Computation-information gap in high-dimensional clustering
arxiv(2024)
摘要
We investigate the existence of a fundamental computation-information gap for
the problem of clustering a mixture of isotropic Gaussian in the
high-dimensional regime, where the ambient dimension p is larger than the
number n of points. The existence of a computation-information gap in a
specific Bayesian high-dimensional asymptotic regime has been conjectured by
arXiv:1610.02918 based on the replica heuristic from statistical physics. We
provide evidence of the existence of such a gap generically in the
high-dimensional regime p ≥ n, by (i) proving a non-asymptotic low-degree
polynomials computational barrier for clustering in high-dimension, matching
the performance of the best known polynomial time algorithms, and by (ii)
establishing that the information barrier for clustering is smaller than the
computational barrier, when the number K of clusters is large enough. These
results are in contrast with the (moderately) low-dimensional regime n ≥
poly(p, K), where there is no computation-information gap for clustering a
mixture of isotropic Gaussian. In order to prove our low-degree computational
barrier, we develop sophisticated combinatorial arguments to upper-bound the
mixed moments of the signal under a Bernoulli Bayesian model.
更多查看译文
AI 理解论文
溯源树
样例
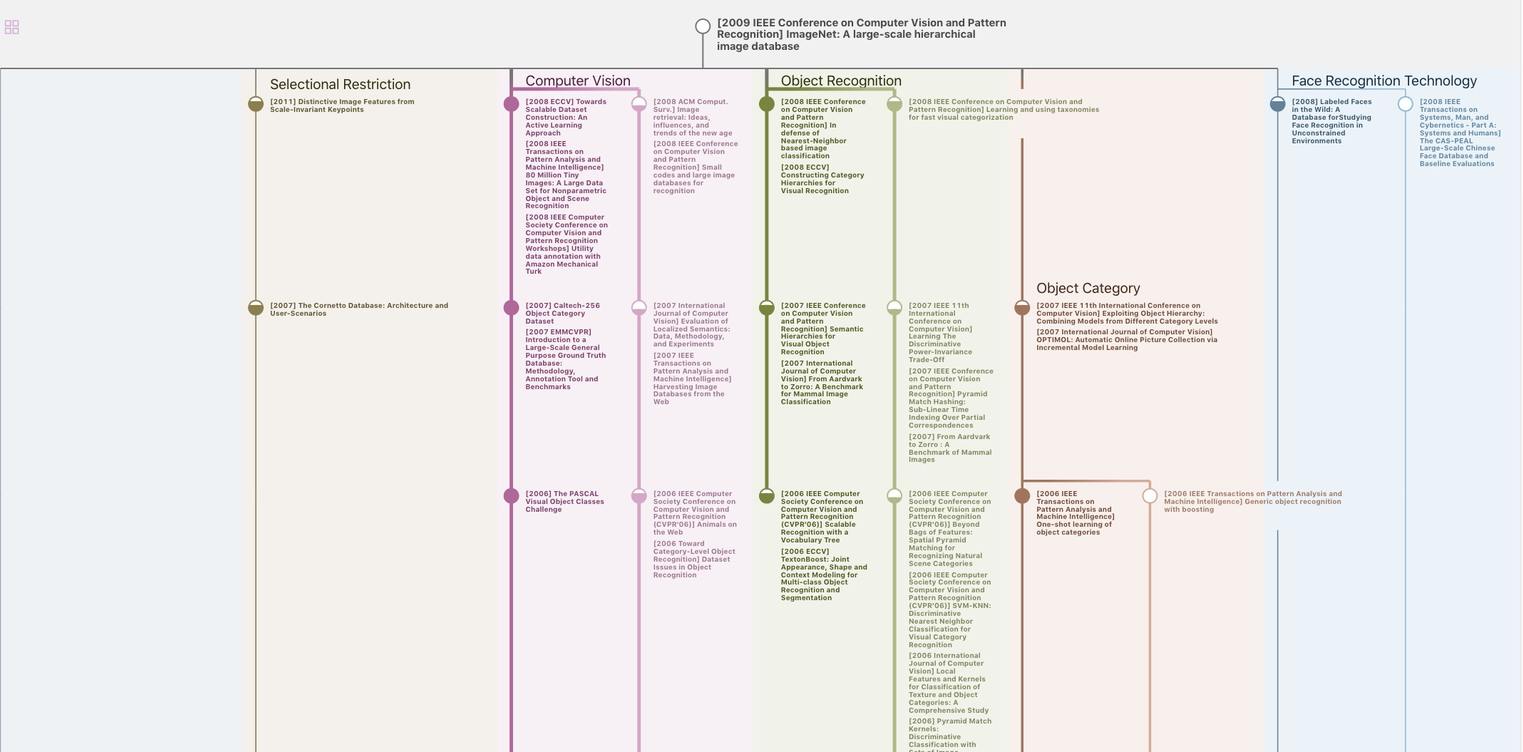
生成溯源树,研究论文发展脉络
Chat Paper
正在生成论文摘要