Multimodal Adversarial Fusion for Typhoon Intensity Forecasting
IEEE TRANSACTIONS ON GEOSCIENCE AND REMOTE SENSING(2024)
摘要
Typhoon poses a significant threat to casualties and economic damage in coastal areas. Accurate forecasting of typhoon intensity (TI) is crucial for effective disaster management and mitigation strategies. Despite making certain progress in integrating this task with deep learning techniques, it still faces limitations due to insufficient consideration of the intramodal characteristics and the intermodal discrepancy. In this article, we propose a novel multimodal adversarial fusion (MAF) approach for TI forecasting, which contains feature encoding and multimodal fusion modules. Within the feature encoding module, we adopt a novel block attention mechanism to efficiently reveal the relationships of observational factors in multiple latent spaces, thereby obtaining more comprehensive representations feature of the observational data. Moreover, we introduce a complex convolutional operation to encode the components of wind speed within the reanalysis data, which can maintain their characteristics through the real and imaginary parts of complex numbers during the encoding procedure. Finally, we design the adversarial fusion scheme to effectively narrow the modality gap and facilitate a more comprehensive fusion for TI forecasting. Comprehensive experiments are conducted on CMA Best-Track (CMA-BST)/ERA-Interim data and extensive results verify the effectiveness of our method for TI forecasting.
更多查看译文
关键词
Adversarial training,attention mechanism,complex convolutional network,typhoon intensity (TI) forecasting
AI 理解论文
溯源树
样例
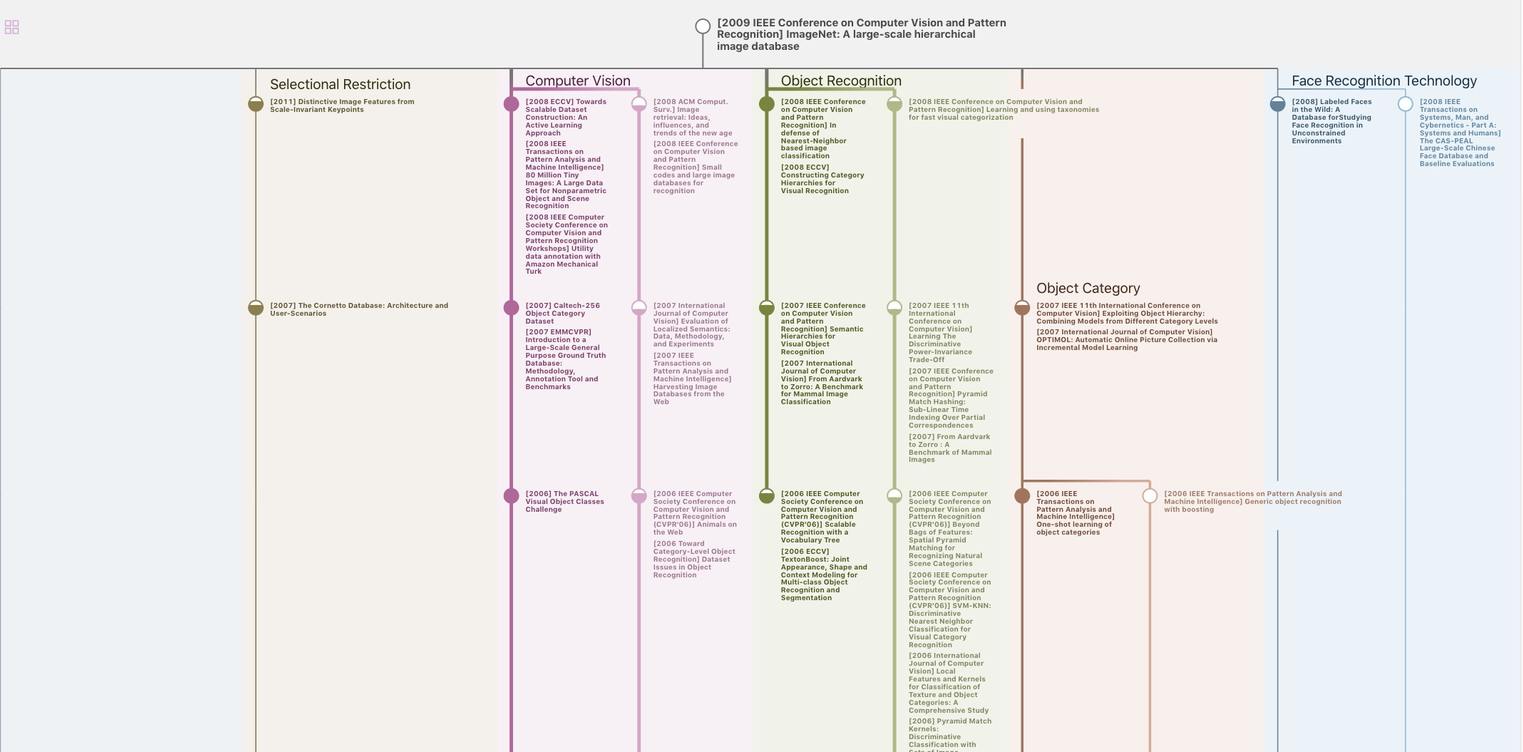
生成溯源树,研究论文发展脉络
Chat Paper
正在生成论文摘要