生成论文摘要