PocketGen: Generating Full-Atom Ligand-Binding Protein Pockets.
bioRxiv : the preprint server for biology(2024)
摘要
Designing small-molecule-binding proteins, such as enzymes and biosensors, is crucial in protein biology and bioengineering. Generating high-fidelity protein pockets-areas where proteins interact with ligand molecules-is challenging due to complex interactions between ligand molecules and proteins, flexibility of ligand molecules and amino acid side chains, and complex sequence-structure dependencies. Here, we introduce PocketGen, a deep generative method for generating the residue sequence and the full-atom structure within the protein pocket region that leverages sequence-structure consistency. PocketGen consists of a bilevel graph transformer for structural encoding and a sequence refinement module that uses a protein language model (pLM) for sequence prediction. The bilevel graph transformer captures interactions at multiple granularities (atom-level and residue/ligand-level) and aspects (intra-protein and protein-ligand) with bilevel attention mechanisms. For sequence refinement, a structural adapter using cross-attention is integrated into a pLM to ensure structure-sequence consistency. During training, only the adapter is fine-tuned, while the other layers of the pLM remain unchanged. Experiments show that PocketGen can efficiently generate protein pockets with higher binding affinity and validity than state-of-the-art methods. PocketGen is ten times faster than physics-based methods and achieves a 95% success rate (percentage of generated pockets with higher binding affinity than reference pockets) with over 64% amino acid recovery rate.
更多查看译文
AI 理解论文
溯源树
样例
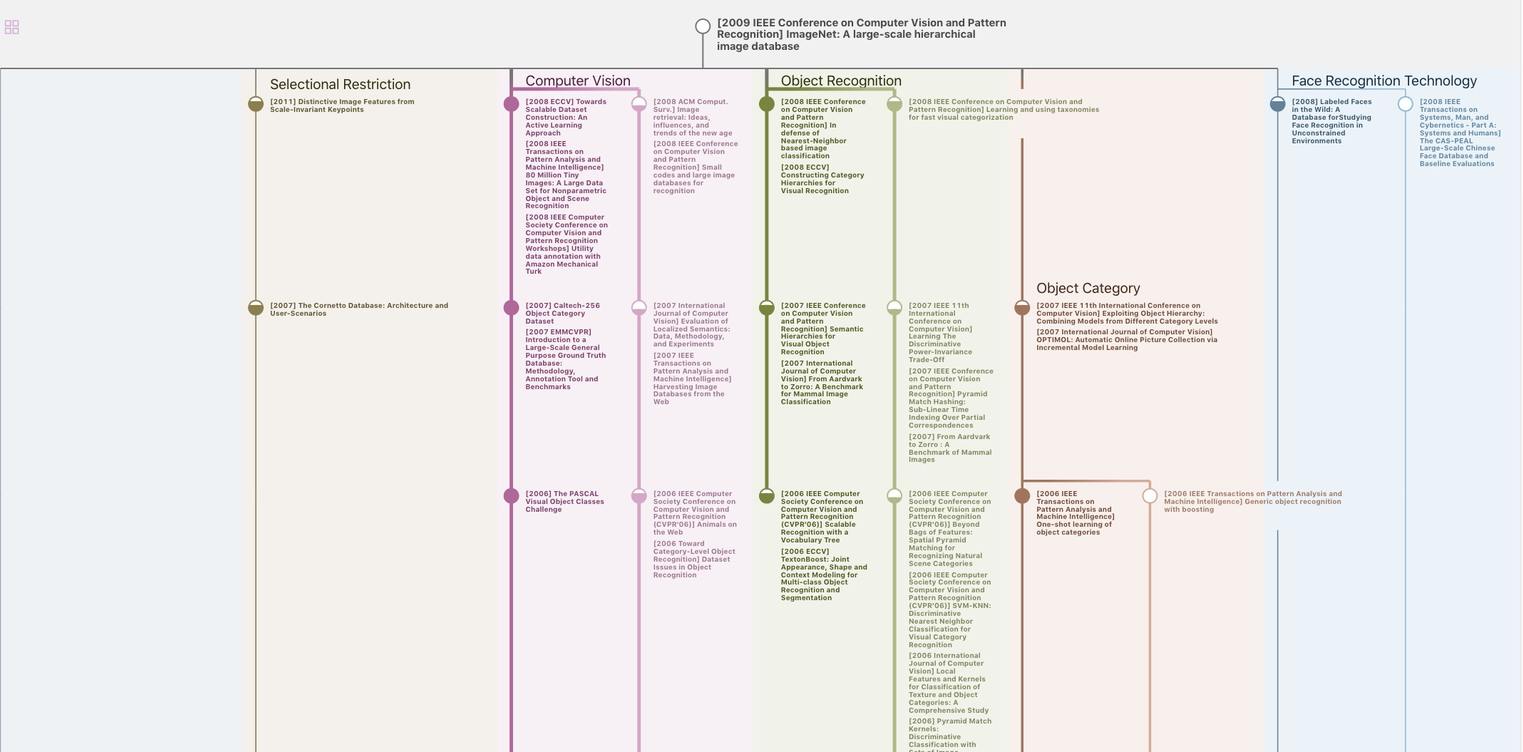
生成溯源树,研究论文发展脉络
Chat Paper
正在生成论文摘要