A Non-Intrusive Machine Learning Framework for Debiasing Long-Time Coarse Resolution Climate Simulations and Quantifying Rare Events Statistics
JOURNAL OF ADVANCES IN MODELING EARTH SYSTEMS(2024)
摘要
Due to the rapidly changing climate, the frequency and severity of extreme weather is expected to increase over the coming decades. As fully-resolved climate simulations remain computationally intractable, policy makers must rely on coarse-models to quantify risk for extremes. However, coarse models suffer from inherent bias due to the ignored "sub-grid" scales. We propose a framework to non-intrusively debias coarse-resolution climate predictions using neural-network (NN) correction operators. Previous efforts have attempted to train such operators using loss functions that match statistics. However, this approach falls short with events that have longer return period than that of the training data, since the reference statistics have not converged. Here, the scope is to formulate a learning method that allows for correction of dynamics and quantification of extreme events with longer return period than the training data. The key obstacle is the chaotic nature of the underlying dynamics. To overcome this challenge, we introduce a dynamical systems approach where the correction operator is trained using reference data and a coarse model simulation nudged toward that reference. The method is demonstrated on debiasing an under-resolved quasi-geostrophic model and the Energy Exascale Earth System Model (E3SM). For the former, our method enables the quantification of events that have return period two orders longer than the training data. For the latter, when trained on 8 years of ERA5 data, our approach is able to correct the coarse E3SM output to closely reflect the 36-year ERA5 statistics for all prognostic variables and significantly reduce their spatial biases. We present a general framework to design machine learned correction operators to improve the predicted statistics of low-resolution climate simulations. We illustrate the approach, which acts on existing data in a post-processing manner, on a simplified prototype climate model as well as a realistic climate model, namely the Energy Exascale Earth System Model (E3SM) with 110 km resolution. For the latter, we show that the developed approach is able to correct the low-resolution E3SM output to closely reflect the climate statistics of historical observations as quantified by the ERA5 data set. We also demonstrate that our model significantly improves the prediction of atmospheric rivers, an example of extreme weather events resolvable by the low resolution model. Development of non-intrusive correction operators for coarse scale climate simulations Design of a training procedure that improves dynamics and allows for characterization of extremes with return period longer than the training data Application to Energy Exascale Earth System Model and demonstration of improvement on global and regional statistics
更多查看译文
关键词
climate modeling,extreme event statistics,debiasing,nudging,machine learning
AI 理解论文
溯源树
样例
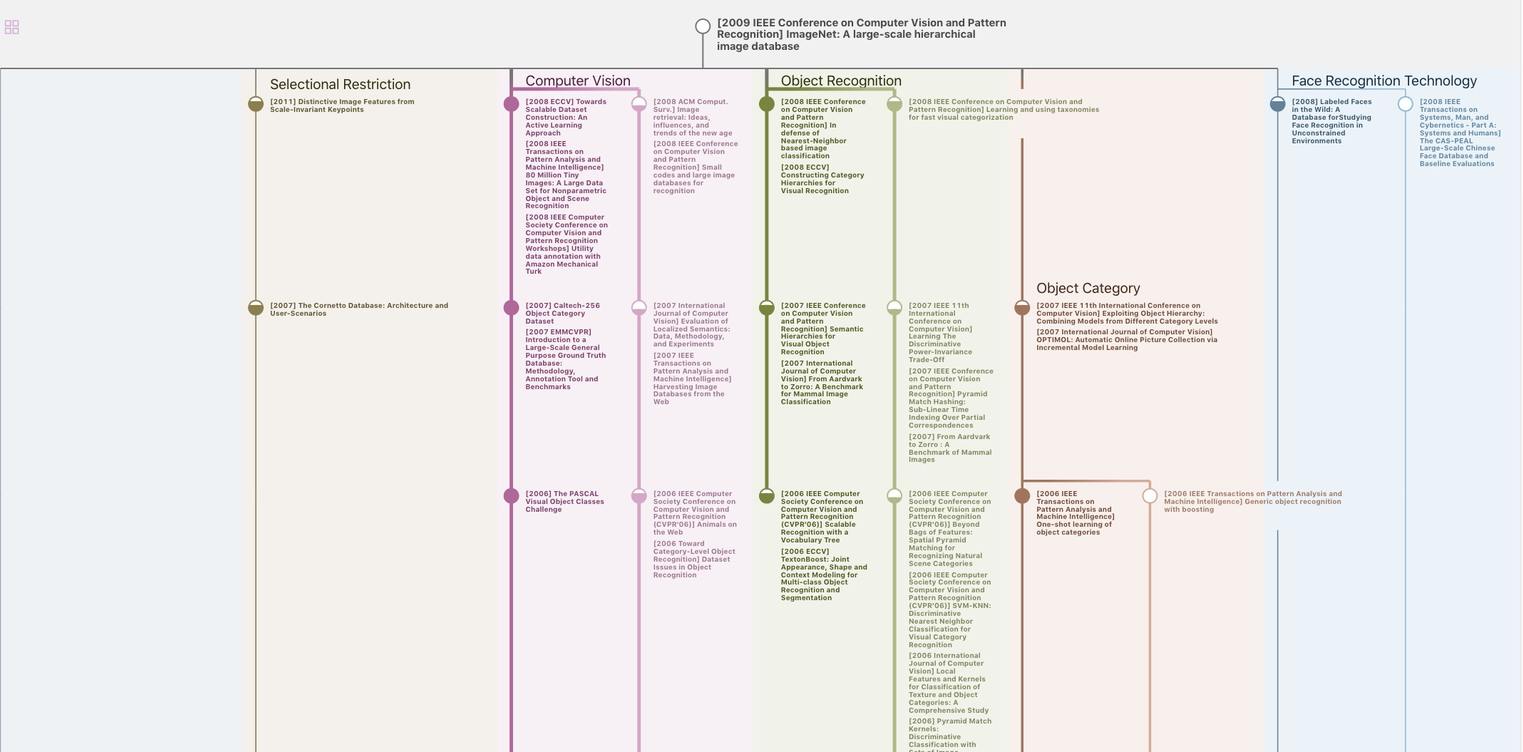
生成溯源树,研究论文发展脉络
Chat Paper
正在生成论文摘要