CMSE: Cross-Modal Semantic Enhancement Network for Classification of Hyperspectral and LiDAR Data
IEEE TRANSACTIONS ON GEOSCIENCE AND REMOTE SENSING(2024)
摘要
The fusion of hyperspectral image (HSI) and light detection and ranging (LiDAR) data is widely used for land cover classification. However, due to different imaging mechanisms, HSI and LiDAR data always present significant image differences, and the dimensions and feature distributions of HSI and LiDAR are highly dissimilar. This makes it challenging to represent and correlate semantic information from multimodal data. Current methods for classifying pixel-by-pixel features, which rely on cascaded or attention-based fusion, cannot effectively use multimodal features. To achieve accurate classification results, extracting and fusing similar high-order semantic information and complementary discriminative information contained in multimodal data is vital. In this article, we propose a cross-modal semantic enhancement network (CMSE) for multimodal semantic information mining and fusion. Our proposed CMSE framework extracts features from the image on multiple scales, capturing more representative local sparse features with different sizes of convolution kernels. To represent high-level semantic features related to land cover, we establish a Gaussian-weighted matrix and semantically transform the spatial and spectral features of distinct branches. Finally, we build a multilevel residual fusion module to incrementally fuse spectral features from HSI and elevation features from LiDAR. Additionally, we introduce a cross-modal semantically constrained loss to guide multimodal semantic feature alignment. We evaluate our approach on three multimodal remote sensing (RS) datasets, namely the Houston2013, Trento, and MUUFL datasets. The experimental results demonstrate that our proposed CMSE model achieves superior performance in terms of accuracy and robustness compared to other related deep networks.
更多查看译文
关键词
Semantics,Laser radar,Feature extraction,Hyperspectral imaging,Land surface,Data models,Data mining,Classification,land cover,multimodal,remote sensing (RS),semantic features
AI 理解论文
溯源树
样例
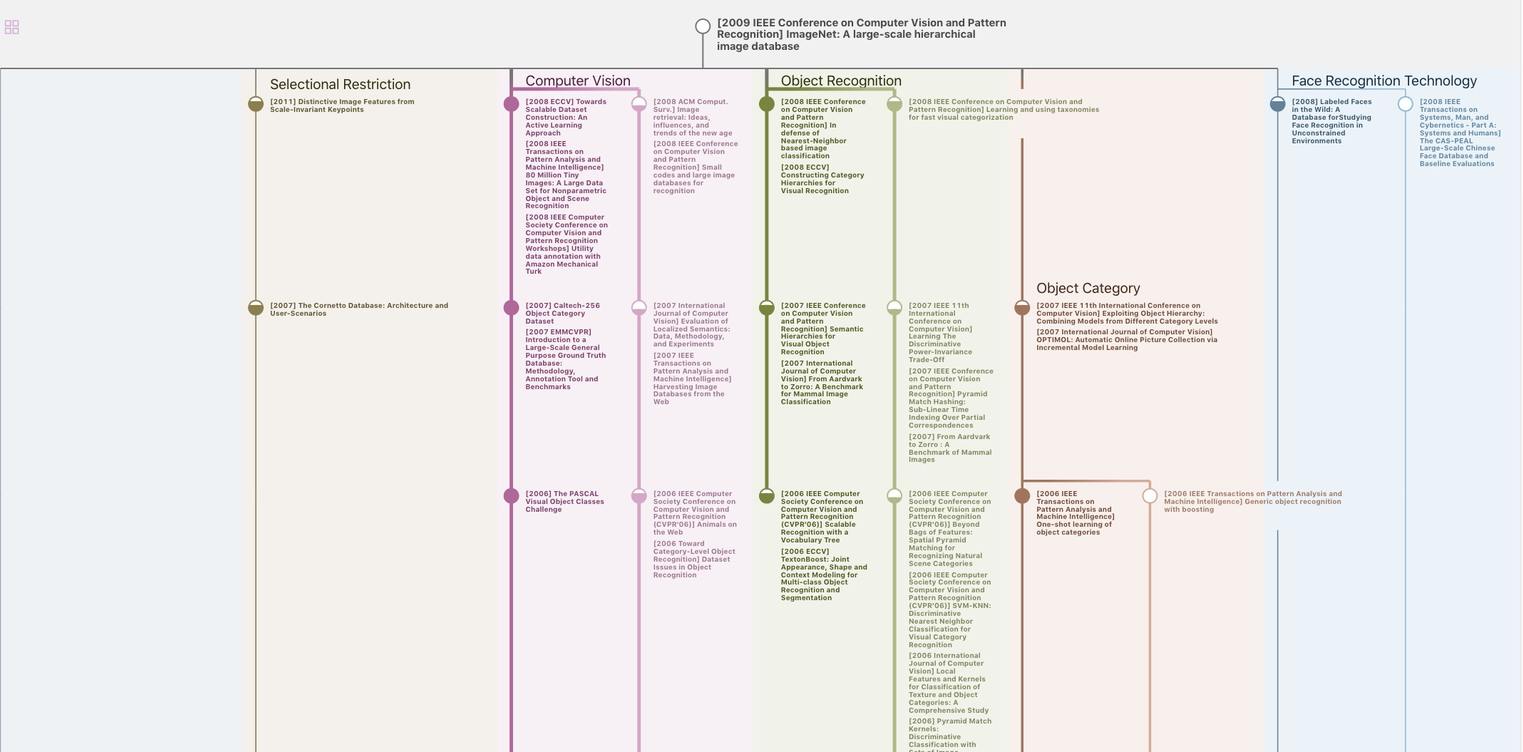
生成溯源树,研究论文发展脉络
Chat Paper
正在生成论文摘要