Multiscale Attention-Based Subdomain Dynamic Adaptation for Cross-Domain Scene Classification
IEEE geoscience and remote sensing letters(2024)
摘要
Cross-domain scene classification is proposed to address the problems of difficult labeling of remote-sensing (RS) image datasets and poor generalization ability of supervised models, aiming to better utilize existing knowledge. To minimize the difference between the source- and target-domain distributions, many deep-domain adaptation methods have been generated, but most of them are based on the difference metric function, which only globally aligns the edge distributions without taking into account the effect of each sample on the network in different and the relationship between related subdomains in different domains of the same category, resulting in a large amount of fine-grained information being lost. In addition, existing domain adaptation methods do not adaptively balance the weights of the marginal and conditional distributions well. To overcome the above difficulties, a multiscale attention-based subdomain dynamic adaptation (SAMRA) method is proposed. The relative importance of the two is balanced by calculating the dynamic weights of each sample in the different domains to globally adjust the marginal distribution and by obtaining finer-grained key information from the subdomains to locally adjust the conditional distribution. Moreover, multiscale feature generation and attention mechanisms support the extraction of more robust features and more complete information, as well as more purposeful transfer. The results of cross-domain experiments show that the improvement of SAMRA over state-of-the-art deep-domain adaptation methods is significant.
更多查看译文
关键词
Attention mechanism,cross-scene classification,deep subdomain adaptation,multiscale feature generation
AI 理解论文
溯源树
样例
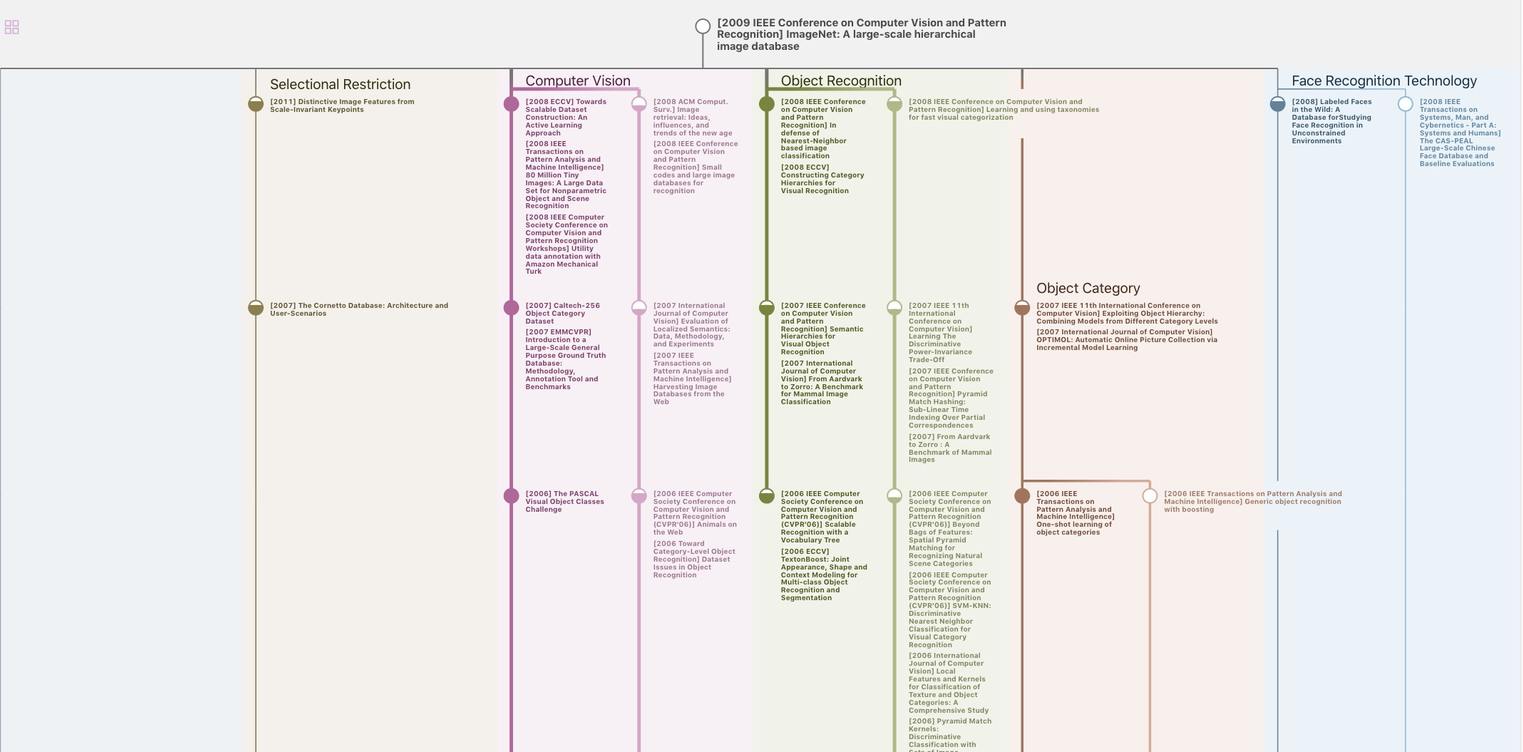
生成溯源树,研究论文发展脉络
Chat Paper
正在生成论文摘要